Research
Predicting cerebral aneurysm growth using hemodynaic metrics obtained from MRI flow measurements.
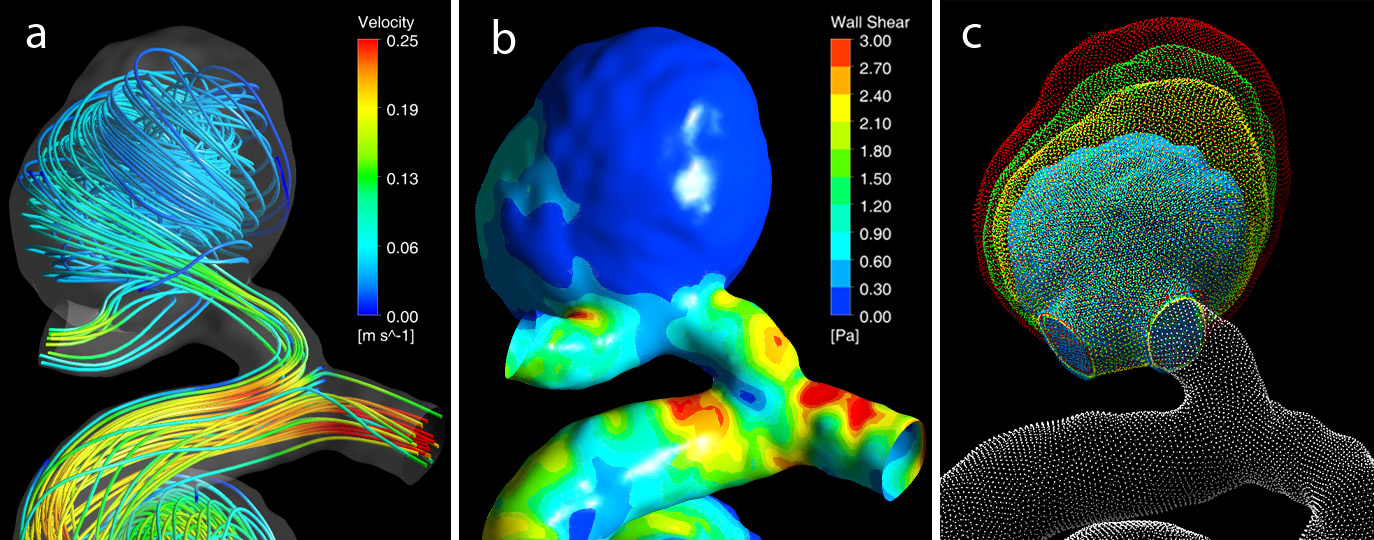
Multi-modality 4D Flow MRI data enhancement for quantification of cerebral aneurysms hemodynamics.
This project is performed in collaboration with a team at Northwestern University and is funded by the NIH R21 NS106696 grant. The ultimate goal of this research is to develop a data fusion approach for augmenting MRI measurements of cerebral blood flow. Local hemodynamic forces can affect growth and rupture of cerebral aneurysms, potentially resulting in brain compression or stroke. The flow in cerebral aneurysms can be measured with time-resolved, three-directional MRI (4D flow MRI); however, the accuracy of the obtained velocity fields may be inadequate for reliable estimation of the relevant flow metrics. Alternatively, the flow can be modeled with computational fluid dynamics (CFD) ot particle image velocimetry (PIV). The modeling approach provides superior resolution but relies on various simplifications and assumptions. We work on integrating in vivo MR velocimetry with patient-specific computational and experimental models in order to attain high-fidelity and high-accuracy velocity fields, thus allowing us to reliably compute relevant hemodynamic metrics.
Measuring cerebrospinal fluid flow to inform Alzheimer's Disease using magnetic resonance imaging enhanced by physics-constrained neural networks.
Recent advancements in Alzheimer’s disease (AD) research implicate cerebrospinal fluid (CSF) flow in the AD pathogenesis. Specifically, CSF flow is intricately linked to metabolic waste clearance in the brain. Obtaining reliable estimates of CSF flow from in vivo imaging data is a critical first step in understanding potential CSF-related mechanistic pathways for AD pathogenesis. However, the current imaging tools for measuring CSF flow are limited. Our study aims to develop a methodology for enhancing the accuracy of CSF flow measurement with MRI. We are developing physics-constrained neural networks for enhancing MRI velocimetry data in order to overcome the limitations of state-of-the-art flow quantification methods. This project will result in a clinical imaging tool for reliable quantification of CSF movement and improve our understanding of the flow dynamics in the ventricular system of the brain. In this study, a team of biomedical imaging and health science researchers at Purdue collaborates with a neurological disorders radiologist at the Indiana University School of Medicine.
Design and optimization of a chemofiltration device for removing chemotherapy toxins from blood.
Chemotherapy drugs injected intra-arterially to treat cancer can cause systemic toxic effects. A catheter-based Chemofilter device, temporarily deployed in a vein during the procedure can filter excessive drug from the blood thus reducing chemotherapy side-effects. This cross-disciplinary project, funded by the NIH R01 CA194533 grant, brings together clinicians, scientists and engineers from the University of California San Francisco, University of California – Berkeley, California Institute of Technology and Purdue, as well as industrial partners. The CFM lab’s objective is to optimize hemodynamic performance of the Chemofilter device. A multi-physics and multi-scale approach is used to simulate the transport and capture of the chemotherapy drugs. Developing computational models capable of coupling continuum mechanics with cellular interactions and intermolecular forces allows us to accurately predict filter efficiency and optimize its performance for specific agents and hemodynamic conditions. Alternative designs of the Chemofilter are evaluated in order to increase the contact area of the membrane, while minimizing its obstruction to the flow. The computational results are compared to in vitro experiments and animal studies conducted by our collaborators.