Unlocking the secrets of thermal grease using machine learning
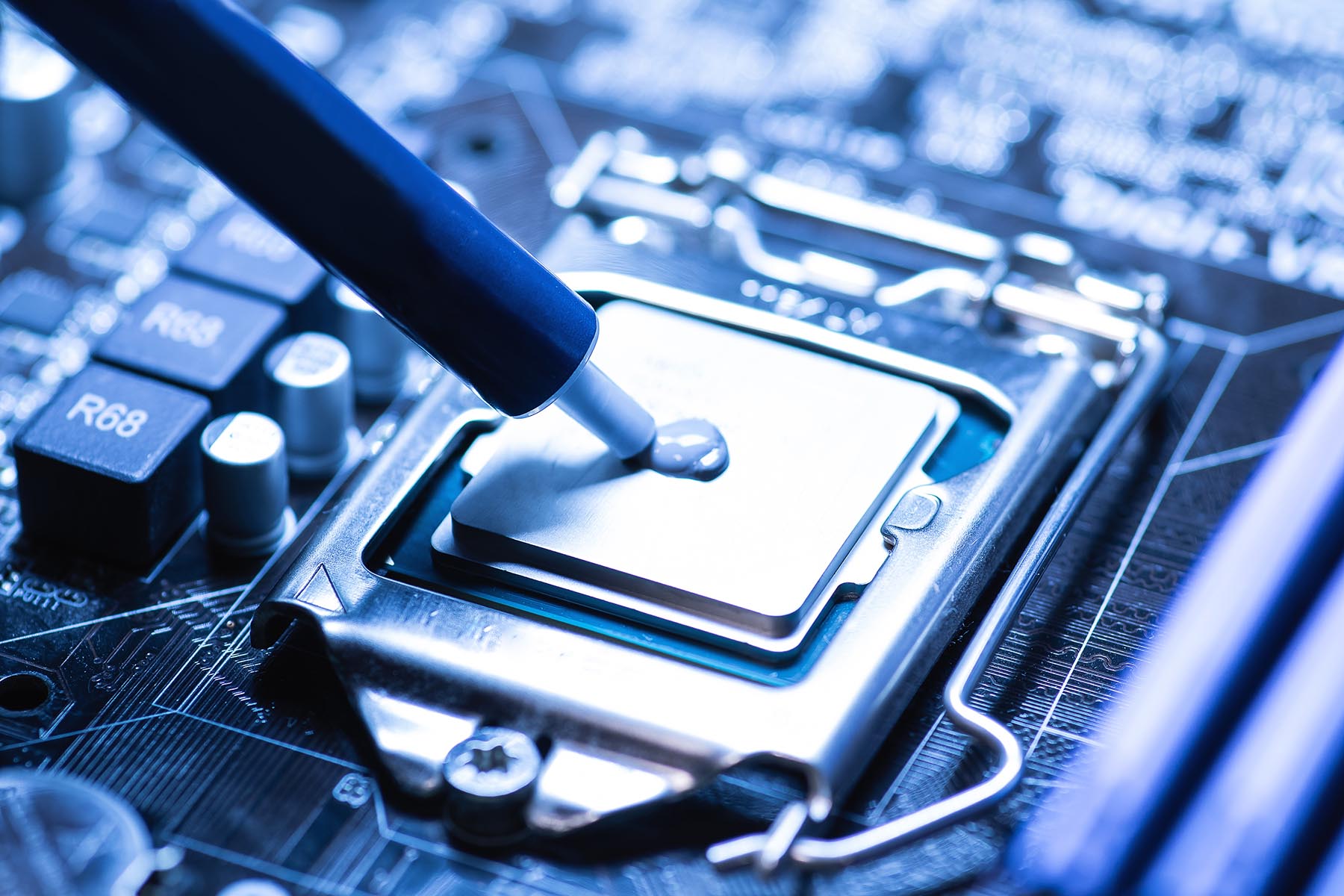
Semiconductors generate a lot of heat, which shortens their life and decreases performance. To remove that heat, a metal heat sink is often attached to conduct heat out to the environment. Between the two, a layer of thermal grease is often applied to bridge the gap and improve heat transfer. This paste-like material incorporates a polymer with embedded metal or ceramic particles to ensure that the heat is transferred efficiently.
“This is becoming more of an issue with today’s semiconductors,” said Amy Marconnet, associate professor of mechanical engineering, whose research focuses on heat transfer. “As they add more and more transistors onto a single chip, it generates more and more heat, requiring more effective materials to dissipate the heat.”
