Purdue Prof. Christopher Brinton selected for prestigious DARPA Director's Fellowship
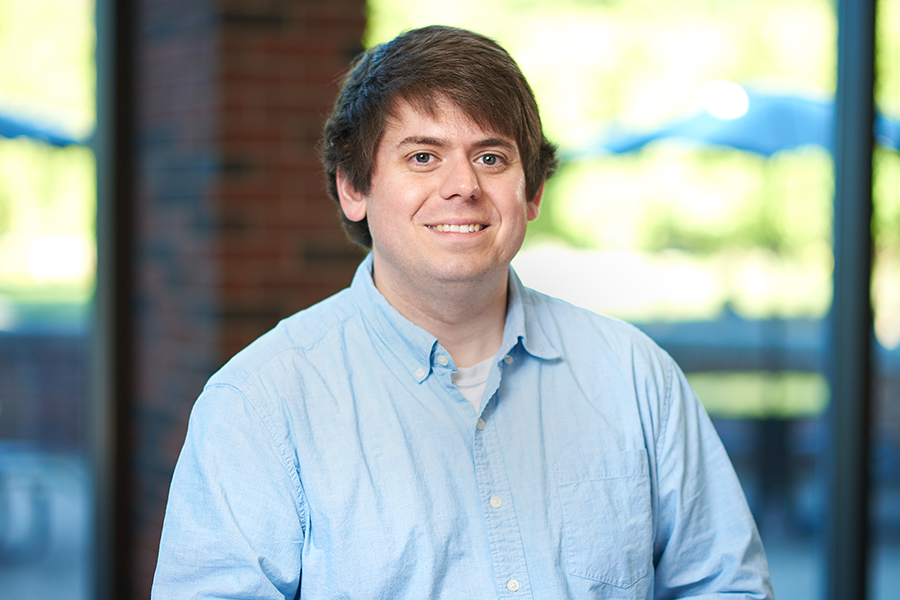
Christopher Brinton, Elmore Associate Professor in Purdue University’s Elmore Family School of Electrical and Computer Engineering, has been awarded the prestigious Director’s Fellowship from the Defense Advanced Research Projects Agency (DARPA). This is a highly competitive fellowship that provides additional funds for Brinton to continue the research from his DARPA Young Faculty Award (YFA).
Brinton won the YFA in 2022. This program aims to consistently establish and sustain groups of young scientists motivated to pursue high-risk, high-reward research by pairing them with DARPA program managers and providing them with funding for a two-year period. Winners are chosen in a range of research areas from engineering, physics, chemistry to computer and social science. The long-term goal of the YFA program is to develop the next generation of academic scientists, engineers, and mathematicians who will focus a significant portion of their career on DoD and National Security issues.
At the end of the initial two-year period for the YFA, awardees are eligible for the Director’s Fellowship, which is reserved for a small number of awardees who have demonstrated exceptional YFA project performance. The award extends his YFA research for a third year.
Brinton said it is an honor to have been selected for the Director’s Fellowship
“I am grateful to all the guidance I received from DARPA, especially my program mentors, in working on what we believe to be a very consequential research problem,” he said. “I look forward to using the funds from this award to further expand the state-of-the-art in distributed learning over non-terrestrial network systems and demonstrate the impact of our algorithms in intelligence applications spanning the commercial and defense sectors.”
Brinton’s project, titled “FL-NTN: Fog Learning Orchestration of Heterogeneous Model Training across Hybrid Terrestrial and Non-Terrestrial Network Systems,” investigates the integration of non-terrestrial network (NTN) satellite constellations into global intelligence systems. Existing techniques for supporting the growth in machine learning (ML) capabilities focus on terrestrial network infrastructure, placing additional resource burdens on this infrastructure and missing datasets captured in remote locations with insufficient access to the core network. Contemporary NTNs offer several unique characteristics, including wide coverage areas and satellite constellation topologies, presenting an opportunity to scale up distributed ML training and synchronization on a global scale. This project establishes a novel architecture for learning over hybrid terrestrial and NTN systems, jointly optimizing intelligence quality and resource efficiency.