Purdue Prof. Murat Kocaoglu wins NSF SMALL Award
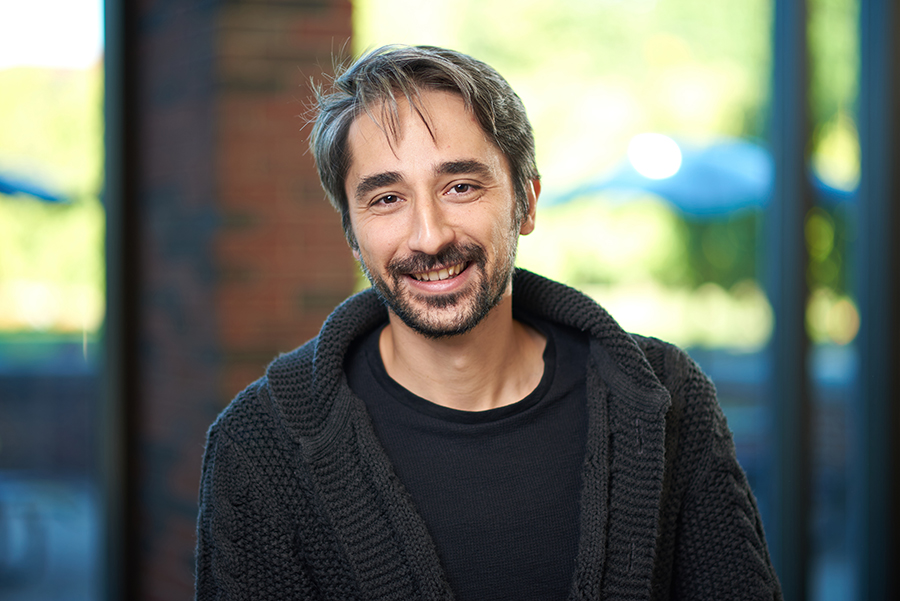
Murat Kocaoglu, Assistant Professor in Purdue University’s Elmore Family School of Electrical and Computer Engineering, has won an NSF SMALL Award for his work on Causal Structure Discovery from Diverse Data.
Causal reasoning from data is critical in many domains, from medicine to computer software security. Recently, the role of causality in machine learning (ML) has been understood through ML solutions' overreliance on correlations, resulting in a lack of generalizability. To tackle this problem, researchers train models with data from multiple environments to extract useful features across domains. Other studies suggested that the causal relations between features can be leveraged to train robust models that generalize.
However, unlike these ML methods that can use any collection of datasets, most of the existing causal discovery algorithms heavily rely on the assumption that we have access to interventional data, such as those from a randomized controlled trial. In practice, the datasets from different environments may carry common causal knowledge but not necessarily arise due to well-defined interventions. Methods to systematically extract such common causal knowledge across domains from data is currently missing. This prevents ML solutions from leveraging causal structure explicitly.
This project aims to address this gap by developing novel algorithms that can extract cause-effect relations from unstructured, diverse datasets. The project outcomes are expected to unlock the potential of causal reasoning for data-rich domains with access to data from different environments and significantly widen the use of causal discovery among ML practitioners. Kocaoglu says he is grateful for the NSF’s support to pursue his research vision.
“I believe the outcomes of this project may unlock the use of unstructured, passively collected datasets for reasoning about cause-effect relations,” he says. “That means we may be able to evaluate the causal effect and use it in machine learning/AI tasks and for decision-making without having to collect experimental data from randomized controlled trials in data-rich domains such as healthcare and cloud computing.”
The research project will be conducted in three main thrusts. The first thrust will focus on characterizing the fundamental limits of causal knowledge extraction from diverse datasets under minimal assumptions about the data generating process. In the second thrust, the team will develop causal discovery algorithms to achieve these fundamental limits from such diverse datasets. Finally, the proposed discovery algorithms will be rigorously evaluated across a wide range of datasets, demonstrating their performance on downstream ML tasks enabled through the learned causal structure.
The grant comes from the Division of Information and Intelligent Systems (CISE/IIS) through the Robust Intelligence (RI) program. The RI program supports research in all aspects of the computational understanding and modeling of intelligence in complex and realistic contexts. RI systems are characterized by flexibility and resourcefulness and the use of a variety of modeling or reasoning approaches, demonstrating a high level of intelligence and adaptability.