Student Spotlight
Amitangshu Mukherjee
A day when machines can perceive, reason, and interact like humans may not be far off—and Amitangshu Mukherjee is helping bring that future closer.
Amitangshu began his academic journey at Heritage Institute of Technology in his hometown, later earning his MS from Iowa State University. In 2020, he joined Kaushik Roy's Nanoelectronics Research Laboratory (NRL) at Purdue University, where he now performs cutting-edge research in next-generation AI perception systems.
His focus? Developing deep learning-based active vision systems inspired by how humans see and understand the world. "I'm exploring how to help AI better recognize rare or uncommon objects and understand complex scenes," he explains. His work, published in Transactions on Machine Learning Research, dives into improving visual robustness—ensuring AI performs better on difficult or unfamiliar samples.
"Human-inspired vision offers powerful ways to tackle the blind spots in today's AI systems," he says. Amitangshu is especially interested in models that integrate both vision and language to build smarter, more adaptive AI. The goal is to create systems that can safely and reliably interact with people and environments—think humanoid robots or AR/VR tools that actually 'get' the world around them.
"Deep learning still struggles with rare or unexpected inputs," he notes. "Improving this is crucial for autonomous systems, where safety and consistency matter most." By incorporating active learning and human-like inference strategies, his research aims to strengthen AI's ability to reason about structure and context—just like we do.
Looking ahead, Amitangshu is committed to designing AI that's not only robust and intelligent but also sustainable. "It's about building systems that work with humans—and for the world we live in."
He also offers simple yet powerful advice to fellow students: "Keep it simple and show up every day. Work hard, but don?t forget to rest."
Amitangshu joined NRL during the early uncertainty of the COVID era, but found a strong sense of belonging through his supportive lab mates. "They made everything easier—lots of laughs, collaboration, and once it was safe, some great hangouts too."
Some of his favorite PhD memories? Presenting at CVPR in New Orleans, hiking in Yellowstone, and spotting the legendary Wapiti Lake wolf pack. Closer to home, he finds peace in quiet walks through Happy Hollow and Celery Bog.
As Amitangshu continues to bridge human cognition with artificial intelligence, one thing is clear—he's not just shaping smarter machines, he's helping shape a more thoughtful future.

Jimmy Gammell
Jimmy Gammell is a PhD student whose research in deep learning is reshaping how we think about securing our digital lives. Jimmy's work at Kaushik Roy's Nanoelectronics Research Laboratory (NRL) at Purdue University is advancing the frontier of deep learning, with a specific focus on interpretability and generative modeling. His research, which began in 2021, is pushing the boundaries of how deep learning methods can be applied to side-channel leakage localization—a critical area in cryptographic security.
Before joining Purdue, Jimmy earned his degree in electrical and computer engineering, as well as applied math, from the University of Colorado Boulder. Today, his innovative deep learning techniques are helping identify hidden vulnerabilities in cryptographic algorithms, where information might unintentionally leak through unintended channels. This is crucial for protecting sensitive data and ensuring the security of communications. As Jimmy explains, "Traditional methods often overlook complicated or atypical statistical relationships that humans don't anticipate." His work addresses this gap, enabling more accurate detection of these leaks.
"This helps improve the security of data transmission and storage in applications like credit card transactions, internet use, and top secret government document protection," Jimmy explains. "It's exciting because we are building aspects of this technology from the ground up, rather than iterating on prior work. This requires fine-tuning both the theory and implementation to make it useful in the real world."
For Jimmy, it's not just about the big ideas. He believes that "being aware" of the fine details is the best lesson in both life and research. His advice to aspiring PhD students is to "Dive deep into the minute implementation details of state-of-the-art methods in your field. These are often more important than high-level conceptual ideas."
After completing his PhD, Jimmy plans to apply his deep learning expertise to the tech industry, where he hopes to do research in generative modeling and interpretability.
But it's not all research for Jimmy. Outside the lab, he's an avid outdoor enthusiast who finds solace in nature. One of the highlights of his PhD journey was a trip to Red River Gorge in Kentucky with his NRL lab mates. There, they tackled a thrilling via ferrata climb, blending teamwork and adventure.
With his passion for deep learning and dedication to cryptographic security, Jimmy Gammell is making an impact—and there's no doubt he will continue in the years to come.


Shristi Das Biswas
Innovation isn't just a goal—it's a way of life for Shristi Das Biswas. Her journey began at the Indian Institute of Engineering Science and Technology, where her passion for technology took root. Now, as a researcher at Purdue University's Nanoelectronics Research Laboratory (NRL) under Professor Kaushik Roy, she is redefining how we approach learning paradigms for generative models.
At the heart of her work is PIXELS, a groundbreaking framework that pushes the boundaries of image editing with diffusion models. In a world where everything is customizable, why not take it a step further—down to the pixel? PIXELS enables users to make fine-tuned, region-specific edits without retraining or fine-tuning existing text-to-image models. By leveraging progressive exemplar-driven editing, it allows users to draw inspiration from multiple reference images or multimodal prompts, seamlessly incorporating changes in real-time.
"Imagine what's possible with PIXELS!" Shristi shares enthusiastically. "The potential is endless. With greater control at the pixel level, we're not just generating images—we're crafting them with precision and intent." With its ability to selectively modify individual objects and introduce gradual spatial changes, PIXELS revolutionizes how we train and infer with large generative models—making them more efficient, adaptable, and less dependent on massive computational resources.
But beyond the technical breakthroughs, Shristi embodies a relentless drive to push boundaries. Her advice to aspiring innovators? "Find what inspires you—and don't let it go."

