LiDAR revs up traffic safety studies
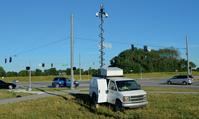
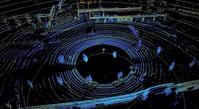
In an era when data gathering methods continually evolve to yield faster and more accurate results, traffic safety analysis remains a laborious and reactive process. However, research currently taking place at the Purdue Lyles School of Civil Engineering aims not only to improve the speed and quality of safety studies, but make them more predictive as well.
“Often with intersections, you’ll have a lot of ‘near misses’ but not many actual crashes,” says Andrew Tarko, professor of civil engineering and director of the Center for Road Safety. “There really hasn’t been a way to track or study these incidents, and so we will sometimes have to wait years to collect enough data from crashes to determine if there is actually a problem at an intersection.”
Through his research, however, Tarko has found a way to solve this issue using LiDAR (Light Detection and Ranging) technology. LiDAR is a surveying system that measures distance by illuminating a target with a laser light.
Mapping companies use LiDAR to create 3-D images of roads and cities, but Tarko’s team is able to digitally remove the backgrounds entirely from 3-D images, retaining only the information created by moving objects, such as vehicles and pedestrians.
Although the raw data is not easy to decipher, the schools of Civil Engineering and Mechanical Engineering came together to transfer it into usable information. With software created by mechanical engineering graduate student Vamsi Krishna, the countless dots on a black screen can be combined with an overview map.
The result shows vehicles and pedestrians — fully mapped — with data points, detailing speeds of the objects, their exact proximities to each other, at any given time and in any conditions.
From the data collected, and in a short time period, new safety measures — such as repositioning pavement markings, adjusting traffic signal timing, correcting geometry, or enhancing enforcement — can be implemented before a real crash ever takes place.
Tarko’s team successfully completed Phase 1 of the project in August as a proof of concept. It was jointly funded by the Federal Highway Administration and the Indiana Department of Transportation (INDOT) via NEXTRANS and the Joint Transportation Research Program (JTRP) for $450,000.
Phase 2, expected to run from August 2016 to August 2018, is funded through JTRP for $420,000 and includes two sub-periods. In the first year, a prototype trailer-based TScan unit will be built for INDOT field-test use across the state over the second year. Phase 3, funded at $270,000, may follow up with a second INDOT TScan unit for integrated use with the prototype to cover large areas.
Brad Steckler, director of traffic engineering for INDOT, says he is optimistic about the system’s potential to generate immediate, practical value to reduce risk of severe crashes at intersections and other highway locations such as horizontal curves.
“So far, it’s been wonderfully promising,” he says. “The notion of observing close calls has been around for a while, but it’s always been a manual and time-consuming task. This automated method removes that obstacle.”