Lightning Talk Presenter
Debanjan Konar
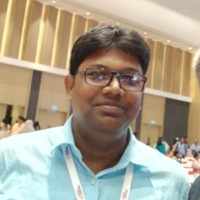
Debanjan Konar
Purdue University
About
Debanjan Konar earned his Ph.D. from the Indian Institute of Technology Delhi, New Delhi,
India. Currently, Dr. Konar is working as a Fulbright-Nehru Postdoctoral Fellow at Purdue
University. Prior to that, he was a Postdoctoral Research Scientist at Helmholtz Zentrum
Dresden-Rossendorf (HZDR), Dresden, Germany. He also worked as a Lead Research
Scientist (Quantum Computing) at BosonQ Psi Pvt. Ltd. (Bangalore), India. He was a
Helmholtz Visiting Fellow (Quantum Computing) at the Steinbuch Center for Computing
(SCC), Karlsruhe Institute of Technology (KIT) in Karlsruhe, Germany. His research interests
include quantum machine learning, hybrid classical-quantum algorithms, quantum-inspired
neural networks, etc. Dr Konar has authored several articles published in top-notch AI
journals and conferences. He is a Fulbright Fellow, a senior member of IEEE, an ACM
member, Euro-Science member. He has won many prestigious awards, like the Fulbright-
Nehru Postdoctoral Research Fellowships for 2023–2025, the Helmholtz Visiting Researcher
Grant, and many more.
Debanjan Konar, Ph.D.
Fulbright-Nehru Postdoctoral Fellow (Quantum Computer Sc.),
Purdue Quantum Science and Engineering Institute (PQSEI), and
School of Industrial Engineering, Purdue University, West Lafayette, USA