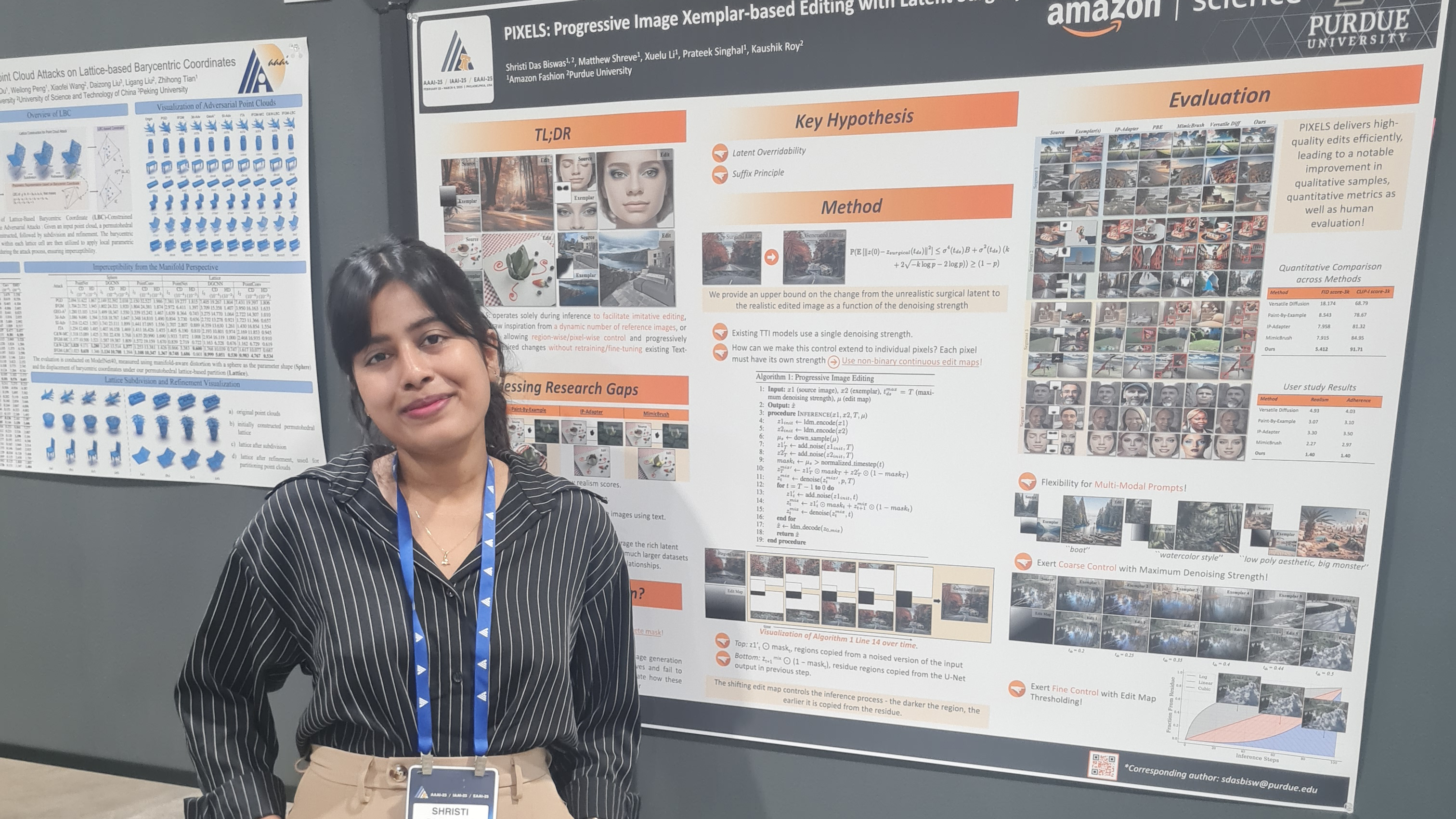
Kang He
The thrill of discovery began at the Harbin Institute of Technology, where a passion for AI and machine learning took root. Now a PhD researcher at Purdue University's Nanoelectronics Research Laboratory (NRL) under Professor Kaushik Roy, Kang He is dedicated to advancing large language model (LLM) reasoning, optimizing prompts, and tackling the complexities of few-shot learning.
After earning his MS from the University of Pennsylvania, Kang joined Purdue in 2020, eager to explore the fast-evolving world of AI. His current work focuses on enhancing reasoning tasks with LLMs, particularly addressing the challenges of inference-time scaling.
With the rapid advancements in LLMs, Kang finds himself in a dynamic field full of both challenges and opportunities. "The fast-paced nature of this work drives me to constantly refine my approach," he says. "It's both stimulating and impactful."
Kang's research improves logical coherence in AI-generated reasoning, outperforming traditional chain-of-thought (CoT) and tree-of-thought (ToT) methods. "LLMs still struggle with generating high-quality long reasoning without inconsistencies," he explains. His new framework enhances accuracy and interpretability, making AI more reliable for complex reasoning tasks.
Kang's journey has been shaped by his deep curiosity and drive to think beyond existing methods. His advice to future PhD students? "Stay curious! Always think deeply!" This mindset fuels his passion and will propel him toward his dream job as an applied ML researcher in industry.
One of Kang's most memorable experiences was attending the 2024 Conference on Empirical Methods in Natural Language Processing, where he presented his research and connected with fellow researchers. He enjoyed exchanging ideas on emerging trends and real-world applications. The experience inspired him to push his research further, reinforcing his commitment to making meaningful advancements in AI.
With a clear vision and innovative mindset, Kang He is shaping the future of AI reasoning—one breakthrough at a time.
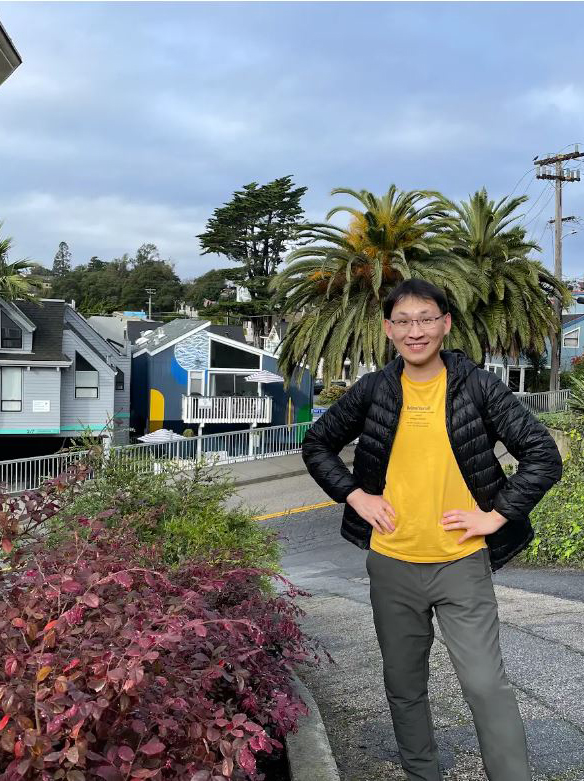
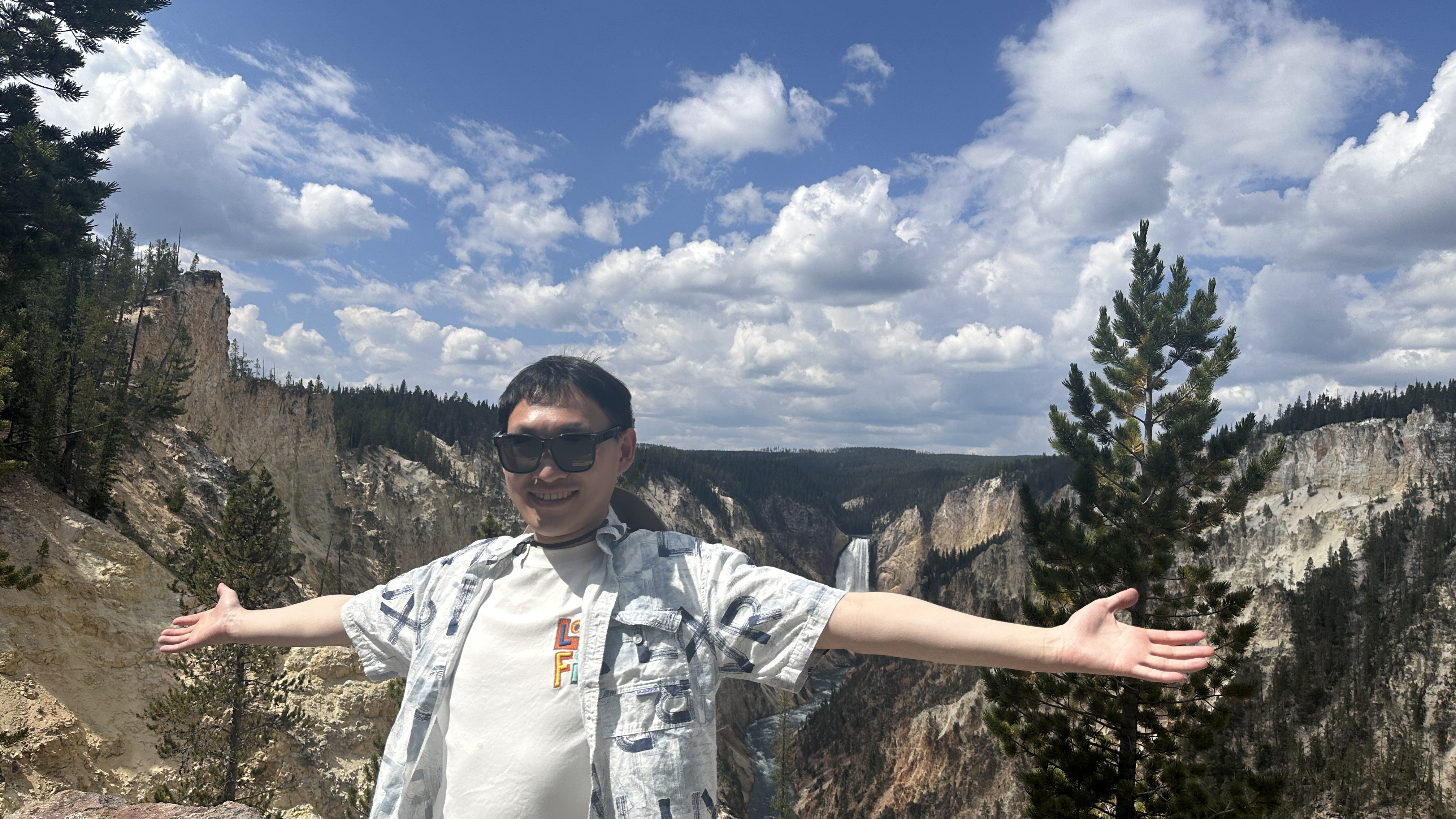
Eunseon Yu
The future of computing isn't just about speed—it's about efficiency, reliability, and innovation. This belief drives Eunseon Yu, a researcher in Kaushik Roy's Nanoelectronics Research Laboratory (NRL) at Purdue University, as she explores the world of CMOS and memory devices for in-memory computing. Her work focuses on developing next-generation solutions that will transform the way we process information.
