Purdue alumnus Debasmit Das wins 2024 Eaton Award in Design Excellence
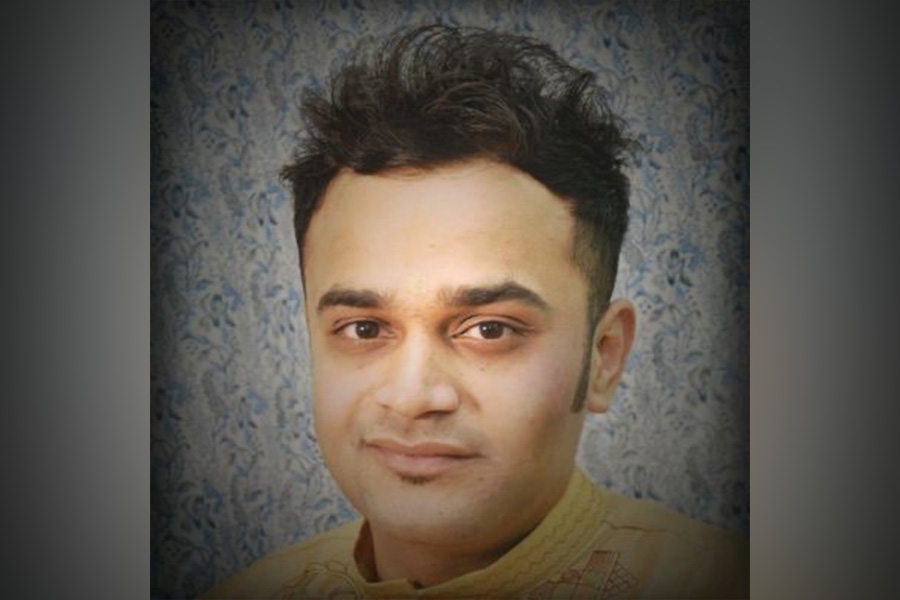
Debasmit Das, a rising star in the field of machine intelligence, has been awarded the prestigious 2024 Eaton Award in Design Excellence from Purdue University's Elmore Family School of Electrical and Computer Engineering (ECE). This award, which honors recent ECE alumni for outstanding work in design, is a testament to Das’s contributions to the field of Transfer Learning. Das earned his PhD from Purdue ECE in 2020.
The Eaton Award in Design Excellence was established through the generous support of Jim and Shirley Eaton. Jim Eaton, who earned his BSEE in 1958, MSEE in 1963, and Ph.D. in 1967, is a proud alumnus of Purdue, as was his father, a well-known faculty member in the School from 1942 to 1967. The award aims to recognize early achievements in design and to encourage young engineers to continue pushing the boundaries of innovation.
Das said he is honored to receive the 2024 Eaton Award.
“I am proud to be an alumnus of Purdue, which provided such a wonderful environment for doing research on artificial intelligence,” said Das. “For the future, I am looking into personalization of generative models both language and vision.”
Das's research in Transfer Learning has been groundbreaking, particularly in the areas of domain adaptation and small-sample learning. During his time at Purdue, his thesis work focused on these challenges, which are critical in adapting existing machine learning models to new environments that may be limited in computational resources or data availability. His novel approaches, such as graph matching for minimizing distribution shifts between different environments, have been published in leading conferences and journals like ICIP, ICANN, and EAAI. This work has garnered over 180 citations, highlighting its significant impact on the research community.
In addition to his work on domain adaptation, Das developed a few-shot learning framework that predicts data statistics and produces a low-dimensional feature space, published in the IEEE Transactions on Image Processing (TIP) journal. He also contributed to zero-shot learning, publishing his findings in conferences like IJCNN and SMC, which have collectively received more than 85 citations. These contributions have been supported by NSF Grants, underscoring the competitive and impactful nature of his research.
Das’s journey continued at Qualcomm AI Research, where he has been instrumental in advancing transfer learning for user personalization applications. His work has spanned critical areas such as biometric security, including face and fingerprint anti-spoofing, and speaker verification, with publications in top-tier conferences like WACV, ICLR, and INTERSPEECH. His innovative methods in self-supervised learning, parameter initialization, and scene understanding have also been recognized in conferences like NeurIPS, ICLR, and CVPR.
Beyond his research, Das has actively contributed to the academic and research community through extensive reviewing, editorial duties, and organizing sessions at international conferences. His role as an associate editor for IEEE Transactions on Computational Social Systems and Wiley Applied AI Letters, coupled with his involvement in 200+ reviewing and organizational activities, underscores his dedication to advancing the field.
With over 20 publications, 10+ patents filed, and close to 400 citations, Debasmit Das is a deserving recipient of the 2024 Eaton Award in Design Excellence. His work at Purdue and beyond has already left a lasting impact on the field of machine intelligence, and his future contributions are eagerly anticipated by the academic and professional communities alike.