But thermal grease isn’t perfect. Its complex fluidic nature (called its rheological behavior) sometimes causes it to expand and contract as the heat rises and falls. These cyclical stresses may cause the grease to become more fluid and start flowing in unexpected ways. They may also physically degrade the grease over time, leaving voids where heat can escape and cause issues for sensitive electronic components.
Fix the rheology, and you may fix many of the heat issues.
To help Purdue’s Cooling Technologies Research Center tackle this thermal grease issue, Amy brought in Ivan Christov, associate professor of mechanical engineering. “Ivan does some cool work on complex fluid modeling and two-phase flow, so he was perfect for this project,” Amy said.
“My job is to establish what kind of materials these are, how they behave, and how they flow,” Christov said. “Then we can understand what changes to make to stop it from flowing when we don’t want it to.”
Their research has been published in the Journal of Rheology and selected as a featured article.
“When we started investigating these thermal greases, the only information we had was their material data sheets,” said Pranay Nagrani, a Ph.D. student co-advised by Christov and Marconnet who conducted most of the experiments. “But when it comes to rheology of complex fluids — studying how they flow — it’s not as simple as just plugging in a constant number. We had to do some experiments to create a rheological profile of these greases.”
Nagrani collaborated with the team of Kendra Erk, associate professor of materials engineering, to conduct experiments in her lab using a rheometer. This machine uses a concentric cylinder to push against the grease, measuring the forces involved to characterize its response to shear stress.
Once they had experimental results, Nagrani began to build quantitative models using machine learning. Normally, machine learning requires huge datasets to infer patterns or trends, rather than just the results of one experiment. So they used a technique called physics-informed neural networks (PINNs), which incorporate guesses for the rheological laws of the fluid to enhance the dataset, enabling quantitative models to be built from a single type of experiment.
“We analyzed two different greases,” Christov said. “In one of them, the stress built up over time. In the other, stress relaxed over time, which would cause it to potentially become more fluid and create voids. Nobody has ever measured this before in thermal greases.”
“I’m used to physical experiments where we study the degradation of materials over time, and that can take weeks or longer,” Marconnet said. “Having a rheological model of the properties of these greases gives us a much more efficient tool of understanding their thermal performance.”
Marconnet says that the results were well received. “All of the industry partners we work with at CTRC are interested in ways to accurately characterize properties quickly.”
“We are so proud of these results,” Christov said. “We managed to contribute some really new fundamental knowledge about these materials that was lacking. This data-driven approach opens up a new way of characterizing these complicated soft materials.”
Writer: Jared Pike, jaredpike@purdue.edu, 765-496-0374
Source: Ivan Christov, christov@purdue.edu
Amy Marconnet, marconnet@purdue.edu
Data-driven rheological characterization of stress buildup and relaxation in thermal greases
Pranay P. Nagrani, Ritwik V. Kulkarni, Parth U. Kelkar, Ria D. Corder, Kendra A. Erk, Amy M. Marconnet, and Ivan C. Christov
https://doi.org/10.1122/8.0000679
ABSTRACT: Thermal greases, often used as thermal interface materials, are complex paste-like mixtures composed of a base polymer in which dense metallic (or ceramic) filler particles are dispersed to improve the heat transfer properties of the material. They have complex rheological properties that impact the performance of the thermal interface material over its lifetime. We perform rheological experiments on thermal greases and observe both stress relaxation and stress buildup regimes. This time-dependent rheological behavior of such complex fluid-like materials is not captured by steady shear-thinning models often used to describe these materials. We find that thixo-elasto-visco-plastic (TEVP) and nonlinear-elasto-visco-plastic (NEVP) constitutive models characterize the observed stress relaxation and buildup regimes, respectively. Specifically, we use the models within a data-driven approach based on physics-informed neural networks (PINNs). PINNs are used to solve the inverse problem of determining the rheological model parameters from the dynamic response in experiments. These training data are generated by startup flow experiments at different (constant) shear rates using a shear rheometer. We validate the “learned” models by comparing their predicted shear stress evolution to experiments under shear rates not used in the training datasets. We further validate the learned TEVP model by solving a forward problem numerically to determine the shear stress evolution for an input step-strain profile. Meanwhile, the NEVP model is further validated by comparison to a steady Herschel–Bulkley fit of the material’s flow curve.