BME's Culurciello aims to propel machine intelligence to the next level
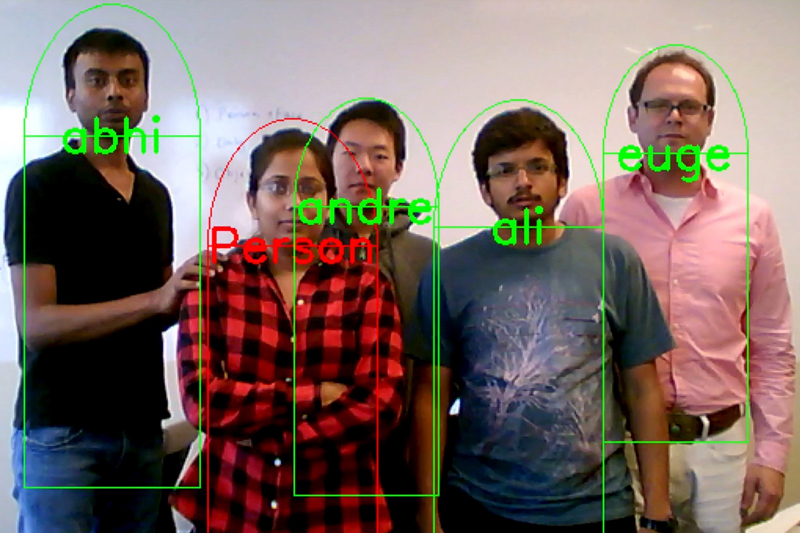
FWDNXT, based in the Purdue Research Park, has developed a low-power mobile coprocessor called Snowflake for accelerating deep neural networks effective at image recognition and classification. Snowflake was designed with the primary goal of optimizing computational efficiency by processing multiple streams of information to mix deep learning and artificial intelligence techniques with augmented reality application.
“Everybody was looking for a solution like this,” said Eugenio Culurciello, an associate professor in the Weldon School of Biomedical Engineering at Purdue. “We have a special computer that can operate on large data very fast with low power consumption. Our mission is to propel machine intelligence to the next level.”
Culurciello says the goal of FWDNXT is to enable computers to understand the environment so computers, phones, tablets, wearables and robots can be helpful in daily activities. FWDNXT uses innovative algorithms to differentiate items the same ways humans do.
Culurciello says Snowflake is able to achieve a computational efficiency of more than 91 percent on entire convolutional neural networks, which are the deep learning model of choice for performing object detection, classification, semantic segmentation and natural language processing tasks. Snowflake also is able to achieve 99 percent efficiency on some individual layers.
FWDNXT has shown expertise in scene analysis and scene parsing, which allows the computer to perceive the outside environment. That ability is among the most difficult challenges in augmented reality content, Culurciello says.
FWDNXT’s innovation in hardware and software will be used to drive cars autonomously, to recognize faces for security and other purposes and numerous other day-to-day purposes, such as helping people find items on their shopping lists as they walk down a store aisle or smart appliances recognizing a user’s preferences.
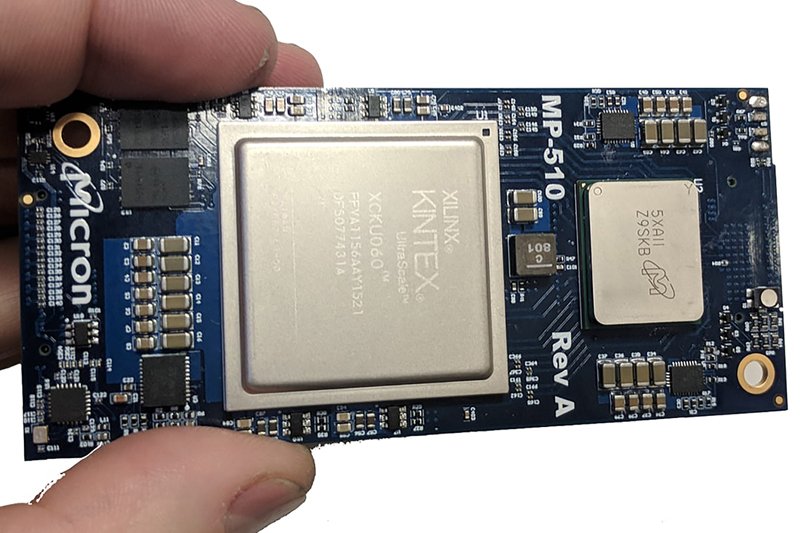
Culurciello says there has been remarkable development in machine learning in the past five years as computer scientists turned to specialized chips to do more complex computing instead of depending on a central processing unit.
“It can operate on large data very fast with low power consumption,” Culurciello said. “We want to have the maximum performance with the minimal energy.”
FWDNXT was able to create a new, efficient computer architecture with funding originally from grants it received from Purdue and the Navy, including one worth nearly $1 million.
Culurciello says FWDNXT wants to make microchips that will be used in virtually all smart device — for instance, in cars to start them, in appliances to recognize persons’ preferences, in mobile phone to listen to voices.
Culurciello says FWDNXT has found a strategic partner, has obtained multimillion dollars in funding, and the next step is to seek Series A funding. FWDNXT also is looking to add to its team, which already includes Ali Zaidy, the lead architect designer of Snowflake and a deep learning expert; Abhishek Chaurasia, the team’s deep learning lead developer; Andre Chang, architect and compiler of deep learning; and Marko Vitez, neural network optimization wizard.
Another big milestone will be to develop a prototype microchip due in the first half of 2018. FWDNXT has shown it can run on FPGA, but a custom microchip would make it even more efficient.
FWDNXT hopes to be selling programmable logic prototypes soon and hopes to be able to sell the initial microchips to preferred customers in the next year or so.
FWDNEXT has filed patent applications related to Snowflake through the Purdue Office of Technology Commercialization. Culurciello also credits the Purdue Foundry, an entrepreneurship and commercialization accelerator in Discovery Park’s Burton D. Morgan Center for Entrepreneurship at Purdue, with helping FWDNXT come up with a business plan that will help attract investors and expand its team.