
More than half of the nation’s major power failures from 2000–2016 were caused by severe weather, affecting millions of people and costing billions of dollars, says Roshanak Nateghi, assistant professor of industrial engineering and environmental and ecological engineering.
“The number of billion-dollar climate disasters is expanding rapidly and so is the cost associated with them,” she says.
At the same time, vast quantities of data are available from numerous sources, and advances in machine learning are providing new modeling tools to improve resilience.
“Power outage risk is a function of various factors, such as the type of natural hazard; expanse of overhead transmission and distribution systems; the extent of rural versus urban areas; and the level of investment in operations and maintenance activities, including tree trimming and replacing old equipment,” says postdoctoral research associate Sayanti Mukherjee. “We are proposing a multi-hazard approach to characterize the key predictors of severe weather-induced sustained power outages. Our proposed framework can help regulatory commissions make risk-informed resilience investment decisions.”
The research group also has developed a “multi-dimensional infrastructure resilience” model geared toward hurricanes. “Despite the scientific consensus on the multivariate nature of resilience, the majority of existing approaches either focus on modeling a single dimension of resilience, or modeling its various dimensions separately,” Nateghi says.
For example, many approaches involve modeling a single performance measure, such as the number of protective devices activated during disaster impacts, the duration of loss of service, or the fraction of customers without power. However, the need for accurate and holistic disaster resilience modeling is reinforced by recent devastation from hurricanes Harvey, Irma, Jose and Maria, which have crippled communities in the United States and Caribbean Islands.
“These catastrophic storms call for a paradigm shift to a more holistic conceptualization of disaster resilience in order to foster improved adaptive capacity in the affected communities,” Nateghi says. “Resilience is much more than a single metric.”
The approach is said to be multivariate or multimetric because the models include many interwoven attributes of specific power grids and then determine the most important factors that predict the influence of storm impacts on the systems.
“We don’t just focus on making accurate predictions of impact,” she says. “We also care about identifying the most important predictors of damage and measuring their degree of influence so that an infrastructure operator can go back and make certain investments to minimize the impact of the next storm.”
Machine learning can be used in conjunction with more conventional modeling to add the ability to predict the impact of hurricanes on a system.
The model represents a new predictive tool to best prepare for and respond to future climate hazards. “Moreover, the model can be used to simulate ‘what-if’ scenarios to identify strategies for enhancing the resilience of the system,” Nateghi says.
The research was funded by the National Science Foundation and supported by Purdue’s Center for Climate Change, and the Energy Center. Doctoral student Debora Maia Silva provided data visualization.
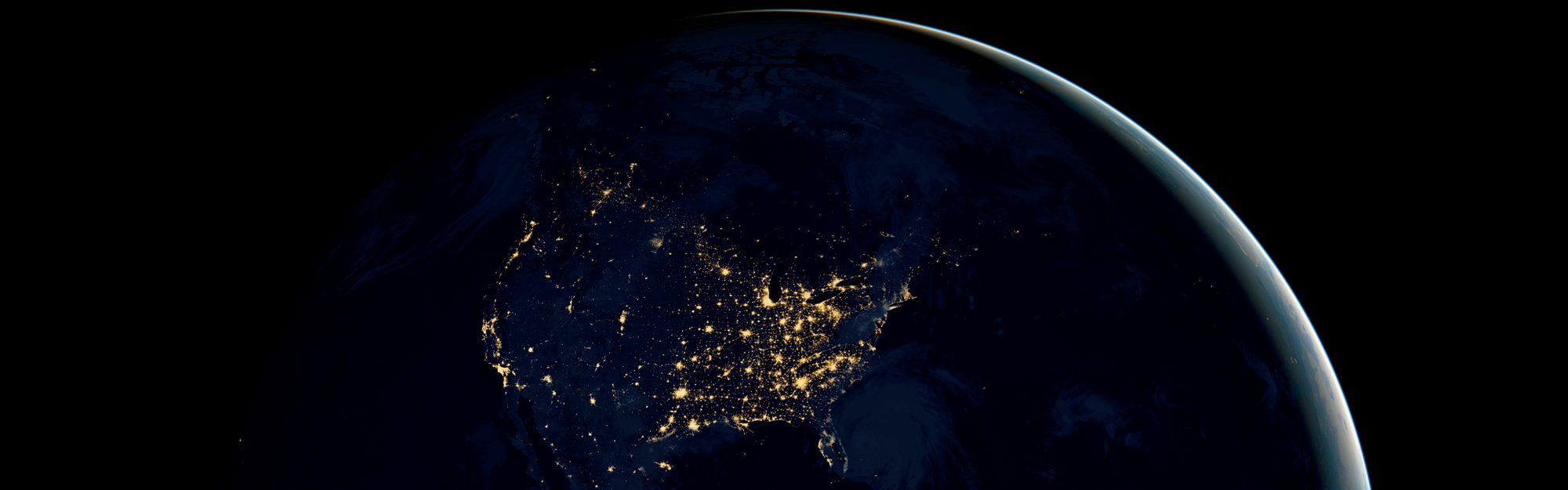
Tsunami Protection
Nateghi also has led research using an “ensemble-of-trees data-miner” to analyze the history of tsunamis along the Pacific coast of Japan’s Tohoku region. The modeling technique harnesses numerous “decision trees” to capture complex non-linear relationships of data.
Findings showed seawalls higher than 5 meters reduce damage and death, while coastal forests also play an important role in protecting the public. Seawalls more than 5 meters high were shown to reduce the destruction rate, and, more specifically, a 10-meter increase in height was associated with about a 5-6 percent decrease in the destruction rate.
Working with colleagues at Tohoku University, Maryland Institute College of Art and the University of Michigan, she studied data from tsunamis in 1896, 1933, 1960, and 2011.
The Japanese have embarked on a 10-year reconstruction project costing about 31.5 trillion yen, or about $255 billion, which includes the construction of tsunami seawalls along Tohoku’s Pacific coast. Critics of the program have voiced skepticism about the effectiveness of seawalls.
However, findings detailed in a research paper published in the journal PLOS ONE may bolster support for seawalls and coastal forests.
The same analysis method also could be used to study tsunamis in other regions. “All the insights are conditioned on the type of infrastructure you have in place, the topography and intensity of the tsunami,” Nateghi said. “But the methodology is extendable. You could do similar analyses for other regions using this method.”
In addition to NSF funding, the work was supported by the Japan Society for the Promotion of Science.
Safeguarding Urban Reservoirs
Nateghi’s research also has harnessed the Random Forest algorithm to learn how to safeguard urban water supplies, which are critical to the growth and well-being of cities.
“These supplies can be vulnerable to hydrological extremes, such as droughts and floods, especially if they are the main source of water for the city,” says doctoral student Renee Obringer. “Maintaining these supplies and preparing for future conditions is a crucial task for water managers, but predicting hydrological extremes is a challenge.”
The researchers initially developed the models using Lake Sidney Lanier in Atlanta, Ga., as the test site. However, further analysis demonstrated that the model based on the Random Forest algorithm is transferable to other reservoirs, specifically Eagle Creek in Indianapolis, Ind., and Lake Travis in Austin, Texas.