An Assessment Tool For The Air Compressor System
An Assessment Tool For The Air Compressor System
Anomaly detection
Primary researcher: Da-Chun (Allen) Wu, Ph.D. candidate
This research integrates machine learning with the IIOT platform to improve energy efficiency in industrial settings by detecting unwanted air demand in compressed-air systems, shown in Fig.1. Utilizing an unsupervised machine learning model, the project distinguishes between operating and non-operating hours, allowing for the identification of abnormal signals during inactive periods and triggering necessary maintenance alerts. Employing techniques like k-means clustering, the model analyzes data from sensors embedded within the systems that monitor compressor energy consumption and air usage metrics such as pressure and airflow. This data is transmitted in real-time to an edge computer, where the model learns normal operational patterns and quickly identifies deviations during downtime. These detections prompt alerts to facility managers, facilitating rapid interventions to fix potential leaks, thus preventing unnecessary energy usage and reducing maintenance costs.
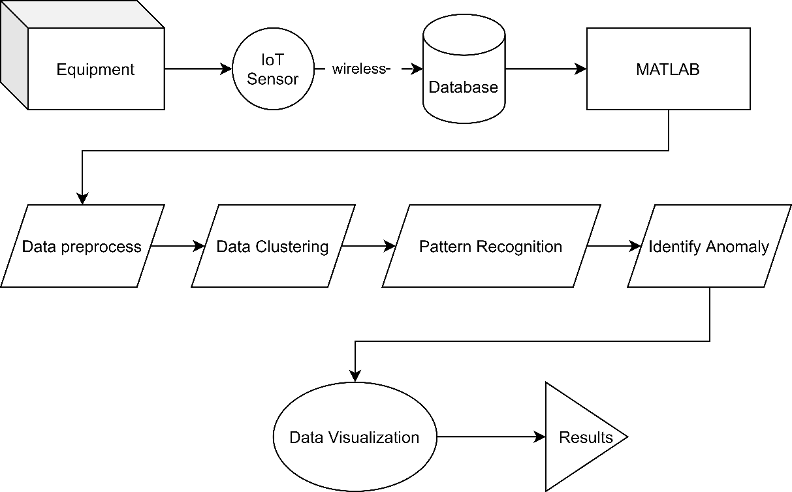
Compressor pressure setting optimization
Primary researcher: Da-Chun (Allen) Wu, Ph.D. candidate
This research project uses the IIOT platform to enable air compressor users to identify potential energy savings by adjusting compressor pressure settings. Utilizing sensors on the demand side that monitor pressure and airflow, an algorithm was developed based on Long Short-Term Memory (LSTM) networks to explore the correlation between compressor pressure settings and energy consumption. This algorithm accounts for constraints on the demand side and determines an optimal pressure setting, which can lead to significant energy savings compared to the baseline. This AI-driven approach not only optimizes operational efficiency but also contributes to substantial energy conservation in industrial applications.
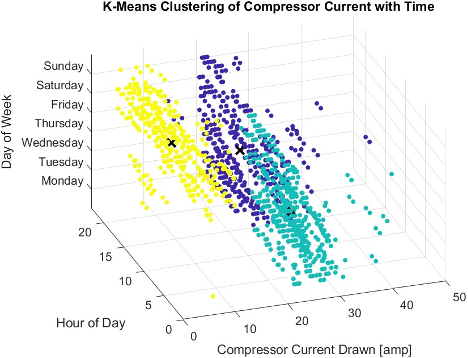
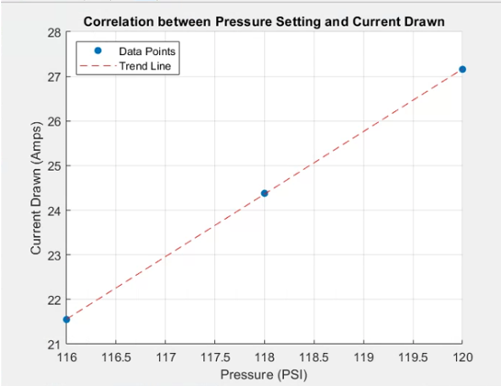