Her journey began in South Korea, where she earned her BS and MS degrees from Gachon University before joining Purdue. Now, she is focusing on creating energy-efficient ferroelectric devices, an advancement that could revolutionize memory chips by making them faster, more reliable, and less power-hungry.
"I am developing and fabricating energy-efficient ferroelectric devices. But beyond that, I want to understand how these devices interact with their environment and how we can integrate them into new computing architectures to improve memory performance," Eunseon shares.
Her research goes beyond standard device characterization—she explores how these devices perform at the array level, an area that has often been overlooked. By optimizing the ferroelectric stack for low-voltage operation, she is helping to pave the way for more efficient artificial intelligence and in-memory computing solutions.
"Our work is cutting edge. We don't just prove feasibility—we demonstrate real performance. By assessing ferroelectric devices in crossbar arrays, we gain critical insights to improve their reliability and efficiency," she explains.
Eunseon thrives in an environment that pushes her to think beyond the obvious. She believes in the importance of being proactive and open-minded, embracing different perspectives, and always seeking to learn.
"I want to be in an environment that challenges me. I want to make a meaningful impact by developing energy-efficient computing solutions," she says.
But it's not just about research—Eunseon cherishes the moments of spontaneity that spark creativity. Some of her favorite memories from Purdue were the impromptu coffee-room conversations with her advisor and lab mates, where casual chats often led to groundbreaking ideas. She also enjoyed the diverse insights gained from Purdue ECE seminars—and when she needed a break, she could often be found on the tennis courts, recharging for her next big breakthrough.
With a passion for innovation and a drive to create meaningful change, Eunseon Yu is helping shape the future of energy-efficient computing—one device at a time.
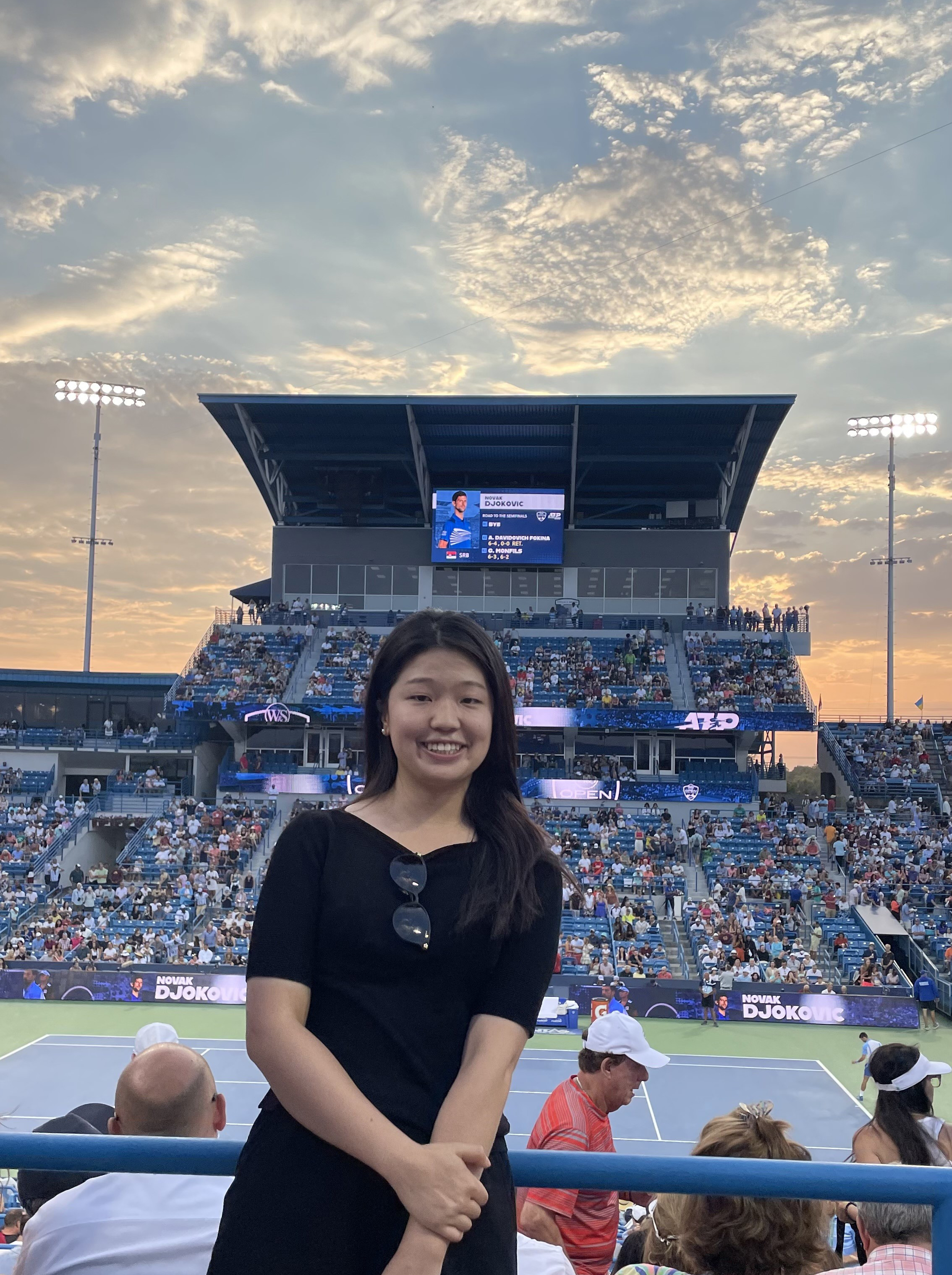
Marco Apolinario
For Marco Apolinario, the human brain is more than just an organ—it's a blueprint for the future of artificial intelligence (AI). From the National University of Engineering in Lima, Peru, Marco joined Kaushik Roy's Nanoelectronics Research Laboratory (NRL) at Purdue University to explore how AI can learn like the brain—efficiently, adaptively, and with minimal energy consumption.
