Advancements in embodied language models like PALM-E and RT-2 have significantly enhanced language-conditioned robotic manipulation. However, these advances remain predominantly focused on vision and language, often overlooking the pivotal role of tactile feedback which is advantageous in contact-rich interactions. Our research introduces a novel approach that synergizes tactile information with vision and language. We present the Multi-Modal Wand (MMWand) dataset enriched with linguistic descriptions and tactile data. By integrating tactile feedback, we aim to bridge the divide between human linguistic understanding and robotic sensory interpretation. Our multi-modal representation model is trained on these datasets by employing the multi-modal embedding alignment principle from ImageBind which has shown promising results, emphasizing the potential of tactile data in robotic applications. The validation of our approach in downstream robotics tasks, such as texture-based object classification, cross-modality retrieval, and the dense reward function for visuomotor control, attests to its effectiveness. Our contributions underscore the importance of tactile feedback in multi-modal robotic learning and its potential to enhance robotic tasks. The MMWand dataset is publicly available at https://hyung-gun.me/mmwand/.
Multi-Modal Representation Learning with Tactile Data
Authors: Hyung-Gun Chi, Jose Barreiros, Jean Mercat, Karthik Ramani, Thomas Kollar
In 2024 IEEE/RSJ International Conference on Intelligent Robots and Systems (IROS)
https://doi.org/10.1109/IROS58592.2024.10802699
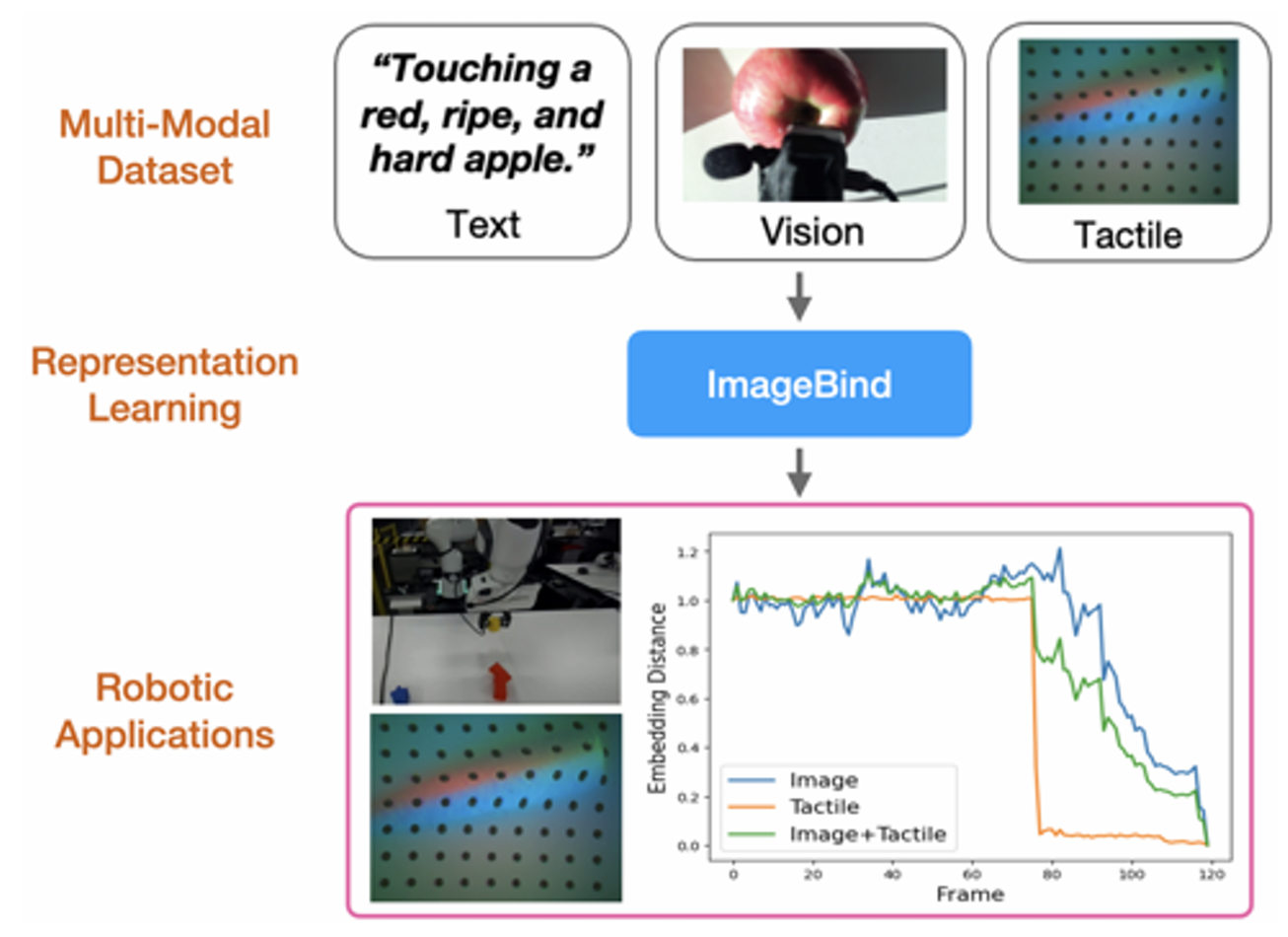

Hyung-gun Chi
Hyung-gun Chi is a PHD student in Electrical and Computer Engineering at Purdue University. Before he joining the C-Design Lab, He received his B.S degree from the school of Mechanical Engineering at Yonsei University, South Korea in 2017. His research interests are computer vision, and machine learning.