Paper by PhD student Liming Wu earns recognition at CVPR 2021
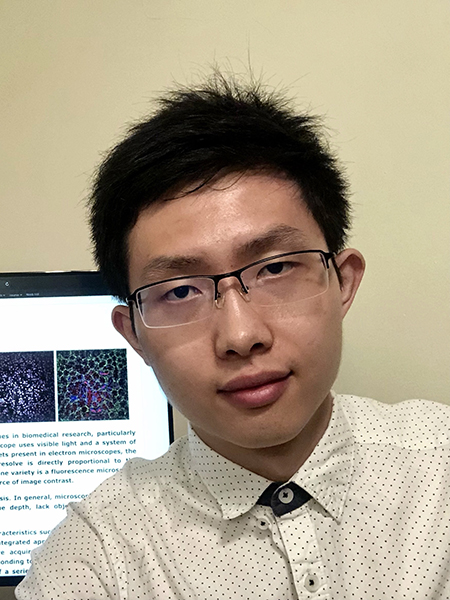
Liming Wu, a PhD student in Purdue University’s School of Electrical and Computer Engineering, has won a runner-up award for Best Paper at the Computer Vision for Microscopy Image Analysis (CVMI) Workshop at the 2021 IEEE International Conference on Computer Vision and Pattern Recognition (CVPR). Wu is a student of Edward J. Delp, the Charles William Harrison Distinguished Professor of ECE. Titled “RCNN-SliceNet: A Slice and Cluster Approach for Nuclei Centroid Detection in Three-Dimensional Fluorescence Microscopy Images,” the paper details research into how robust and accurate nuclei centroid detection is important for the understanding of biological structures in fluorescence microscopy images.
Existing automated nuclei localization methods face three main challenges: (1) Most of object detection methods work only on 2D images and are difficult to extend to 3D volumes; (2) Segmentation-based models can be used on 3D volumes but it is computational expensive for large microscopy volumes and they have difficulty distinguishing different instances of objects; (3) Hand annotated ground truth is limited for 3D microscopy volumes. To address these issues, the paper presents a scalable approach for nuclei centroid detection of 3D microscopy volumes. It describes the RCNN-SliceNet to detect 2D nuclei centroids for each slice of the volume from different directions and 3D agglomerative hierarchical clustering (AHC) is used to estimate the 3D centroids of nuclei in a volume. The model was trained with the synthetic microscopy data generated using Spatially Constrained Cycle-Consistent Adversarial Networks (SpCycleGAN) and tested on different types of real 3D microscopy data. Extensive experimental results demonstrate that the proposed method can accurately count and detect the nuclei centroids in a 3D microscopy volume.
By making use of RCNN, this system can easily distinguish touching nuclei without any post-processing steps such as watershed or morphological operation. This method can better capture small nuclei and partially included nuclei on the border. The slice-and-cluster strategy is robust handling the errors from RCNN-SliceNet's misdetections on some slices. The 3D AHC will treat RCNN-SliceNet's misdetection as outliers and 3-way majority voting will further align the centroid location and remove outliers. These methods achieved better results than segmentation-based methods on ellipsoidal nuclei data.
CVPR is the premier annual computer vision event comprising the main conference and several co-located workshops and short courses. The 2021 event was held virtually and featured presentations, tutorials, workshops, and panels delivered by leading authors, academics, and experts.