Labeled MOOCs (Massive Open Online Courses), this education format has revolutionized not just how courses are taught but also who can access them.
Purdue’s own investment in nanoHUB, a global resource for nanotechnology simulation, collaboration, and education, is based on such a promise.
But MOOCs are not without challenges. For one thing, they have been plagued by high dropout rates. Exactly who accesses these courses? Why do students lose interest? Can educators proactively act on data to nip problems before a student drops out? These are the questions that researchers at Purdue’s School of Engineering Education are looking to answer. And they are using smart data coupled with machine learning and a generous dose of ethical sensibilities to do so.
A Foundation of Smart Data
Krishna Madhavan, associate professor of engineering education, points out that the popularity of ed tech has led to a surge in data collection, but that in and of itself is not an asset.
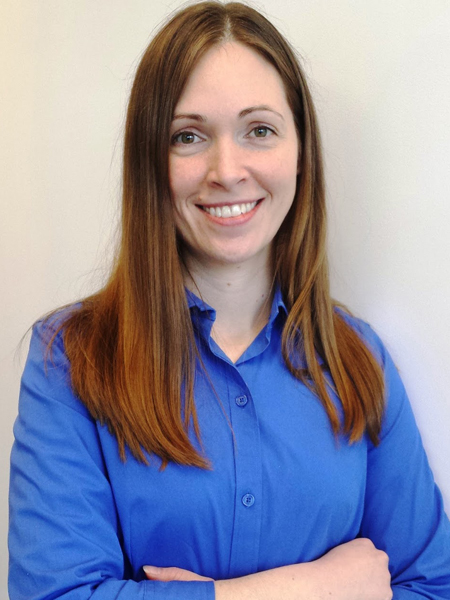

“The notion of ‘smart’ comes from actually telling which data are actionable assets and then following that up with actions that you derive from those insights. It’s not just about data or methodologies; it’s about how you can translate them into something meaningful for the users,” Madhavan says.
How does this notion of smart data translate to MOOC research? Madhavan points to the example of churn prediction: The data can yield early indicators about what might cause a student to drop out of a course. For instance, one of his researchers found that how a learner writes functions in MATLAB, an engineering software program, is an early predictor for later performance in class. In essence, learning how to write functions was a critical unit on which subsequent lessons were constructed. So if a student didn’t have that foundation right, the chances of failure or dropout were high.
“If you know when people are about to drop out of any learning model, online or offline, then you can intervene early. You can produce models that can then [proactively] predict when to intervene,” Madhavan says. An analysis of data for MOOCs has shown that the first few weeks are really critical for students to engage, he says, so instructors can proactively and more frequently communicate to check in on students during that period.
Building on Machine Learning
While it might be easy for a professor to predict student performance based on a set of indicators when class size is small, the task becomes particularly challenging when applied to MOOCs. “When you’re dealing with 50,000 students, you can’t know every person and the workload. It just doesn’t scale,” Madhavan points out.
This is where machine-learning models are useful in proactively predicting behavior and, by extension, making recommendations for intervention. The models are trained on smart data and can prove to be an important ally in increasing the efficiencies of the MOOC model. They can help automate workflows that can kick into action when somebody is about to drop out, for example. As more data points accumulate and you get more information, you have to keep refining your models to reflect that new intelligence, Madhavan says.
Eliminating Programming Bias
While machine-learning models can be extremely useful, Kerrie Douglas, assistant professor of engineering education, bases her research on the evaluation of these models to eliminate societal bias.
It is important, Douglas says, to figure out not just what evidence counts (think, smart data) but also what evidence is good enough. “We need to make sure that all learners are adequately represented in assessment models. We live in an educational environment that is run by data,” Douglas says. “Decisions and claims are made all the time based on data that is not always ethical. Just because a model found a relationship doesn’t mean that the inferences we can make from that are accurate and helpful,” she adds.
It is especially important that assessment models be bias-free in engineering education given the high stakes involved. “Assessments open and shut doors for learners,” she says.
When representation is key, what exactly do we mean by “all” learners? The diversity can be based on geography, ethnicity, race, socioeconomic status, and even learning styles, Douglas says. In a recent example, she found that non-native English speakers scored substantially lower on an assessment. “It was clear that the way the question was phrased was more challenging for the non-native speaker. It wasn’t that they had a different knowledge of what was to be measured; their level of English was the problem,” she says.
Douglas’s research informs the next iterations of MOOCs so assessment developers can work to collect the most useful and fair information. The professionals at nanoHUB, for example, use the research to inform future course offerings.
Together, Douglas and Madhavan work on complementary aspects of online education delivery. Douglas’s research evaluates how to construct the pedagogical framework needed to generate the right kind of data. Madhavan’s research focuses on, among other things, new approaches to working with data and translating that data into actionable insights.
Madhavan points out that the next step is to get educators to act on what the models are telling them. The notion of data fluency is very critical for success in education, including in MOOCs, he says. “Sometimes data might run counter to instincts. Then what do you do? This is definitely an opportunity for us to really think about how we use data and how we might influence [education delivery] decisions based on that. It’s a very different way of acting.”
Douglas, too, sees plenty of opportunity around the corner. “It’s an interesting time where engineers from around the world can learn together through online platforms, and there’s a huge amount of related data that’s being collected,” she says. “Advances in data science are generating the ability to ask new kinds of questions about how learning happens and how we can personalize that to meet more learner needs. After all, there’s no one-size-fits-all model in education.”