Lightning Talk Presenter
Sanjaya Lohani
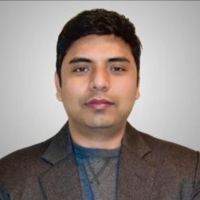
Sanjaya Lohani
University of Illinois Chicago
About
Dr. Sanjaya Lohani is a Senior Research Engineer at the University of Illinois at Chicago (UIC) in the USA. Before joining UIC, he worked as a fellow at the IBM-HBCU Quantum Center in Washington DC, and as a Postdoctoral AI Researcher at Tulane University in New Orleans. He earned his Ph.D. from Tulane University in May 2020.
Dr. Lohani's expertise lies in Artificial Intelligence (AI), Quantum Information Science and Computing, and Free Space Communication. His work involves staying at the forefront of the latest technologies. He has been recognized with the prestigious "Elizabeth Land Parks and Franklin Parks" fellowship for developing a computer artificial intelligence technique for free-space optical communications. Additionally, he has received awards such as the Incubic-Milton Chang Award, Emil Wolf Outstanding Finalist, and the Materials Computation Center (MCC) Award.
His research combines quantum information science, physics, engineering physics, and computer science. Dr. Lohani's current projects focus on creating innovative solutions for the broader research community, including artificial intelligence, algorithms for quantum computing, quantum communication and networking, and cutting-edge quantum technologies.