"My current project focuses on co-designing learning rule algorithms and energy-efficient neural hardware accelerators capable of on-device learning," Marco explains. "This will make learning on edge AI systems faster, more secure, and significantly more energy-efficient."
The contrast between human intelligence and today's AI systems is staggering. While the brain operates on just 20 watts of power (the same as a lightbulb), modern AI can consume as much energy as an entire household over several years. Marco's research aims to bridge this gap by developing brain-inspired learning algorithms that enable AI systems to adapt and evolve in real-time, all under extremely low power consumption—just like neurons in the human brain.
Marco's fascination with neuroscience, AI, and hardware design led him to tackle one of AI's biggest challenges: energy consumption. By designing algorithms and hardware that enable on-device learning, his work has the potential to revolutionize AI applications in healthcare, robotics, and edge computing—bringing smarter, more sustainable AI to users everywhere.
"This is super exciting because AI will be capable of learning how to solve complex problems—recognizing patterns, making decisions, learning from experience—all without relying on massive power-hungry servers," Marco shares. His research could extend AI accessibility, reduce environmental impact, and unlock new possibilities for AI-powered devices in remote areas.
To future labmates and aspiring researchers, Marco offers this advice: "Take full advantage of the freedom to explore your ideas… and the many opportunities Purdue offers outside of the lab." He believes that both personal and professional growth are key to a fulfilling research journey.
His own time at Purdue's NRL has been filled with memorable experiences, from discovering new hobbies to forming lifelong friendships with researchers from around the world.
One of his standout moments? A three-week workshop on neuromorphic engineering in Telluride, Colorado—where cutting-edge research met the breathtaking backdrop of the Rocky Mountains. "The world-renowned researchers, the inspiring lectures, the hikes, and even the 4th of July parade—it was an unforgettable experience."
As Marco continues pushing the boundaries of energy-efficient AI, his vision remains clear: to design AI systems that are as intelligent and adaptable as the human brain—while consuming just a fraction of the power. His work at Purdue is paving the way for a smarter, more sustainable AI future.
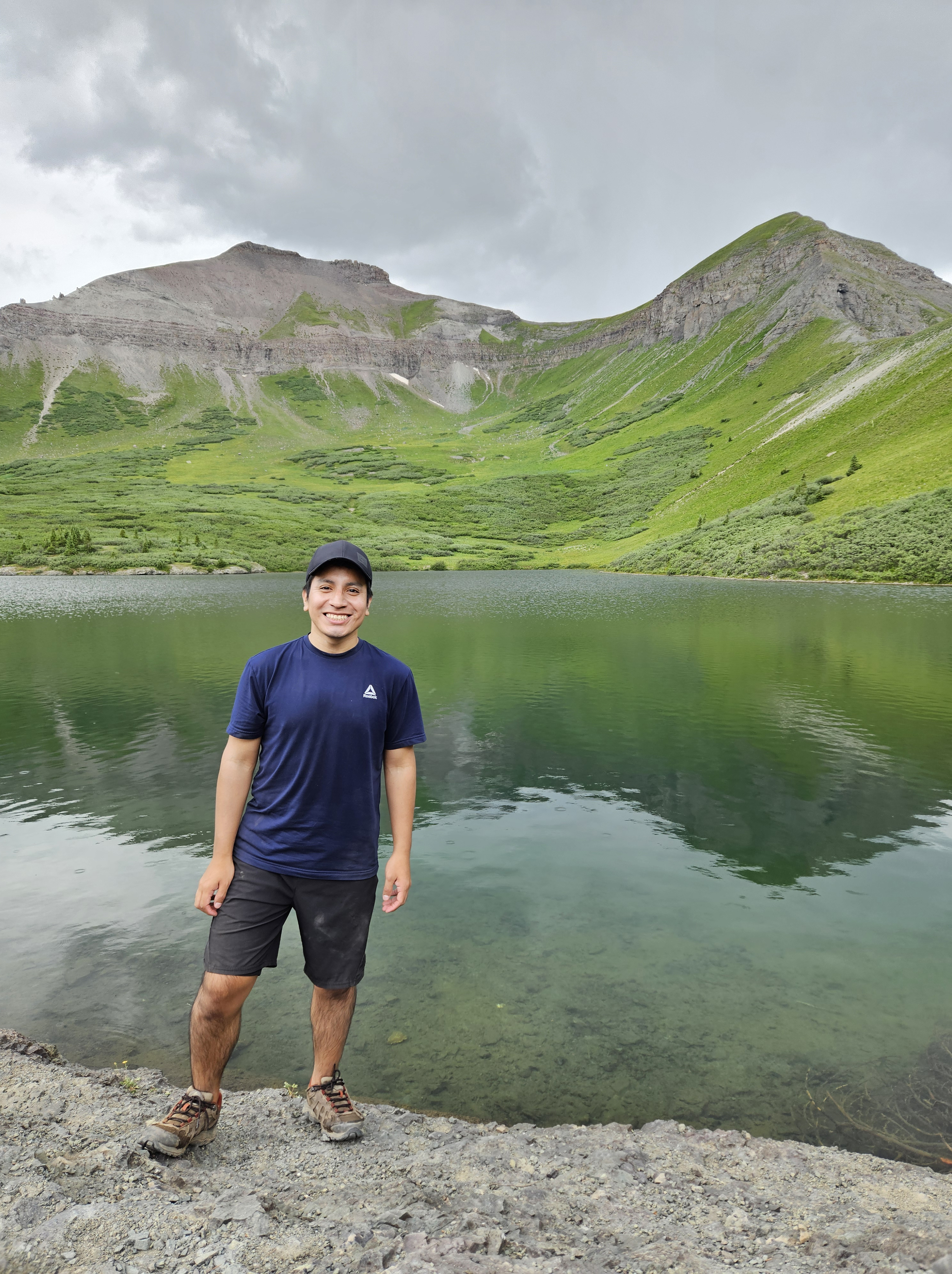
Adarsh Kosta
From the prestigious Indian Institute of Technology Kharagpur to Purdue University's Nanoelectronics Research Laboratory (NRL), Adarsh Kosta is making strides in the world of neuromorphic computing. Under the mentorship of Kaushik Roy, he is dedicated to developing efficient algorithms and neural network architectures that mimic the computational power of the human brain.
