Lightning Talk Presenter
Ruizhe Zhang
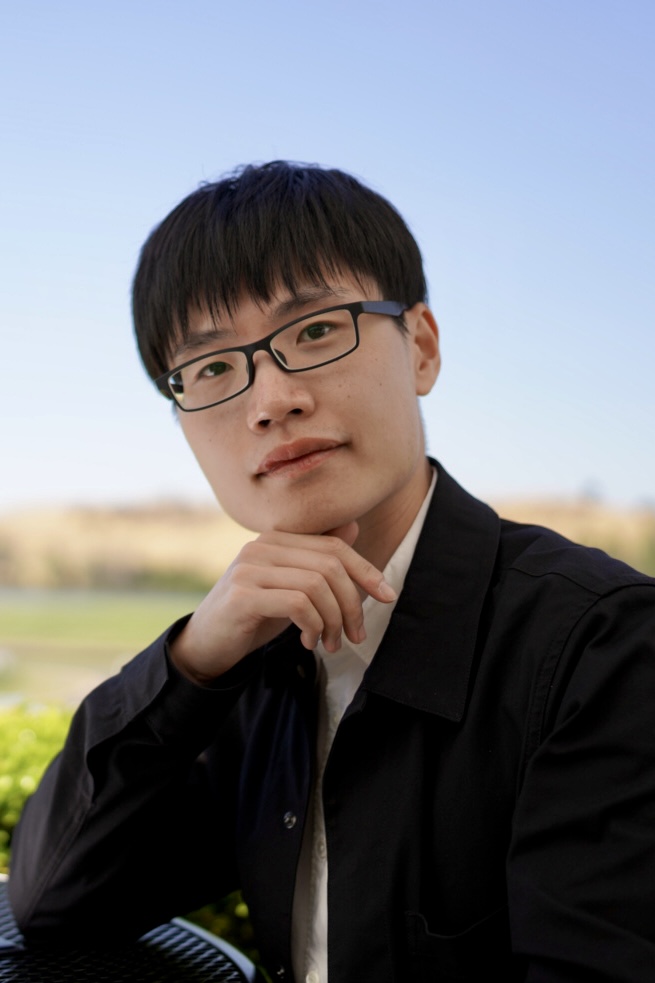
Ruizhe Zhang
Simons Institute for the Theory of Computing
About
Ruizhe Zhang is currently a Quantum Postdoctoral Fellow at the Simons Institute for the Theory of Computing at UC Berkeley. His research interests lie in the theoretical and algorithmic foundations of quantum computing, optimization, and deep learning. He received his Ph.D. in Computer Science from the University of Texas at Austin, advised by Dr. Dana Moshkovitz. He was the recipient of the University Graduate Continuing Fellowship at UT Austin.