
ONR: Threat and Situational Understanding with Networked-Online Machine Intelligence (TSUNOMI), $13M
The Threat and Situational Understanding with Networked-Online Machine Intelligence (TSUNOMI) project receives $13M in total with congressional aid to the team of researchers from Saab and Purdue ICON. The project will develop an explainable machine learning framework with multimodal automatic target recognition and sensor resource management for early warning and situational awareness from surface vessels equipped with an automated verification and validation machine learning pipeline, and seeks to formulate effective techniques and algorithms to blend information from multiple sensors, such as cameras and radar, that have been deployed in an area to accurately identify objects that might enter that area.

NASA University Leadership Initiative: Safe, Secured and Assured Autonomy, $8M
The team seeks to develop a novel integration of secure and safe autonomous systems used on unmanned Advanced Air Mobility (AAM) aircraft with the goal of advancing their technical readiness level and be ready for industry to consider using these technologies. The team intends to validate these systems with flight tests of multiple aircraft.
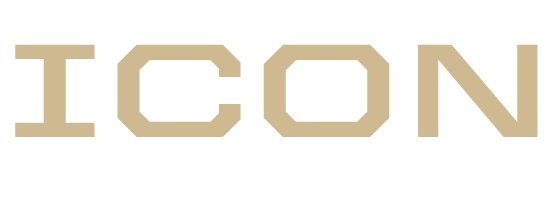
AI-assisted Multi-Agent Autonomy with Human in the Loop
This is a multi-university project funded by Northrop Grumman Corporation as part of the Real Application of Learning Machine consortium. This project aims to develop AI-assisted dynamic adaptive planning for multi-agent systems with human-in-the-loop shared autonomy. Methodologies come from a combination of control, optimization, learning and robotics by collaborations among faculties at multiple universities. The project develops techniques along with implementations for multi-agent platforms, which provide environment perception and situation awareness; perform real-time, dynamic and distributed solutions to control of assets, decision making and task assignment in changing environments; integrate human inputs/corrections to achieve mixed-human-machine autonomy; and utilize machine learning methods to continuously improve performance.
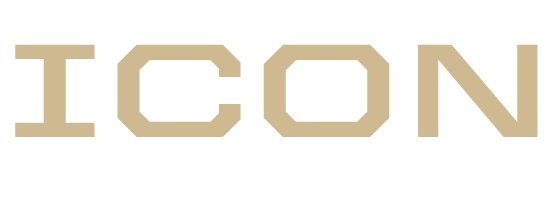
Cognitive Autonomy for Human CPS: Turning Novices into Experts
Human interaction with autonomous cyber-physical systems is becoming ubiquitous in consumer products, transportation systems, manufacturing, and many other domains. This project seeks constructive methods to answer the question: How can we design cyber-physical systems to be responsive and personalized, yet also provide high-confidence assurances of reliability? Cyber-physical systems that adapt to the human, and account for the human's ongoing adaptation to the system, could have enormous impact in everyday life as well as in specialized domains (biomedical devices and systems, transportation systems, manufacturing, military applications), by significantly reducing training time, increasing the breadth of the human's experiences with the system prior to operation in a safety-critical environment, improving safety, and improving both human and system performance. Architectures that support dynamic interactions, enabled by advances in computation, communication, and control, can leverage strengths of the human and the automation to achieve new levels of performance and safety. This research investigates a human-centric architecture for "cognitive autonomy" that couples human psychophysiological and behavioral measures with objective measures of performance.
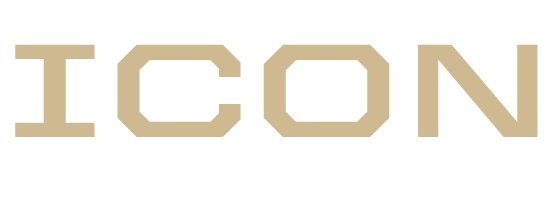
Project drawing on recovery lessons from Hurricane Sandy aims to improve U.S. resilience and disaster preparedness
Understanding the recovery of communities after disruptions has important implications for efficiently allocating resources, better planning for disasters, and reducing time and cost of recovery. Virtually all communities are embedded in highly interdependent social and physical infrastructure. This coupling between social and physical networks can lead to complex cascading effects that cannot be understood by looking at these networks in isolation. The full implications of these interdependencies for the resilience of communities and their ability to recover after disasters are not currently understood. This research seeks an understanding of the underlying factors that lead to resilience and recovery of interdependent social and physical networks after disasters. The researchers will collect data from communities impacted by Hurricane Sandy to create and test modeling approaches for improved knowledge of both social and physical factors that lead to recovery. It will also lead to a better understanding of the interdependencies between the social and physical systems, and will identify potential tipping points where small changes in the social and physical systems significantly impact the recovery of the overall system. The findings from the study will allow governmental and emergency agencies to take actions that will accelerate system recovery and enhance its resilience.