New advances in adversarial attack using computational optics
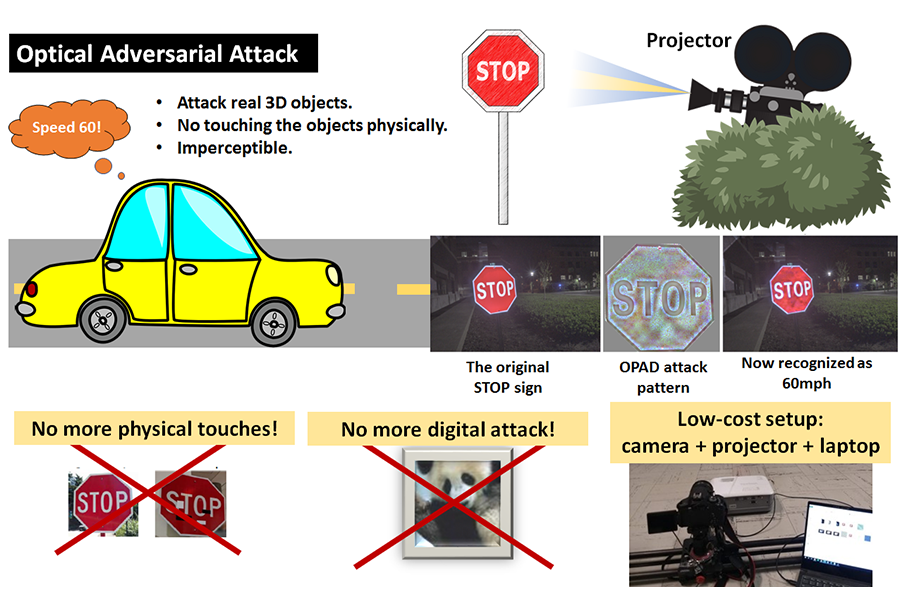
Have you imagined if a stop sign can be hacked to make a car to think that the sign is Speed limit 60mph? Stanley Chan, Elmore Associate Professor in Purdue University’s Elmore Family School of Electrical and Computer Engineering, and his PhD student Abhiram Gnanasambandam made this possible --- using a low-cost projector camera system.
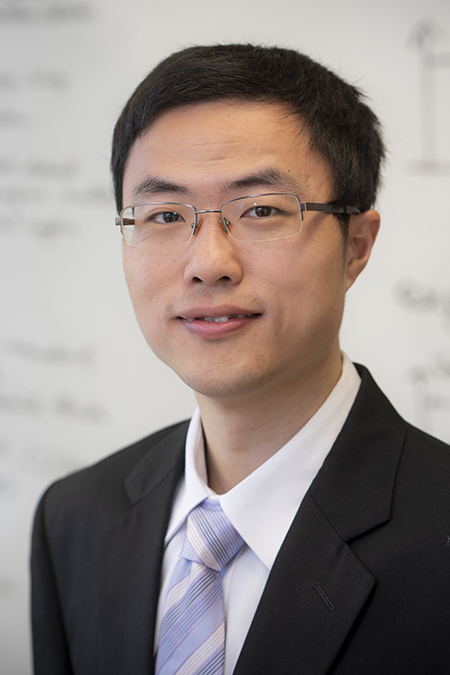
Adversarial attack is an important branch in computer vision and machine learning where people study the robustness of object recognition systems. Past research has demonstrated the feasibility of attacking digital images where an image is downloaded and manipulated on a computer. Attacking physical objects have also been demonstrated, but often the objects are physically altered such as painting symbols and shapes over the objects. A purely optical attack that does not touch the object is substantially more challenging. The two major challenges are that the environment attenuates the projector signal, and the scene responds to the illumination nonlinearly. For example, a pure red signal will appear pink as it is displayed on the object.
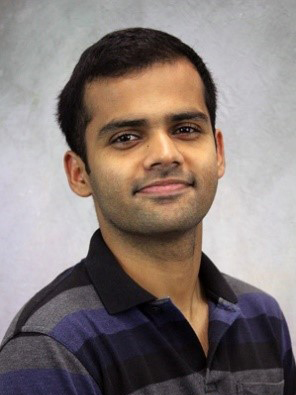
The main innovation of the work is the integration of computational photography techniques and deep learning theory. The researchers spent several months to convert the classical radiometric response model and the spectral response model into a forward image degradation model. They then integrated the degradation model with the adversarial attack optimization to derive the attack patterns. After calibrating the projector-camera system, the researchers were able to attack the object to any desired class in a single-shot. In addition, they also analyzed the theoretical properties of the problem by explaining why some objects are more easily attacked whereas some are more difficult to attack.
The work will be presented in the 2nd Workshop on Adversarial Robustness in the Real World, which is part of the 2021 IEEE International Conference on Computer Vision (ICCV). The co-authors also include Alex Sherman from the Department of Chemistry.
The research is originally supported by an EFC Seed grant from the College of Engineering, and later by the Army Research Office.
Manuscript available on arXiv: http://arxiv.org/abs/2108.06247