ECE researchers develop quantum machine learning algorithm for optimizing arbitrary engineering problems
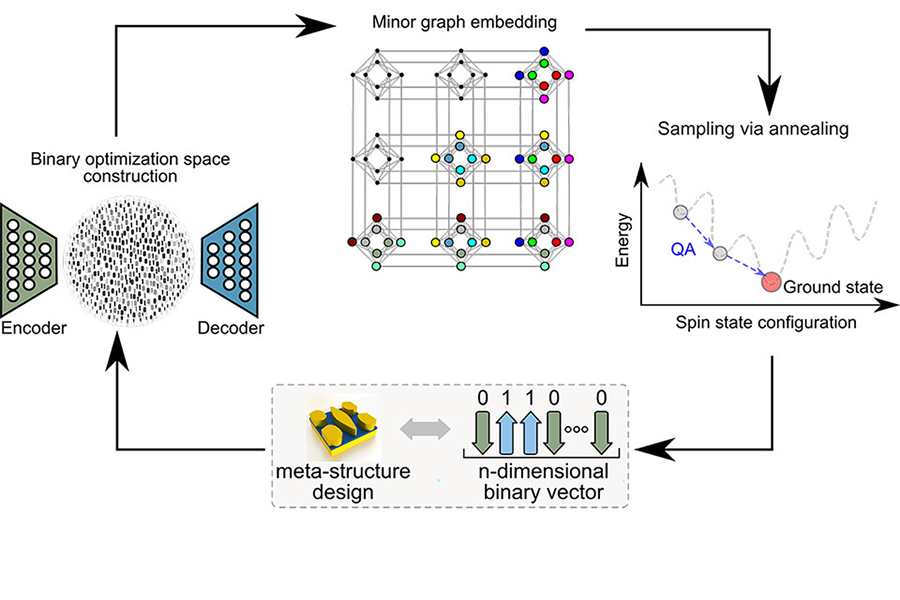
Researchers with Purdue University’s Elmore Family School of Electrical and Computer Engineering developed an efficient quantum algorithm for optimizing arbitrary engineering problems. Blake Wilson, a Ph.D. student working with the Shalaev, Boltasseva, Kildishev, and Kais Research Groups, says this new algorithm uses machine learning to convert any engineering design problem into one that a quantum computer can optimize.
Wilson says the unique thing about this research is the compression of the design problem into an Ising Hamiltonian for the quantum computer to optimize. He says in the past the only problems that could be considered were those whose structure already matched the Ising Hamiltonian structure. However, this new method opens the door for any optimization problem to be converted into an Ising Hamiltonian using machine learning and sampling.
“Our machine learning-based framework is very general,” says Wilson. “It makes no assumptions about what kind of problem you’re working on. All you do is give it a data set of random designs for whatever problem you’re working on and it takes over from there.”
The group demonstrated the framework’s performance by first optimizing thermal emitter topologies for thermophotovoltaic applications. They then optimized diffractive meta-gratings for highly efficient beam steering. Wilson says the technique can scale to solve advanced inverse design problems for science and engineering applications, for instance, the shape of an airplane or the curvature of an aerodynamics problem.
The researchers aim to implement a similar algorithm on a gate model quantum computer and to improve the accuracy of the compression scheme using more advanced data science techniques. Additionally, they’ve already started working to incorporate the partial differential equations governing the engineering problem into the optimization scheme to exploit the problem structure.
In addition to Wilson, the research group includes Zhaxylyk A. Kudyshev, a former postdoctoral research fellow with the Birck Nanotechnology Center, Alexander V. Kildishev, Associate Professor of Electrical and Computer Engineering, Sabre Kais, Distinguished Professor in Purdue’s Department of Chemistry, Vladimir M. Shalaev, Bob and Anne Burnett Distinguished Professor of Electrical and Computer Engineering, and Alexandra Boltasseva, Ron And Dotty Garvin Tonjes Professor Of Electrical and Computer Engineering.
This work was supported by the U.S. Department of Energy (DOE), Office of Science through the Quantum Science Center (QSC), a National Quantum Information Science Research Center, and Purdue's Elmore ECE Emerging Frontiers Center “The Crossroads of Quantum and AI.”
Source: Framework maps continuous-space inverse design problems for compressio