"My work is inspired by the computational capabilities and efficiency of the human brain, aiming to incorporate these principles into modern AI systems," Adarsh shares. His research addresses real-world challenges in computer vision, robotics, and artificial intelligence, making cutting-edge technology more effective and accessible.
Currently, Adarsh is pioneering a method to model and preserve temporal coherence in Video Diffusion models, a key challenge in video generation. "I am drawing inspiration from bio-inspired neurons to effectively capture temporal dynamics in my work," he explains. His approach aims to create visually appealing individual frames while ensuring smooth transitions between them, greatly benefiting applications in media, AR/VR, healthcare, and robotics.
One of Adarsh's primary interests lies in Spiking Neural Networks (SNNs), particularly for computer vision and robotics. Traditional Video Diffusion models often struggle with inconsistencies and high energy demands, leading to processing delays. By leveraging neuromorphic techniques, Adarsh is enhancing the efficiency and reliability of large-scale AI models. His work has the potential to revolutionize multiple industries, enabling more people with limited resources to experiment with advanced video generation tools.
Beyond his research, Adarsh finds endless inspiration in nature. "Biological organisms are designed to evolve and excel. I want to use this background to address real-world challenges and enhance the quality of life," he says. He encourages fellow PhD students to stay curious and persistent, offering a simple yet profound piece of advice: "The world is filled with problems big and small. Do not lose hope. If you feel lost, take a walk, observe the world. You will generate your next big idea."
Adarsh fully embraces PhD life at Purdue, from early morning yoga sessions to late-night food adventures, from intense research discussions to casual coffee chats. Whether navigating nerve-wracking team meetings or enjoying joyful barbecues with lab mates, he cherishes every moment at NRL. One of his most meaningful experiences was the overwhelming support he received from his lab mates following an unexpected accident and emergency surgery. Their kindness and encouragement reassured him that he had found a second family at Purdue.
With his passion for nature-inspired computing and a strong support system at Purdue, Adarsh Kosta is paving the way for groundbreaking innovations in AI and beyond. His journey is a testament to perseverance, collaboration, and the power of curiosity in driving technological advancement.
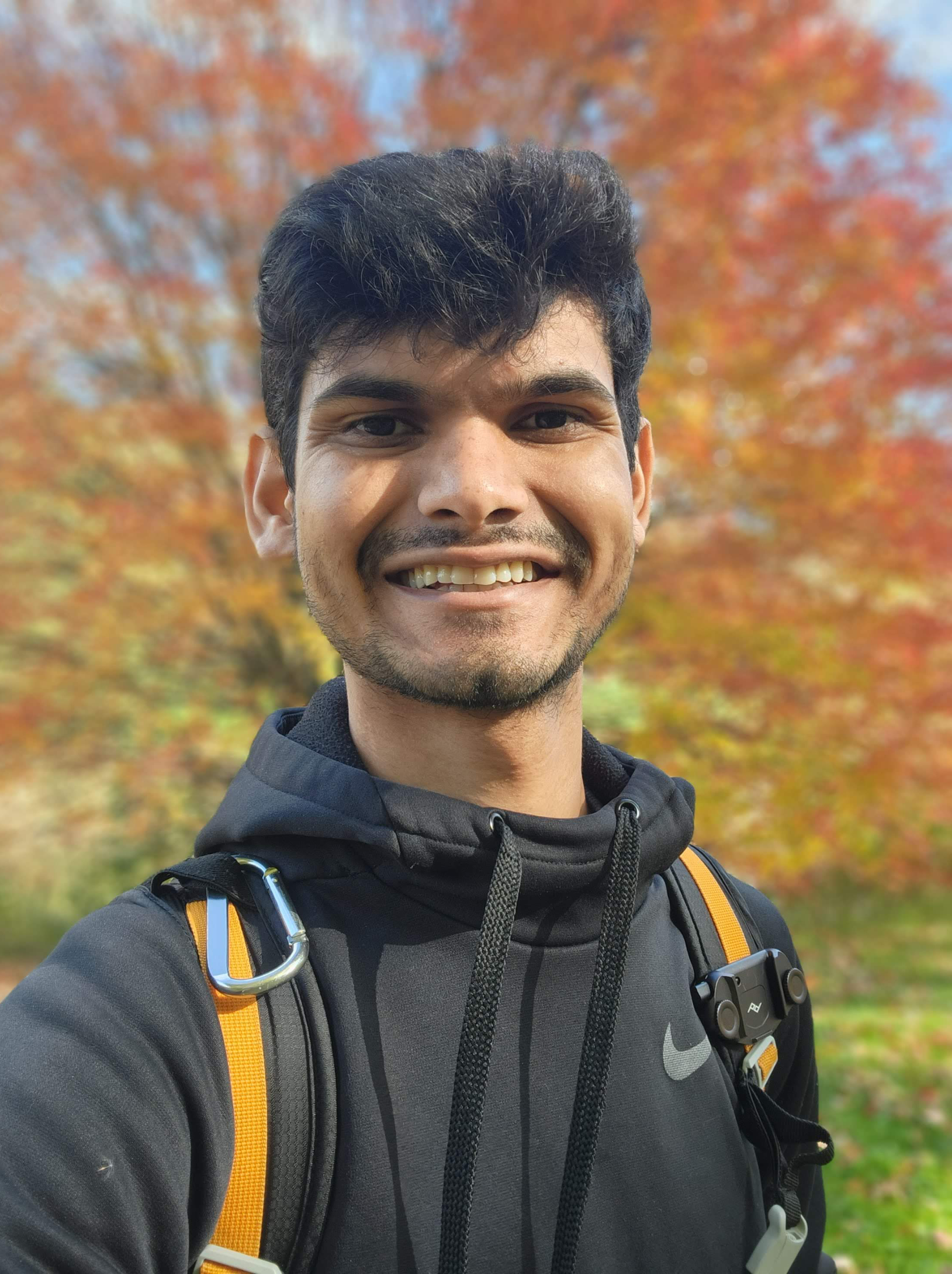
Shubham Negi
Shubham Negi, a dedicated researcher from the Indian Institute of Technology Kharagpur, joined the Nanoelectronics Research Laboratory (NRL) to explore hardware-software co-design for efficient machine learning under the guidance of Professor Kaushik Roy. His research aims to minimize bottlenecks in analog Compute-in-Memory (CiM) accelerators, striving for a more accurate and scalable approach to handling larger datasets.
