Susan Hunter earns DEPSCoR Award
Author: | Chloee Robison |
---|
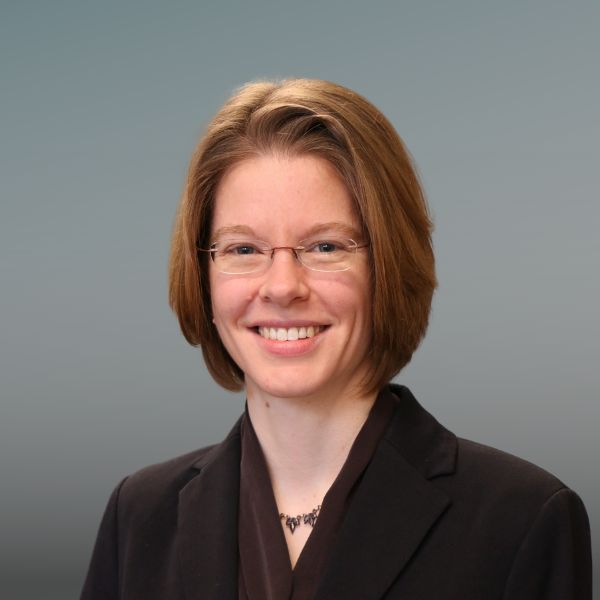
One of the projects was led by Susan Hunter, associate professor in the School of Industrial Engineering. Over the next three years, Hunter’s project will receive its share of $18 million in funding awarded by the program to 25 teams across 15 states.
Hunter’s project, in collaboration with Margaret M. Wiecek, professor of mathematical and statistical sciences at Clemson University, is titled “Theory and Algorithms for Two-Stage Decision Making Under Conflict and Uncertainty.” In the project, “Mathematical models and algorithms are developed to support decision making in the allocation and use of Air Force and Naval resources in circumstances involving two-stage decision processes, conflict, and uncertainty.” Decision-making problems are modeled as Two-Stage deterministic and stochastic Multiobjective Linear Programs (TSMOLPs), which can be solved to determine Pareto sets of outcomes for the first-stage decision variables. This research will aid the Air Force and Navy decision makers in determining optimal use of scarce resources under strict time constraints, conflict, and uncertainty.
Hunter and Wiecek’s project was chosen from among 115 white paper submissions for the award. Of the sixteen universities recognized, Purdue achieved the greatest number of grants.
Congratulations to Hunter and her team for the exciting accomplishment! You can read the full project abstract below.
The proposed research effort falls into the Mathematical Optimization Program and addresses the process of decision making during the planning and execution of Air Force and Naval operations. Mathematical models and algorithms are developed to support decision making in the allocation and use of Air Force and Naval resources in circumstances involving two-stage decision processes, conflict, and uncertainty. Two-stage decision processes arise because military operations often require strategic decisions under incomplete information, such as pre-positioning of materiel for a military campaign, followed by tactical operations decisions after the uncertainty is revealed, such as moving the materiel to subsequent locations of demand. Conflict originates from competing interests and goals, the presence of multiple decision makers, and multiple ways to assess system performance, including cost, safety, reliability, or chance of mission success. Uncertainty results from inaccurate or unknown data obtained from imperfect models or measurements, lack of knowledge, or the volatility of the global environment. Modeling these aspects of the military decision-making process leads to two-stage multiobjective optimization under uncertainty, an area of mathematical optimization that contains fundamental open questions and is responsive to the demand that computational sciences solve increasingly complex problems.
The PIs model the decision-making problems as Two-Stage deterministic and stochastic Multiobjective Linear Programs (TSMOLPs). The goal of solving a TSMOLP is to identify the feasible first-stage decision variables that produce the globally Pareto set of outcomes; this set of decision variables is called the globally efficient set. The PIs analyze foundational TSMOLP model classes to determine and prove structural properties of the efficient and Pareto sets that can be exploited by algorithms exactly or by approximation. The anticipated outcomes include mathematical characterization or approximation of the solution sets to TSMOLPs and a toolbox of provably convergent and efficient algorithms for solving them under a variety of modeling assumptions. The ability to assess the tradeoffs of stochastic solutions and quantification of the uncertainty creates significant added value.
The project makes a pioneering effort on this subject and creates a solid foundation for solving more complex two-stage multiobjective problems. The multiobjective paradigm, having the same rigor as the single objective setting but requiring more algorithmic and computational power, offers a broader perspective. This more inclusive viewpoint allows decision-makers to choose among the Pareto alternatives, that is, to use their judgment on top of mathematical results. The ability to solve TSMOLPs and their more complex variants with efficiency and optimality guarantees will assist the Air Force and Navy decision makers in making timely decisions about optimal uses of scarce resources under conflict and uncertainty. The broader impacts of this work will ultimately stem from the ability to solve intricate problems that become a norm in our global environment.