Mathematical models try to beat the spread
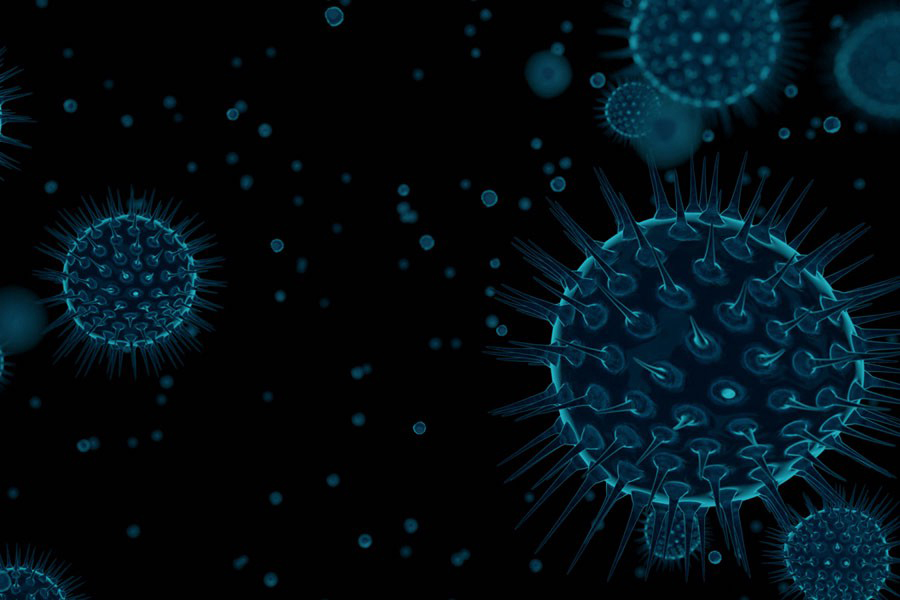
How do we best understand the spread of COVID-19 in order to institute the proper controls to mitigate the virus? Mathematical modeling provides a systematic way, based on fundamental principles and more than a century of research, to try to capture the behavior of the viral reach. Accurate models of COVID-19 can help decision makers predict the epidemic’s course, while provable algorithms can offer guidance on how to alleviate the spread.
In a very basic sense, an algorithm is a systemic set of decisions, based on the current situation, that adjusts iteratively as the algorithm progresses through its analytic data crunching. In the context of epidemics, an algorithm could guide decision makers on how and where to allocate resources, such as ventilators and vaccines.
Typically, due to data limitations, epidemic models partition an entire population into different compartments. For example, in a so-called “SIR” model, each member of the population is placed in the susceptible (S), infected (I), or removed (R) compartment. So, everyone in the population fits into one of these three compartments at any particular point in time.
The problem is that these models assume instantaneous and equal mixing of the whole population. Human behavior, however, is more complicated, with different types of interactions between different groups, communities, and/or subpopulations that may change over time.
These interactions between different members of the population, and the virus spread itself, can be modeled as a networked dynamical system — a system in which different entities, or nodes, are connected, and the state associated with each entity changes over time. An example would be sickness levels of different communities in an epidemic.
A dynamic networked compartmental model lets every subpopulation, or network node, have a set of compartments, and then allows interaction both within that subpopulation and with other subpopulations. The interactions between these subpopulations during the epidemic can then be modeled in different ways — e.g., via shared borders or travel data.
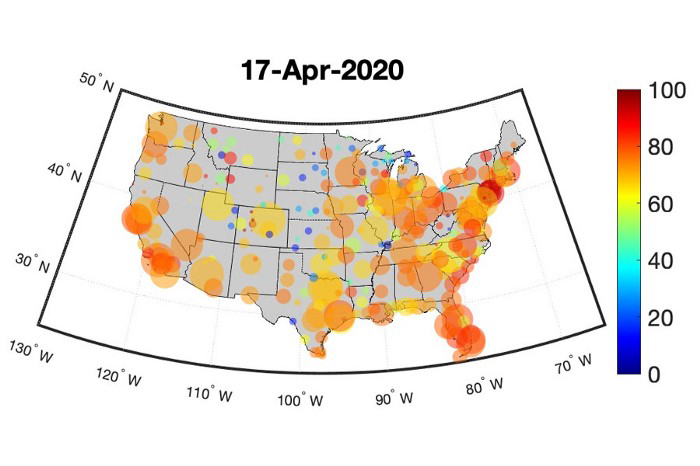
One mechanism for virus movement between spatially separated populations is transportation networks. Transportation data, combined with networked compartmental models of epidemic processes, can be used to improve models of the spread of COVID-19. My group is validating our transportation-spread model with real data to justify its use for mitigation algorithm design. We are collaborating with Professor Raphael Stern of the Department of Civil, Environmental, and Geo- Engineering at the University of Minnesota. Funding is provided through a National Science Foundation Rapid Response Research (RAPID) grant, via the NSF-CNS (Computer and Network Systems) Smart and Connected Communities program.