In his most recent project, Shubham and his team proposed an innovative algorithm-hardware co-design approach. They introduced HCiM, an ADC-less Hybrid Analog-Digital CiM accelerator. HCiM leverages analog CiM crossbars for matrix-vector multiplication while utilizing a digital CiM array to process scale factors associated with partial sum quantization. Shubham explains, "This pioneering method offers a promising solution for low-power edge applications."
A key challenge in Shubham's research involves managing scale factors in hardware. While collaborating with a colleague focused on quantization-aware training methodologies, he realized the complexities of handling scale factors in analog CiM accelerators. "Managing scale factors in hardware is challenging," he notes. "Our hybrid approach takes advantage of both analog and digital domains, enhancing efficiency and accuracy." This work culminated in the paper, "HCiM: ADC-Less Hybrid Analog-Digital Compute in Memory Accelerator for Deep Learning Workloads," which he recently presented at the Asia and South Pacific Design Automation Conference.
Driven by a passion for impactful contributions to machine learning and computer architecture, Shubham aspires to bridge the gap between research and product development. "I want to turn new ideas into practical solutions," he shares enthusiastically.
Reflecting on his Ph.D. journey, Shubham acknowledges both challenges and rewards. "The early days of a Ph.D. are tough, but they teach you invaluable lessons about research and yourself," he says. He recommends Stephen R. Covey's "The 7 Habits of Highly Effective People" to new students and advocates daily meditation, staying active through sports or exercise, and engaging in discussions with peers to enhance understanding and research capabilities.
Shubham cherishes many memorable experiences, crediting insightful discussions with co-authors and NRL lab mates for his growth. "Presenting my work feels like promoting a movie — it's exhilarating!" Some of his notable presentations include DAC'22, CoCoSys, C-BRIC, SRC AIHW annual review, and IROS'24. His trip to IROS in Abu Dhabi stands out as a highlight, where he witnessed the real-time application of his group's research in robotics systems and experienced the vibrant culture of Abu Dhabi and Dubai.
Shubham Negi's journey embodies perseverance, collaboration, and a relentless pursuit of innovation in machine learning and computer architecture.
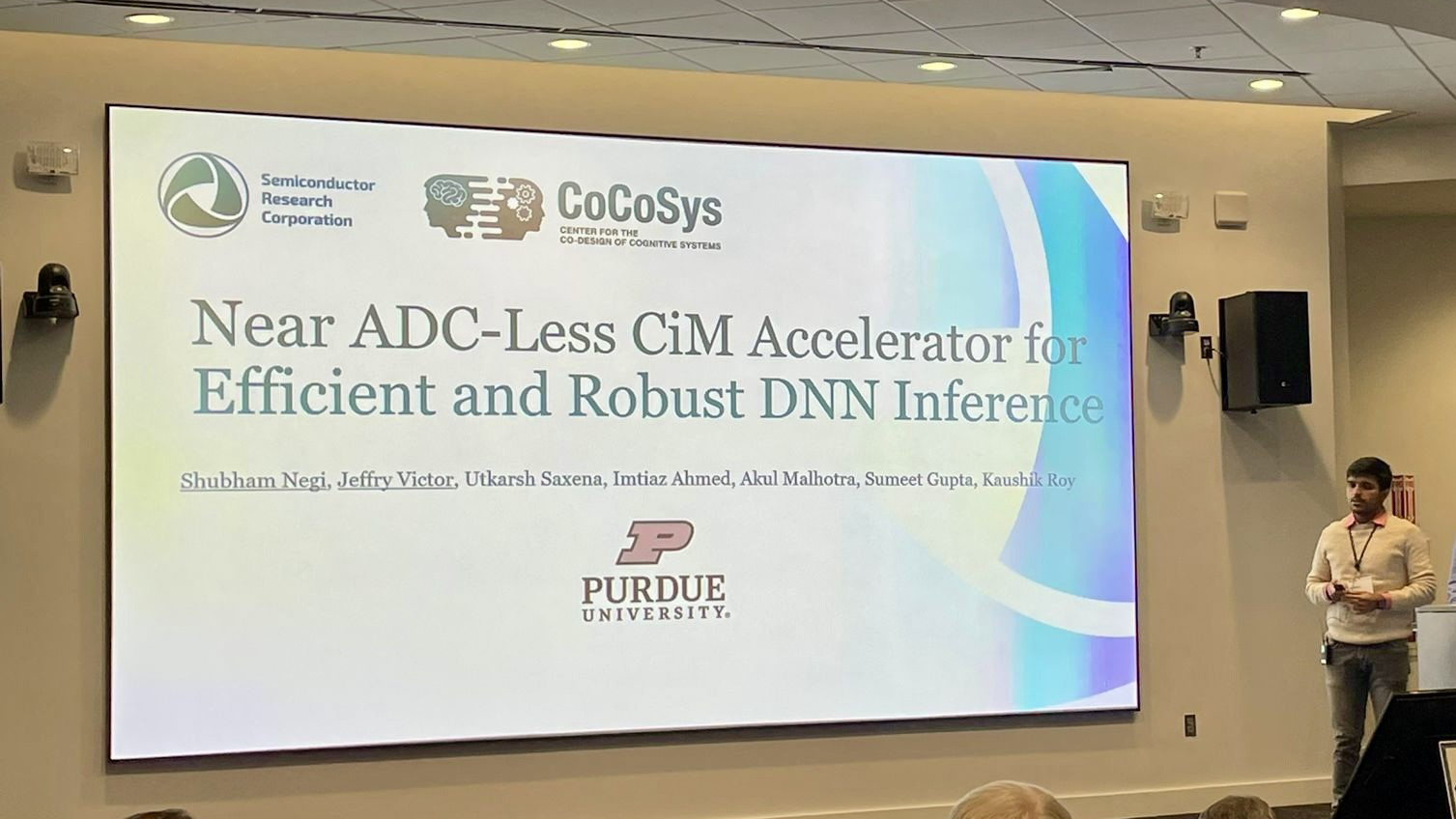
Amogh Joshi
Amogh Joshi, a graduate of the University of Mumbai, is currently a PhD student in Kaushik Roy's Nanoelectronics Research Laboratory (NRL), where he is tackling one of the most pressing challenges in reinforcement learning — enhancing sample efficiency and making reinforcement learning more explainable. His research is focused on incorporating human intuition into AI training, blending the power of physics with cutting-edge AI techniques.
Amogh explains, "Humans naturally grasp many fundamental physics concepts. By infusing this intuitive knowledge into AI agents, we can teach them to recognize optimal behaviors based on physical principles." His innovative approach, named SHIRE, aims to boost AI performance while making the learning process more transparent and interpretable. Instead of modeling complex real-world environments — an inherently difficult and computationally expensive task — Amogh is using task-specific human insights to guide the learning process. This method is not only more efficient but also easier to implement, offering great promise for both the AI and robotics communities.
