2024 Mellichamp Lecture: Ruth Misener
Author: | Joshua Gonzalez |
---|---|
Event Date: | October 22, 2024 |
Speaker: | Dr. Ruth Misener |
Speaker Affiliation: | Imperial London College |
Time: | 3:00-4:15 p.m. |
Location: | FRNY G140 |
Contact Name: | Joshua Gonzalez |
Contact Phone: | 765-494-4365 |
Contact Email: | jgonzal@purdue.edu |
Open To: | Attendance required for ChE PhD students |
Priority: | No |
School or Program: | Chemical Engineering |
College Calendar: | Show |
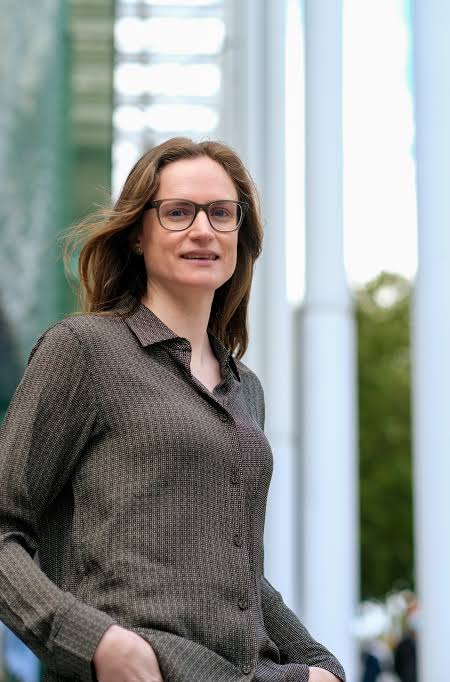
Dr. Ruth Misener
Professor in Computational Optimisation
Department of Computing,
Imperial College London
Hosts: Drs. Gintaras Reklaitis & Cornelius Masuku
Bio:
Dr. Ruth Misener is Professor of Computational Optimisation at Imperial College where she holds a BASF / Royal Academy of Engineering (RAEng) Research Chair in Data-Driven Optimisation. In 2017, she received the MacFarlane Medal as overall winner of the RAEng Young Engineer Trust Engineer of the Year competition. Dr. Misener also received the 2023 Roger Needham Award from the British Computing Society. Her best paper awards are from the: Journal of Global Optimization (2013), International Conference on Autonomous Agents & Multi-Agent Systems (AAMAS 2020, Best Innovative Demo), Conference on the Integration of Constraint Programming, Artificial Intelligence, & Operations Research (CPAIOR 2021), Optimization & Engineering (2021), and Computers & Chemical Engineering (2023). She has given named lectures at Princeton University (2023 Saville Lecture) and Georgia Institute of Technology (2018 Mellichamp Lecture). Dr. Misener leads a team of research engineers in developing the Optimization & Machine Learning Toolkit (OMLT), which won the 2022 COIN-OR Cup as the best contribution to open-source operations research software development. She is associate editor for INFORMS Journal on Computing and Operations Research and is also a NeurIPS Area Chair.
"Autonomous Research Machines: Self-optimising New Chemistry"
Abstract:
Consider "micro reactor flow systems". Because these high-throughput microfluidic devices can control reaction conditions online, they are ideal for quantitatively characterizing diverse chemical synthesis techniques along new reaction pathways. The challenge is: How do we automate the design of experiments to "self-optimize" new chemistry? Together with the BASF Data Science for Materials & Chemistry teams, we’re interested to solve Bayesian optimization challenges which may simultaneously exhibit multiple objectives, mixed-feature spaces, asynchronous decisions, large batch sizes, input constraints, multi-fidelity observations, hierarchical choices, and costs associated with switching between experimental points. This presentation discusses the machine learning contributions that our team has found useful towards achieving these goals. I will also present our methodological and software contributions.