App snaps of meals keep your diet on track
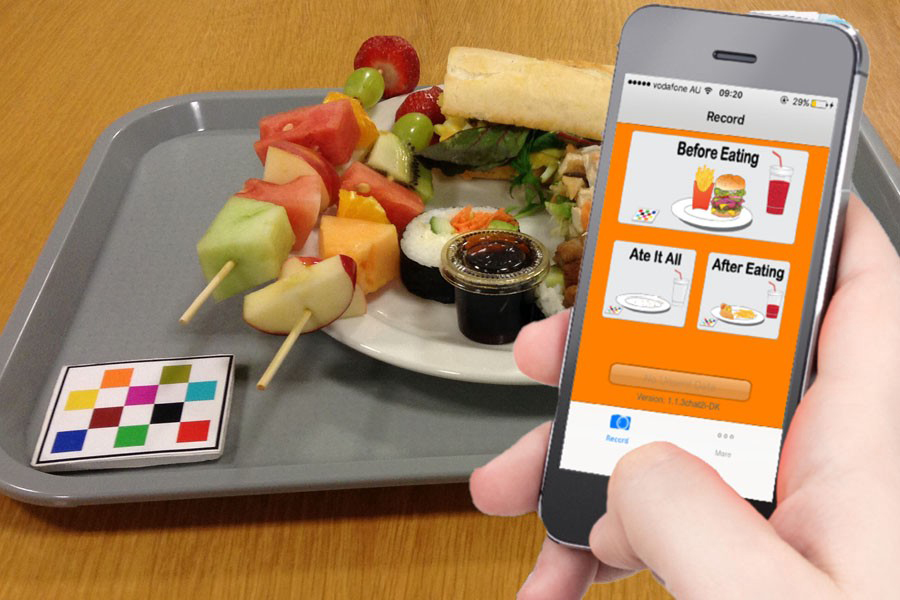
Good nutrition is not only essential for healthy development and basic survival, but also integral to well-being and disease prevention. Health conditions linked to poor diet constitute the most frequent and preventable causes of death in the U.S. and are major drivers of healthcare costs, estimated in the hundreds of billions of dollars annually. While diet needs to be understood in order to be improved, dietary intake remains difficult to measure.
Our research group has designed and developed one of the first mobile, image-based dietary assessment systems that records and analyzes images of eating occasions to accurately measure daily food and nutrient intake. New, unbiased data-capture methods, such as mobile dietary assessment, are inexpensive and customizable — meaning they could overcome the limitations of current approaches, which rely primarily on self-reporting.
Image-based dietary assessment refers to the process of determining what someone eats and how much energy is consumed, based on visual data and associated contextual information. We developed different computer vision and machine learning methods to automatically identify foods and estimate energy intake from a meal image. Our system has been deployed and evaluated in more than 30 dietary studies, with over 2,500 participants from age 6 months to 70 years, in U.S. and international locations.
For evaluating habitual dietary intake, an accurate profile of foods consumed and estimation of portion sizes are paramount. Self-reporting is the most common approach, yet accuracy depends on a person’s willingness to report and the ability to remember and estimate portion sizes. The current gold standard in dietary assessment is the 24-hour recall, in which participants are interviewed to help them remember everything consumed in the last 24 hours.
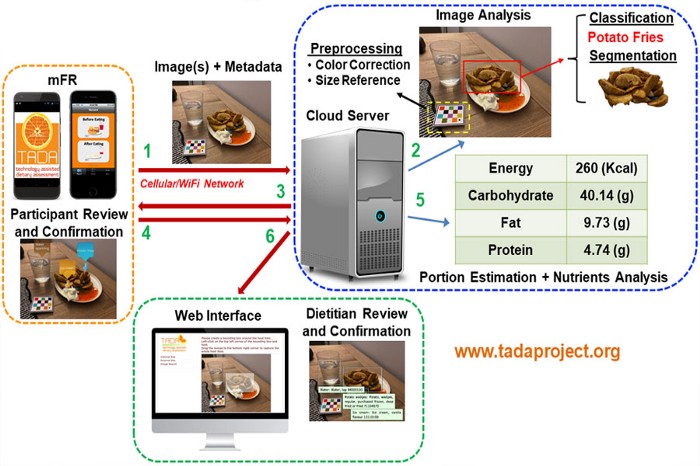
Our Technology Assisted Dietary Assessment (TADA™) system consists of two main components: 1) an image-based dietary recording app called a Mobile Food Record (mFR), and 2) a secure, cloud-based server that communicates with the mFR to process and store the food images. The mFR uses a smartphone camera to photograph images of food. These images are sent to the cloud server, along with metadata, including date, time, and GPS coordinates for analysis and processing; participants may review and confirm analyzed results on their smartphones. The image analysis results also can be viewed via a web interface by dietitians and researchers for evaluation and additional nutrient analysis.
This accurate estimation of dietary intake relies on the system’s ability to distinguish foods from image background, identify the foods, and estimate food portion sizes. Our novel machine learning technology, leveraging deep neural networks and other advanced computer vision approaches, integrates the food classifications and portion size estimates, reduces prediction errors for visually similar foods, maps food images to food energy, and continuously learns from the ongoing data capture and analysis.
The development of more accurate and user-friendly tools to capture information about a person’s diet will help researchers better understand dietary patterns and their effects on health. The focus is on dietary patterns rather than individual nutrients, foods or food groups, which are not consumed in isolation but rather in various combinations over time (a dietary pattern) as the foods and beverages act synergistically to affect health.
Our projects are supported by grants from the National Science Foundation and National Institutes of Health, as well as industry partners, and we have several patent applications under review. Many image processing and computational methods we have developed also could be used for other applications and problems that rely on image/video as input information, such as industrial product inspection, digital agriculture, and autonomous systems.
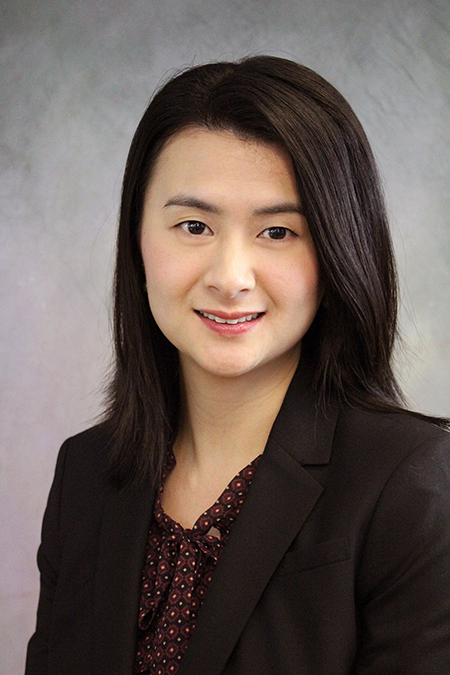
Mobile, wearable sensor technology, coupled with advanced algorithms, is showing increasing promise in its ability to capture diet-related behaviors that provide useful information for assessing dietary intake. Accurate assessment of dietary intake and behavior likely will require synergized effort from image-based sensing (cameras paired with new algorithms that detect and analyze foods); wearable sensing (body-worn sensors to capture eating and diet behaviors); and biochemical/electrochemical sensing (wearable technology that measures nutrient status from body fluids, such as saliva and sweat).
Today, new alliances between nutrition scientists, engineers, and data scientists to create novel tools and predictive algorithms present an unprecedented opportunity to untangle the various roles that whole foods, individual nutrients, sociocultural impacts on eating and lifestyle, and societal infrastructure play in the health of individuals and populations.
Source: App snaps of meals keep your diet on track