The 'fog' of learning
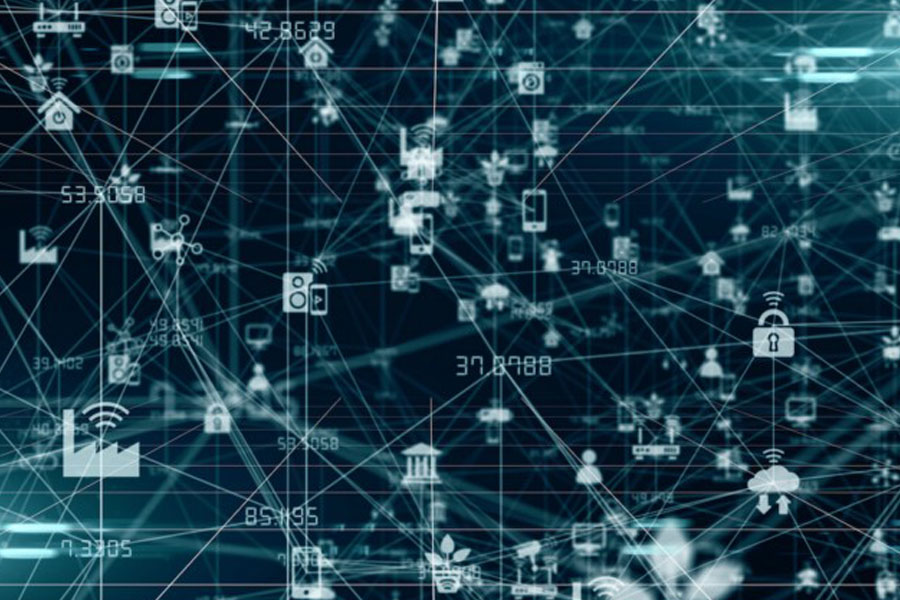
A fog is blanketing computing. Yet it’s not obscuring things; instead, it’s revealing them more clearly. This is fog computing, a decentralized paradigm in which data is processed throughout networks, not only in servers but also at individual devices — the “edges” of the network — and at all nodes in this pervasive, computational “fog” between the edges and the cloud. The benefits are twofold: 1) faster computing, because when you process at the edge, you don’t wait for data to travel to the servers, and 2) cost savings, via less processing power and storage in the data centers or cloud.
Piggybacking on fog computing, fog learning is a new approach to enabling machine learning — the branch of artificial intelligence that lets you sort data at blazing speeds to identify patterns, gain insights, and automate actions.
Machine learning models have to be trained to perform their magic. Fog learning parcels out the training across local network points, from Internet of Things (IoT) devices to servers in cloud datacenters, accounting for differences in compute and communications capability and network proximity. Smart device collaborations pass messages and form consensus among locally trained models, aggregating local learning and “slimming” the data before sending it to servers for global consolidation into updated models, which are then synchronized across the local devices at the network edge.
These IoT devices, in effect, form ad hoc networks within the overall fog network — learning clusters that share training model parameters, and progressively lessen the amount of data sent to the server repositories. This compresses model training times, for quicker execution of data-intensive, time-sensitive tasks — think sudden braking in an autonomous vehicle. The “device-to-device” (D2D) communications driving these ad hoc networks also can reduce device energy consumption substantially compared with upstream and downstream transmissions — crucial for battery-constrained gadgets like smartphones, unmanned aerial vehicles, and wireless sensors.
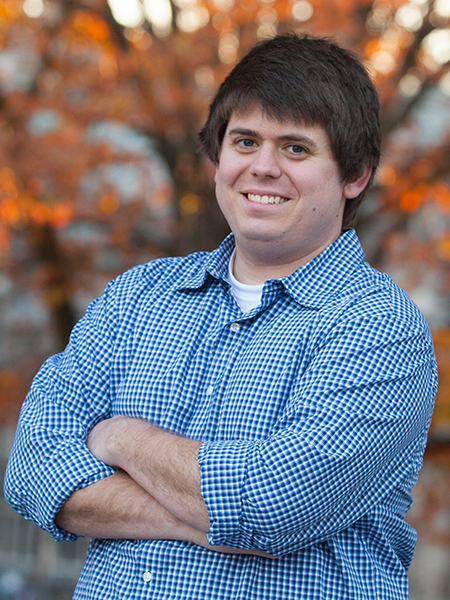
Human learning and machine learning go hand in hand. Today’s networks generate and send tons of data to users as they interact with their devices — at the same time social and communication networks are revolutionizing when and how learning takes place. The fine-grained behavioral data created as users interact with online content, and with one another in their social learning networks, provides an entrée to personalize human learning via machine learning intelligence, embedded in such connected devices as smartphones, tablets and laptops.
To advance this synergy between human and machine learning, I’m working on an initiative to provide analytics for online courses. This is especially vital as the coronavirus pandemic and resultant emphasis on remote instruction have transformed online human learning into one of the most important applications for fog learning.
Virtually every device today — from the smartphone in your pocket to the sensors in homes, infrastructure, factories and fields — is collecting data and computing. Fog learning — distributing machine learning across this fog of computing — advances the progress of dynamic, adaptive, and ever-more- intelligent networks, to help make sense of the big data that suffuses our lives.
Christopher G. Brinton, PhD
Assistant Professor, Elmore Family School of Electrical and Computer Engineering
College of Engineering
Purdue University
Associate Editor, IEEE Transactions on Wireless Communications
Source: The 'fog' of learning