Learning table tennis with augmented reality
Table tennis has experienced a worldwide surge of popularity as a competitive sport. But finding qualified coaches to teach table tennis can be difficult, costly, and time-consuming. That’s why Purdue University researchers have developed a 3D augmented-reality table tennis instruction system, giving instant feedback to beginning players.
“I became faculty advisor and coach for the Purdue Table Tennis club in 2022,” said Karthik Ramani, the Donald W. Feddersen Distinguished Professor of Mechanical Engineering. “I quickly realized that existing methods of table tennis training were highly inefficient. You could watch YouTube videos or hire a trainer, but there was no way to get precise feedback on how well you were doing. We wanted to create something better than videos or human coaches.”
Ramani’s Convergence Design Lab has extensive experience in using augmented reality (AR) in classroom education, robotics, and even teaching welding to factory workers. So naturally they investigated extending AR into the table tennis world.
“A coach will give personal instructions on how to execute a proper stroke, which the player will try to mimic," said Dizhi Ma, a Ph.D. student in electrical and computer engineering. "But it’s difficult for the player to tell if they are executing the stroke correctly. And then what if the coach isn’t there? How do you make sure you’re practicing the right way?”
That’s why Ma and a team of engineers developed avaTTAR, a software and hardware AR platform that records a coach’s movements in 3D, and offers it as instant feedback for beginning players who can see instantly whether they are executing the strokes correctly. Ma presented this research at the 37th Annual ACM Symposium on User Interface Software and Technology (UIST) in Pittsburgh, PA in October 2024.
Step one for the team was conducting surveys of exiting players to determine the pain points of learning table tennis. They determined that a robust system needed to accurately reconstruct a player’s motion; compare it with an instructor’s; offer instant visual feedback; and have an easy-to-use visual interface.
“We use an off-the-shelf webcam to record the full body posture,” Ma said. “We also attach a sensor to the racket, so we can track its orientation. With a machine-learning algorithm, that enables us to construct a 3D skeletal avatar that you can see in real-time.”
In fact, their system constructs two avatars: one for the instructor, and one for the player. The instructor’s avatar is pre-recorded, showcasing the proper stance for all the necessary table tennis strokes. Then the player dons a pair of AR goggles, showing their own stance in comparison with the prerecorded instructor. Any variances instantly light up a different color on their avatar, showing the player in real-time how they should alter their stance.
