NSF Supports $1M to Advance Adversarial Robustness in the Real World
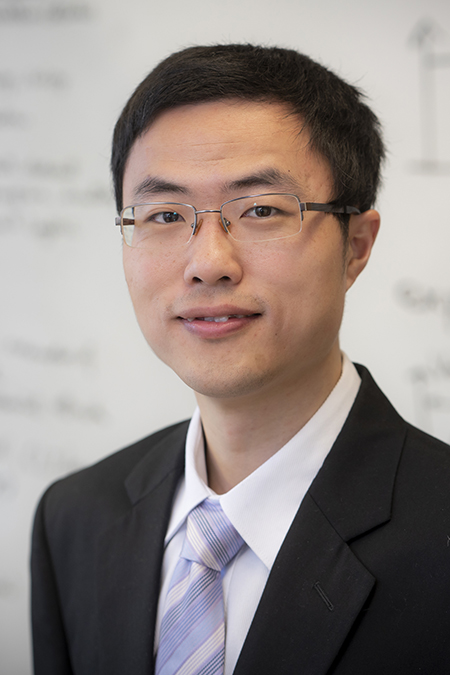
Purdue scientists and engineers have received $1M in support from the National Science Foundation (NSF) to advance the adversarial robustness of computer vision systems in the real physical world. The project, titled “Robust Deep Learning in Real Physical Space: Generalization, Scalability, and Credibility,” is funded through the NSF’s Stimulating Collaborative Advances Leveraging Expertise in the Mathematical and Scientific Foundations of Deep Learning (SCALE MoDL) program. The research will be conducted by Stanley Chan, Elmore Associate Professor in Purdue’s Elmore Family School of Electrical and Computer Engineering, Guang Lin (Purdue Math and ME), Jean Honorio (Purdue CS), Guang Cheng (Purdue Statistics), and Yian Ma (UCSD).
Robust machine learning in real physical space requires co-modeling the deep neural networks and the environment in which the neural networks are operating. Research efforts focusing on one specific domain but not interacting with the other domain will unlikely solve the problem. The combination of skills in electrical engineering, statistics, and computer science possessed by the Purdue-UCSD team offers a unique opportunity to address the problem. The technical approach the team will take is to reformulate the robust adversarial learning problem by incorporating the environmental factors. Four specific research objectives will be pursued: (1) Parametrizing the physical environment via a hierarchy of deterministic and generative approaches, so that the set of all possible distortions can be constrained. (2) Analyzing the generalization bounds of neural networks in the presence of the environmental factors and analyzing the credibility of such a system by studying the robustness and uncertainty quantification. (3) Developing computationally efficient algorithms to seek the equilibrium points of a proposed minimax optimization. (4) Building a computational photography testbed to implement the concepts and validate the theoretical results. On the educational front, the project provides a suite of outreach activities to K-12 to improve their interest in STEM, and research opportunities to undergraduates.
The National Science Foundation’s (SCALE MoDL) program is a multidisciplinary program that provides unique funding opportunities to engineers and scientists to address the mostly needed research questions in deep learning.