In addition to his work on SHIRE, Amogh has worked on developing novel neuromorphic datasets and navigation approaches for aerial robotics. His groundbreaking research was recently showcased in two high-profile conferences. He and his colleagues presented their paper "FEDORA: A Flying Event Dataset fOr Reactive BehAvior" at the IEEE/RSJ International Conference on Intelligent Robots and Systems. Additionally, they shared their research on "Real-Time Neuromorphic Navigation: Integrating Event-Based Vision and Physics-Driven Planning on a Parrot Bebop2 Quadrotor" at the 40th Anniversary of the IEEE International Conference on Robotics and Automation.
Looking ahead, Amogh plans to apply his research on reinforcement learning, autonomous aerial robotics, and neuromorphic computing in a research team in industry, where he hopes to continue pushing the boundaries of what's possible in robotics.
Outside of his academic work, Amogh finds balance through his passion for baking, offering this piece of advice to fellow PhD students: "Make time for your hobbies during your PhD. They offer a much-needed break from the pressures of research and help keep you motivated."
Amogh has also enjoyed the camaraderie that comes with academic life, making lasting friendships and collaborations that have helped both his personal and professional growth. He's particularly excited about building and flying drones autonomously. "Showcasing the group's research contributions through drone flying demonstrations has been really exciting!" he shares (see video below).
Amogh's research is already making a significant impact, and with his dedication and vision, there's no doubt that he will continue to shape the future of AI and robotics.

Deepak Ravikumar
Deepak Ravikumar's academic journey has been marked by impressive achievements, from earning a bachelor's degree at Ramaiah Institute of Technology to receiving his master's degree at Purdue University. His academic passion then led him to pursue a PhD at Purdue University, where he currently works in Kaushik Roy's Nanoelectronics Research Laboratory (NRL).
Deepak focuses on Trustworthy AI, with a particular emphasis on memorization and privacy in deep learning models. His research also explores critical topics like out-of-distribution detection in vision models and adversarial robustness, addressing some of the most pressing challenges in AI security.
Currently, Deepak is investigating the question, "What do deep models memorize?" His groundbreaking research has demonstrated that utilizing second-order information from deep networks can significantly improve understanding of memorization and privacy. This work was recently featured as a spotlight publication entitled "Curvature Clues: Decoding Deep Learning Privacy with Input Loss Curvature" at the prestigious NeurIPS 2024 conference.
"Research in this field has always fascinated me," Deepak shared. "It offers insights into how AI models learn and how they differ from human cognition."
Deepak's work introduces efficient and formal methods to estimate memorization in deep models, offering the potential to reduce compute costs significantly. His approach is faster and simpler than existing methods and provides mathematical guarantees for estimating memorization and privacy. The impact is far-reaching: it enhances data privacy protection, ensures compliance with copyright and privacy laws, and enables testing of data leakage beneficial to individuals, tech companies and government agencies, aiding in the development of practical, scalable data privacy regulations.
Reflecting on his decision to pursue a PhD, Deepak said, "It was the right choice for me. I'm not sure where my career will take me, but I'm proud that my work at NRL is making a meaningful contribution to AI research. I truly enjoy my work, so it never feels like a job." He also offers advice for aspiring PhD students: "Research can be challenging, but don't give up. Stay curious, enjoy the process, and trust that the results will come."
