Building a Better Future
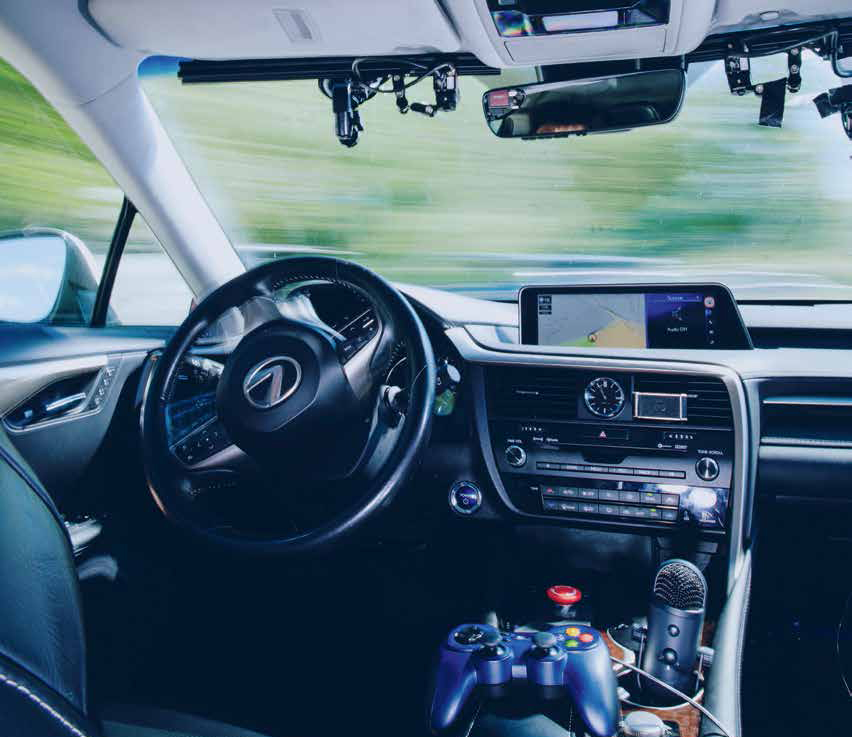
Using ChatGPT, this autonomous vehicle learns passenger preferences via verbal commands and makes performance decisions accordingly.
Five ways faculty are harnessing the power of artificial intelligence
We’re not living in the age of “The Jetsons” just yet, but by the time we reach 2062 — the futuristic year in which the cartoon sitcom was set — we might be.
As they have for millennia, civil and construction engineers will play a key role in shaping the conception and design of infrastructures and systems to sustain our civilization. The integration of artificial intelligence is already impacting the industry by powering solutions that enhance efficiency, safety and sustainability.
At Purdue, faculty in the Lyles School of Civil and Construction Engineering are applying machine learning, data mining and pattern and image recognition to solve problems and improve the needs of society.
Read on to learn how some of our brightest minds are harnessing the power of AI technology to build a better future.
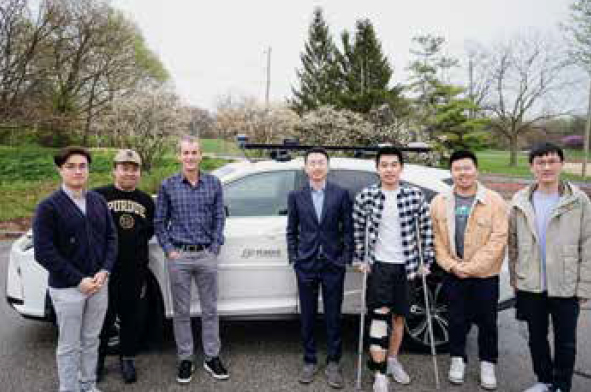
Ziran Wang, assistant professor of civil and construction engineering (center), with his research team and their autonomous vehicle that processes commands using ChatGPT.
A car that can practically read your mind
Imagine climbing into your autonomous vehicle, muttering that you should have woken up 20 minutes earlier. The car interprets this information as “I am running late” and therefore drives a bit more aggressively on the way to your destination.