To deploy suitable control measures, it is key to understand the true prevalence of the disease in any population. However, testing data results do not reveal the true underlying epidemic states — because tests are delayed, biasedly sampled, and inaccurate — so we use statistical tools to estimate the underlying states from the testing datasets that are available. Validating the estimation work is difficult, as the true underlying states are unknown.
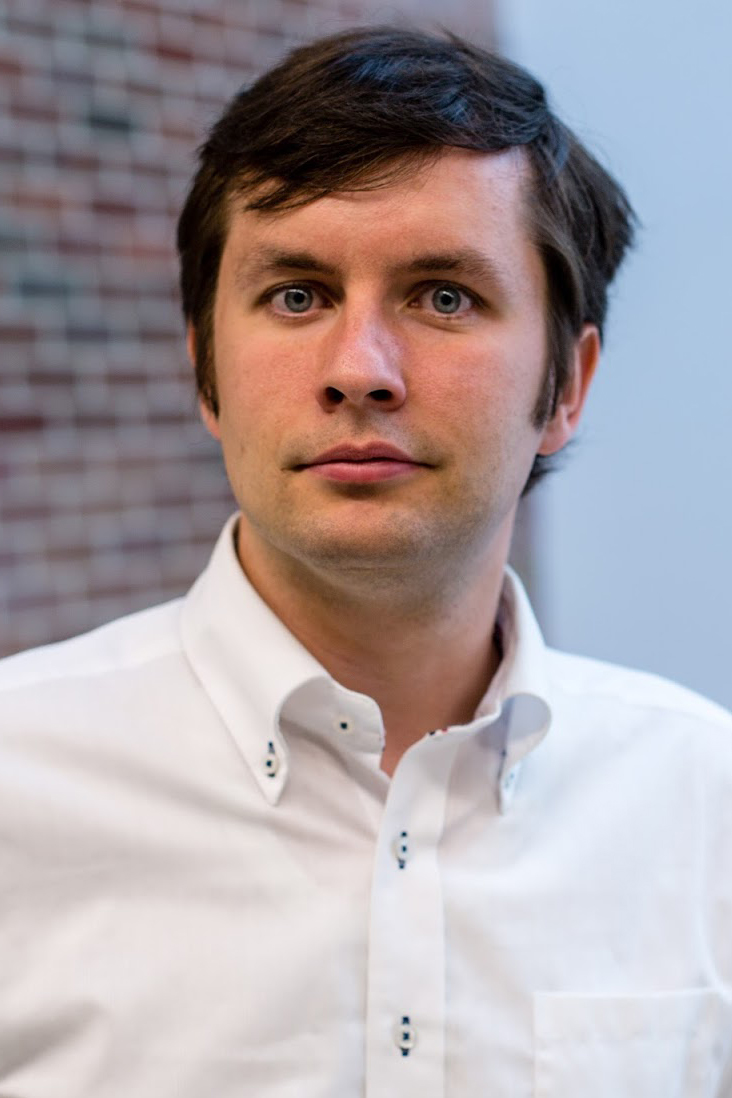
We are working with epidemiology and public health experts to get a better handle on this problem. This research is funded by the C3.ai Digital Transformation Institute, which is sponsored by C3.ai and Microsoft, and conducted in collaboration with Professor Ashish Hota of the Department of Electrical Engineering at the Indian Institute of Technology (IIT) Kharagpur (and a Purdue alum). My group also is working with the Protect Purdue data science team, aiming to provide tools to aid decision makers.
Beyond the coronavirus pandemic, we anticipate insights from our work will shed light on other important processes, such as the spread of influenza, opinion propagation in social media, and cascading failures in power networks.
We now know it is likely the world will have to live with the SARS-CoV-2 virus at least to some extent for a while, and new outbreaks may occur in the future. Therefore, maintaining and improving the tools we have developed and used will be vital to protecting society overall — because the pandemic is a public health, economic, and environmental issue.