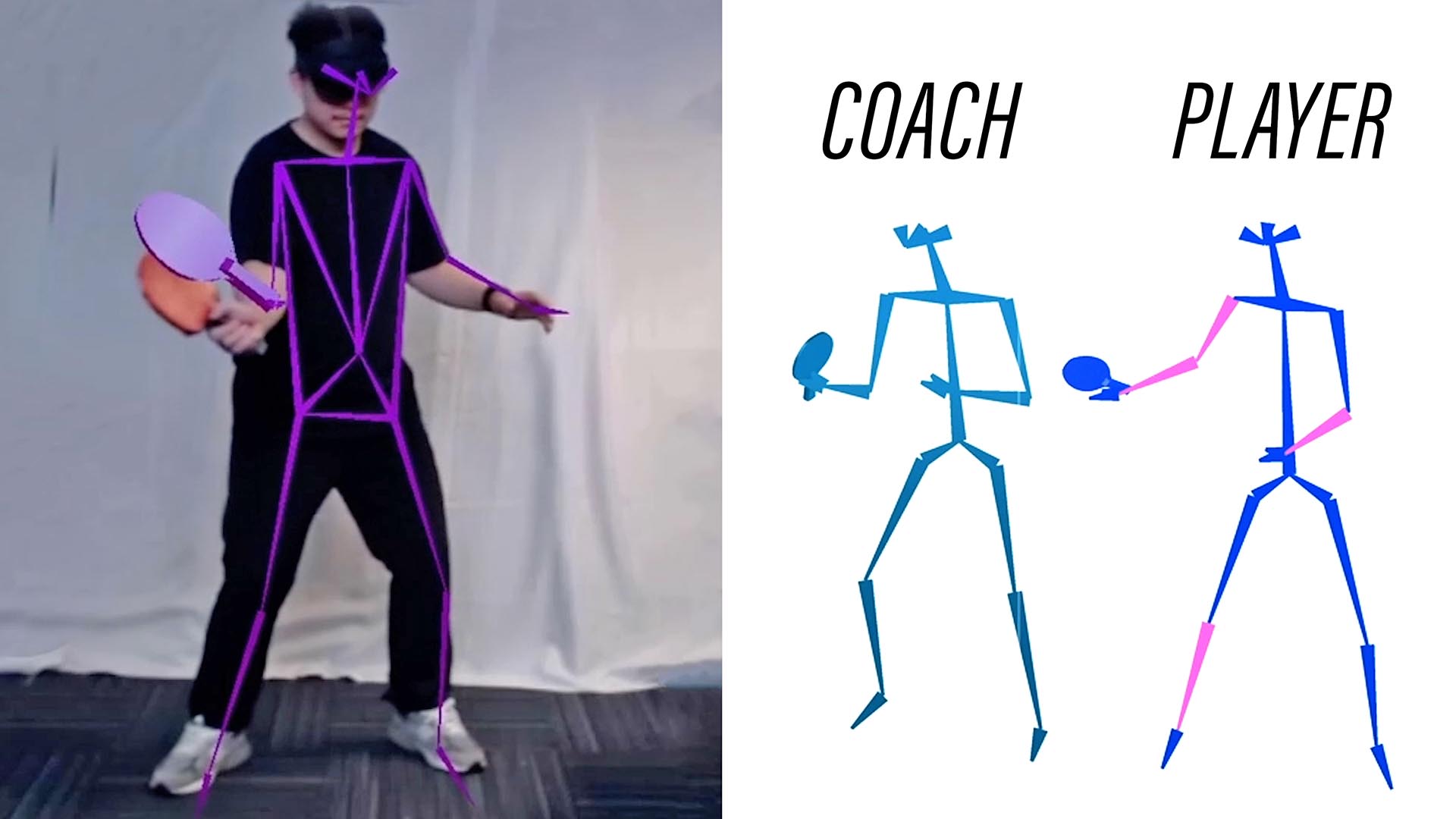
“It’s kind of like a mirror,” Ma said. “But this is much better. You have control of the playback, so you can replay the stroke as many times as you want, and slow down the playback to match its movements. And because it’s 3D, you can actually walk around the avatar and see it from many different angles to help you understand.”
“This is real immersion,” Ramani said. “It’s like having the instructor there with you in the room, except this is more flexible, more scalable, and offers instant feedback customized to your own movements.”
The team also ran a user study to test its efficacy. Players’ posture accuracy increased instantly by 10% with the avaTTAR system, versus using traditional 2D video training methods.
This combination of machine learning and human-computer interaction aligns with Purdue Computes, a university-wide initiative to investigate physical implementations of artificial intelligence and related technologies.
“Sports is a great testing ground for this type of technology,” Ramani said. “Not only could this be expanded to all kinds of racket sports, but it opens doors to all avenues of learning: mechanical tasks, job training, even intricate tasks like surgery can be improved using this system. The cognitive, perception and motor skills of the human all are engaged in both training and learning. It sharpens your decision-making skills, so mind and body are totally connected.”
Source: Karthik Ramani, ramani@purdue.edu; Dizhi Ma, ma742@purdue.edu
Purdue Table Tennis Club: https://www.instagram.com/purduetabletennis/
Writer: Jared Pike, jaredpike@purdue.edu, 765-496-0374
avaTTAR: Table Tennis Stroke Training with On-body and Detached Visualization in Augmented Reality
Dizhi Ma, Xiyun Hu, Jingyu Shi, Mayank Patel, Rahul Jain, Ziyi Liu, Zhengzhe Zhu, Karthik Ramani
https://doi.org/10.48550/arXiv.2407.15373
ABSTRACT: Table tennis stroke training is a critical aspect of player development. We designed a new augmented reality (AR) system, avaTTAR, for table tennis stroke training. The system provides both "on-body" (first-person view) and "detached" (third-person view) visual cues, enabling users to visualize target strokes and correct their attempts effectively with this dual perspectives setup. By employing a combination of pose estimation algorithms and IMU sensors, avaTTAR captures and reconstructs the 3D body pose and paddle orientation of users during practice, allowing real-time comparison with expert strokes. Through a user study, we affirm avaTTAR's capacity to amplify player experience and training results.