Ziran Wang, an assistant professor of civil and construction engineering, and a team of researchers in the Purdue Digital Twin Lab are collaborating with Toyota Motor North America to develop a first-of-its-kind autonomous driving system that leverages large language models to understand the implicit and personalized needs of its passengers.
Using ChatGPT, the autonomous vehicle learns passenger preferences via verbal commands and makes performance decisions accordingly. Not only can the car tell when you’re in a hurry, it also ascertains your referred driving style, be it sporty or cautious. This is different from direct human input in today’s vehicles, where a driver can press a button to change from eco mode to sport mode. It’s using AI to interpret several preference categories to intuitively respond to a passenger’s wants and needs.
“If you’re riding along on the freeway and see another vehicle performing recklessly, stating ‘that car is crazy!’ will prompt ChatGPT to reason that you’d like the autonomous vehicle to change lanes to prevent a collision,” Ziran said.
It can help snag a better parking spot, too.
“The first time you drive to a stadium, the vehicle will navigate to the parking spot nearest the entrance,” Ziran said. “But next time, ChatGPT learns your preference is to leave a bit early so you don’t get stuck in parking lot traffic. The vehicle will adapt and choose a parking spot closer to the parking lot exit.”
Even though commonplace autonomous vehicles may still be a way off, Ziran said the ChatGPT model can be applied to existing advanced driver-assistance systems found in newer cars.
“Driver assistance systems such as adaptive cruise control can benefit from ChatGPT,” Ziran said. “Drivers may prefer larger or smaller gaps based on driving preference, current speed or road conditions, and knowing that information, the adaptive cruise control can respond accordingly. Large language models can help facilitate human-autonomy teaming and improve the system’s performance for the driver.”
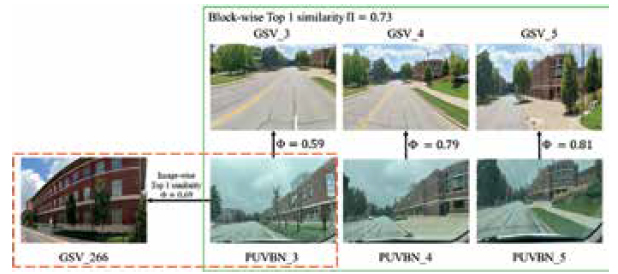
Jie Shan, professor of civil and construction engineering, led a team of researchers in developing a technology that mines photographic data from Google Street View to pinpoint a user’s location.
Technology that uses a photo to pinpoint your location
Poor cell service is a common issue in densely populated areas such as large cities. That can be problematic for tourists relying on their smartphone’s map application to navigate the streets. Instead of a precise location, the app might display a large circle encompassing several city blocks of the neighborhood. Imagine a vehicle equipped with sensors that snap photos of your surroundings to identify exactly where you are.
Jie Shan, professor of civil and construction engineering, and a team of researchers are developing a technology that mines photographic data from Google Street View to match images of a user’s surroundings. The technology operates independent of GPS signals to match the live photo to a database of images from Google Street View.
Not only can the technology identify your location, it also can determine your orientation based on which direction the sensor is pointing.
“Navigational positioning with emerging technologies such as autonomous driving is very important,” Shan said. “There is so much public information available, including Google Street View, aerospace images, digital terrain and city models. This technology leverages AI to track specific features on those images to identify a precise location.”
The researchers used AI technology to classify objects within live images, such as trees, roads, buildings and windows. They also applied the same algorithm to the database of Google Street View images. Photos were then matched to the image database using geometric and spectral characteristics to train the model to identify similar patterns. The researchers tested their method with images captured by both smartphones and drones. The technology developed by Shan and his team could one day be used to help automated drones navigate to their destinations.
“Civil and construction engineering isn’t only about building the infrastructure, it’s also about monitoring what’s been built and using those systems efficiently,” Shan said. “We must have precise measurements to assess the systems in place, and that’s where AI comes in. On the one hand, we sense the environment, but then we should be able to close the loop and use the data that’s been collected for the benefit of society.”
