Prof. Murat Kocaoglu receives NSF CAREER Award
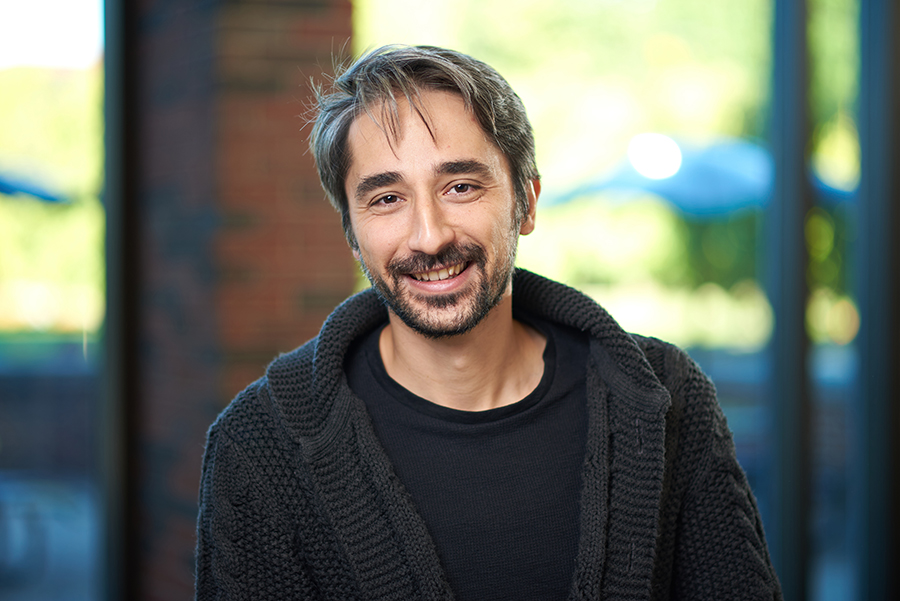
Murat Kocaoglu, assistant professor in Purdue University’s Elmore Family School of Electrical and Computer Engineering, has received a National Science Foundation (NSF) CAREER Award. The awards, presented once each year, include a federal grant for research and education activities for five consecutive years. Kocaoglu’s grant will support a project entitled “Optimism in Causal Reasoning via Information-theoretic Methods.”
CAREER awards are in support of junior faculty who exemplify the role of teacher-scholars through research and education, and the integration of these endeavors in the context of their organizations' missions. Kocaoglu says he is grateful to the NSF for the support for his project, which explores the fundamental problem of causes and effects in the development of artificial intelligence. As an alternative to current algorithms that use worst-case scenario, the project will extend the theory of causation to a much wider set of real-world instances by enabling causal reasoning for most models rather than in the worst case. Kocaoglu will develop novel algorithms that identify information-theoretically simple explanations of the underlying causal system from data.
"With this project, our lab will work on bringing information theory into every step of the causal reasoning pipeline,” says Kocaoglu. “Our goal is to develop practical algorithms in settings where some of the standard assumptions made in the causality literature may only hold approximately, which can be quantified and incorporated into causal reasoning algorithms using information-theoretic tools and quantities. This has the potential of significantly expanding the scope and applicability of causality in the real world."
The first thrust seeks to develop methods to learn causal relations from observational data via an information-theoretic interpretation of Occam’s razor based on the entropy of the causal system. A second thrust will analyze how information-theoretically simple explanations can help approximately compute causal effects that are not identifiable in the worst case. A third thrust will leverage the results of the first two thrusts to develop experimental design algorithms for efficiently learning causal structures and causal effects via interventions.