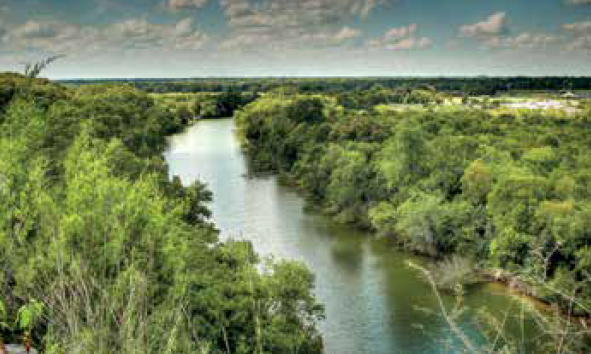
Using data gathered from other rivers, Venkatesh Merwade, professor of civil and construction engineering, developed a deep learning model to extrapolate the riverbed geometry of unmapped sections of the Brazos River.
A better way to map waterways in 3D
Flood events rose nearly 29% in the United States in 2023, leading to $7 billion in estimated damages according to the National Centers for Environmental Information. Understanding the riverbed geometry of specific waterways is crucial to developing water flow models and making recommendations for how to staunch potential floods.
Traditional methods of river mapping are time consuming and not feasible for large sections so data only exists for certain portions of many major rivers in the U.S. Venkatesh Merwade, professor of civil and construction engineering, and a team of researchers have developed a deep learning model that can extrapolate the riverbed geometry of unmapped sections based on the detailed geometry data available from other sections combined with aerial maps.
“We, and many others, have attempted to fill in these gaps using conceptual methods and physics-based methods, but our deep learning model provides results that are more accurate than what has been estimated with other methods,” Merwade said. “The eventual goal is to develop a model that can be applied to any river where data does not yet exist.”
The model has been implemented and tested for some areas of the Mississippi River as well as the Brazos River in Texas. Accurately mapping a river’s three-dimensional geometry by measuring its width, centerline, curvature, slope and other properties allows scientists to conduct detailed studies about flooding or contaminant transport.
PhD candidate Chung-Yuan Liang contributed to the research, some of which is funded by the National Science Foundation. In collaboration with the University of Iowa, Merwade developed RIMORPHIS, a publicly available research portal for river morphology studies. For future projects, Merwade plans to leverage the resources available in the Rosen Center for Advanced Computing at Purdue.
“The traditional methods of mapping a few kilometers of a river haven’t changed in decades,” Merwade said. “It’s as challenging and expensive as it was 20 years ago. We need to develop ways of making this data more readily available so we can better understand our rivers and make more accurate flood predictions. Using tools based on advanced statistical analysis to obtain accurate river morphology information is very exciting.”
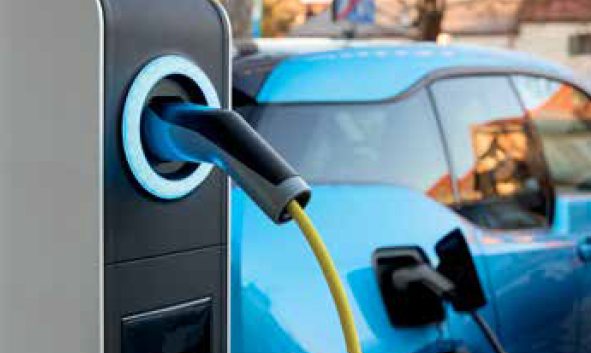
A research team led by Satish Ukkusuri, the Reilly Professor of Civil Engineering, developed AI toolkits to conduct predictive analytics to determine EV demand and travel patterns to inform charging infrastructure needs.
Predicting the need for EV infrastructure
The demand for electric vehicles in the United States is growing. In 2024, it is estimated that one in nine cars sold in the U.S. will be electric.
While electric vehicles boast many attributes, including savings on fuel costs, energy efficiency, lower pollution and cheaper maintenance, there are still some disadvantages to owning an electric car. One of the primary drawbacks? The limited availability of charging infrastructure makes it challenging to recharge an electric car while on the go.
“It’s a bit of the chicken and the egg problem,” said Satish Ukkusuri, the Reilly Professor of Civil Engineering. “There is a growing demand for electric vehicles, but it is important to understand where the demand is coming from so charging infrastructure can be built in the correct locations. If we only accommodate electric vehicles in urban environments, there would be little incentive for rural residents to buy electric vehicles because they don’t have easy access to charging stations.”
Using anonymized high resolution cellphone data, Ukkusuri and his research team developed a machine learning model to identify electric vehicles by examining underlying driving characteristics such as a vehicle’s acceleration and speed as well as stops at charging stations. The team then analyzes traffic patterns to identify where electric vehicle users travel, what activities they participate in during the day and how frequently they visit charging stations.
The research team, which includes PhD students Xiaowei Chen, Omar Hamim and Zengxiang Lei, has developed AI toolkits to conduct predictive analytics to determine EV demand and travel patterns and compare that data with existing charging infrastructure needs. Using this information, the team can make recommendations to the Indiana Department of Transportation, which is funding the research, about EV travel corridors, where charging stations may be needed in the future and inform EV equity planning to fill gaps in the existing infrastructure.
“There’s an expected shift in purchasing behavior of electric vehicles in Indiana and throughout the Midwest,” Ukkusuri said. “We want to be a catalyst for this change by identifying the infrastructure planning needed to support electric vehicles and make it convenient for people to make the EV transition using AI based data driven decision support.”
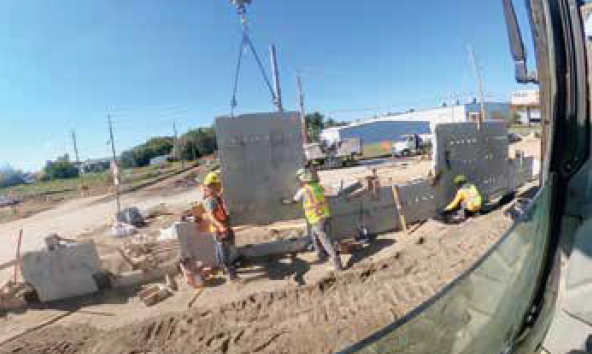
Software developed by Mohammad Jahanshahi, associate professor of civil and construction engineering, can identify specific frames of time-lapse video where certain activities are being conducted at a construction site.
Quality control for road construction
Roads are a critical part of the nation’s transportation system. When new roads are built or old roads are repaired, the contractor is expected to follow specifications for construction outlined by local, state or federal governmental agencies.
However, sometimes corners are cut, and not always intentionally. Currently, transportation officials don’t have an efficient means of ensuring work is being completed according to specifications — and that’s a problem. It is imperative that these regulations are strictly followed to reduce the risk of accidents and make transportation smoother and more efficient.
Mohammad Jahanshahi, associate professor of civil and construction engineering, and PhD students Malleswari Kachireddy and Nikkhil Vijaya Sankar (BSECE ’21), have developed software that uses computer vision and advanced machine learning to search hours of time-lapse video and identify frames where specific work activities are being done. Transportation officials can then review the identified relevant frames, without scrubbing through weeks, or even months, of video. The technology was developed in partnership with the Indiana Department of Transportation, which provided funding for the project.
“Working with INDOT, we focused a specific type of construction — the building of mechanically stabilized earth walls,” Jahanshahi said. “If regulations are not followed, these structures can be deficient and lead to failure. Our tool allows the INDOT staff to perform quality control without being on site for the duration of the project.”
The software was trained to identify four activities in the MSE wall construction process: backfilling, compaction, strap installation and MSE panel installation. The software can be adapted in future applications to identify other activities specific to a project.
“Our system conducts a comprehensive analysis,” Jahanshahi said. “Not only can it identify equipment at a construction site, but it can also track those objects to determine how the work is being done. It can parse information from a complex environment where multiple activities may be completed simultaneously.
“The opportunities for application of AI are limitless. But the technology only enhances human decision-making. You cannot exclude the human from the loop.”