Beyond his academic achievements, Deepak has formed lasting friendships with lab mates from diverse cultural backgrounds. He has also developed a love for running, climbing, and skiing, thanks to his colleagues. A standout memory from his PhD years was hiking Angel's Landing in winter. Deepak also enjoys playing guitar with the band Spider Monke, and one of his fondest memories is their performance at the Knickerbocker.
Deepak's work at NRL continues to inspire, and his contributions to the field of AI are poised to leave a lasting impact on both academia and industry.
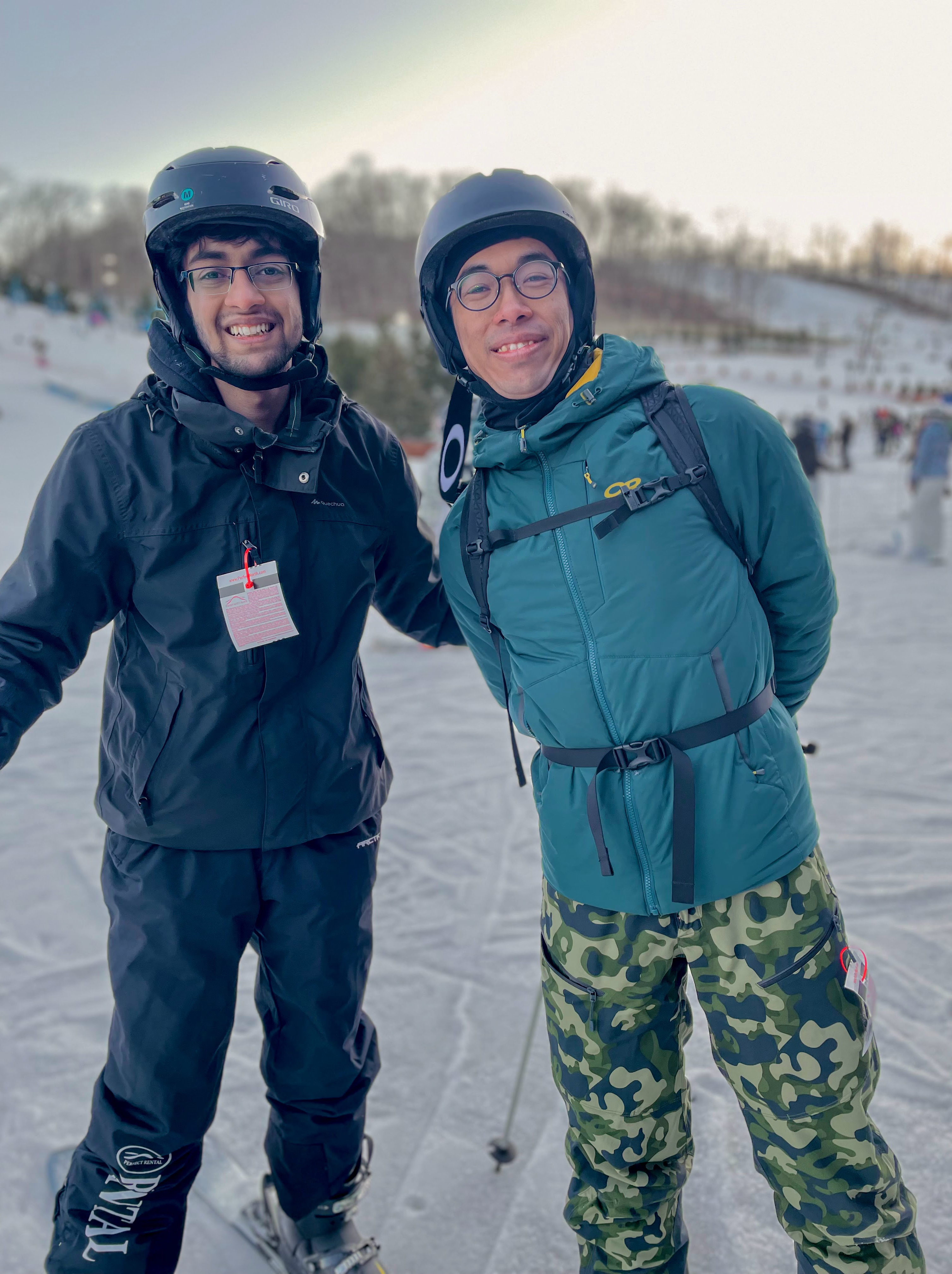
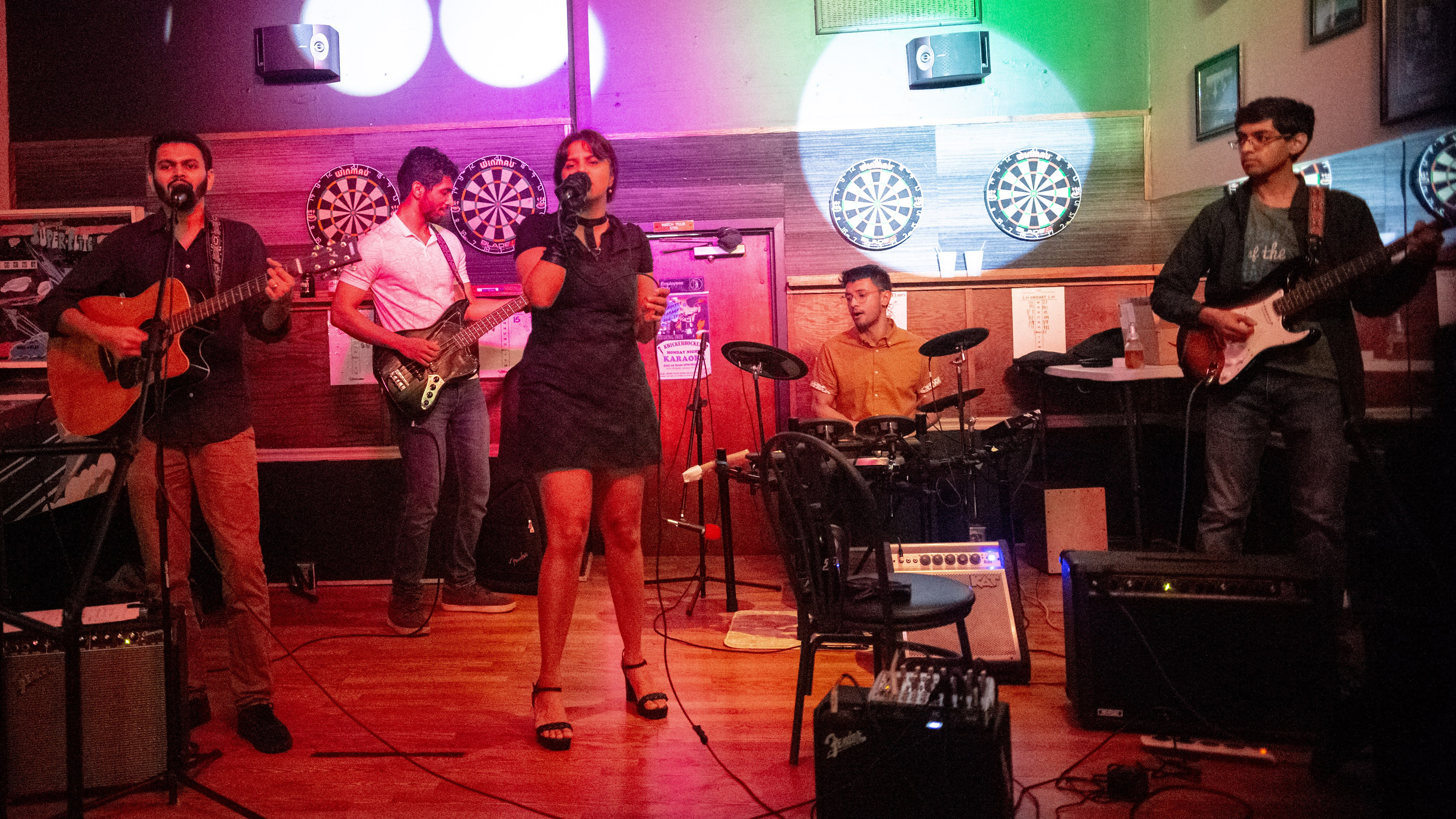
Sakshi Choudhary
Sakshi Choudhary, a graduate of Malaviya National Institute of Technology Jaipur, is currently a PhD student in Kaushik Roy's Nanoelectronics Research Laboratory (NRL) at Purdue University. Her groundbreaking research focuses on efficient distributed learning, specifically improving generalization in decentralized learning scenarios with heterogeneous data distributions.
Sakshi's work is centered around leveraging Sharpness-Aware Minimization (SAM), a cutting-edge optimizer that actively seeks flatter loss landscapes. SAM has been shown to be more robust than traditional optimization techniques. In the world of deep learning, this is a significant advancement, as SAM promises to enhance generalization while addressing the increasing demands of compute, energy, and data that state-of-the-art deep learning models require.
"One of the biggest challenges in distributed learning is handling heterogeneous data while ensuring communication efficiency," Sakshi explains. "Since user-generated data is highly diverse and often cannot be shared due to privacy concerns, there is a critical need for training algorithms that minimize communication overhead -- an area that can account for up to 70% of energy consumption."
"This research is unique because it focuses on decentralized learning with non-identical data distributions," she shares. "We aim to enhance generalization performance while maintaining communication efficiency." She believes that decentralized learning presents great promise, especially in the context of harnessing the distributed compute nodes and private user-generated data worldwide to train Large Language Models (LLMs).
As a PhD student, Sakshi enjoys the creative challenges of research and the opportunity to innovate. "Research can be full of uncertainty," she reflects, "but as Rollo May once said, 'Creative people are distinguished by the fact that they can live with anxiety, even though a high price might be paid for the gift of divine madness. They knock on silence for an answering music.' This quote resonates with me as I embrace the uncertainty in my work to create something meaningful." This feeling has shaped her career path toward a future where she can continue to push the boundaries of creativity and innovation.
Beyond her research, Sakshi is deeply involved in empowering the next generation of STEM leaders. She has contributed to the Stempower program at Purdue, which encourages young girls to pursue careers in science, technology, engineering, and mathematics. "Being part of Stempower was one of the most rewarding experiences," she says. "I will always cherish our trips to nearby schools, where we designed fun science experiments and interacted with Girl Scouts to ignite their passion for science!"
During Sakshi's time at Purdue, she has been rewarded with rich friendships and a sense of belonging. Her NRL friends and beyond made her graduate studies less daunting. With a passion for solving complex problems and a drive to make a difference, Sakshi Choudhary is truly a trailblazer in the world of AI and distributed learning.
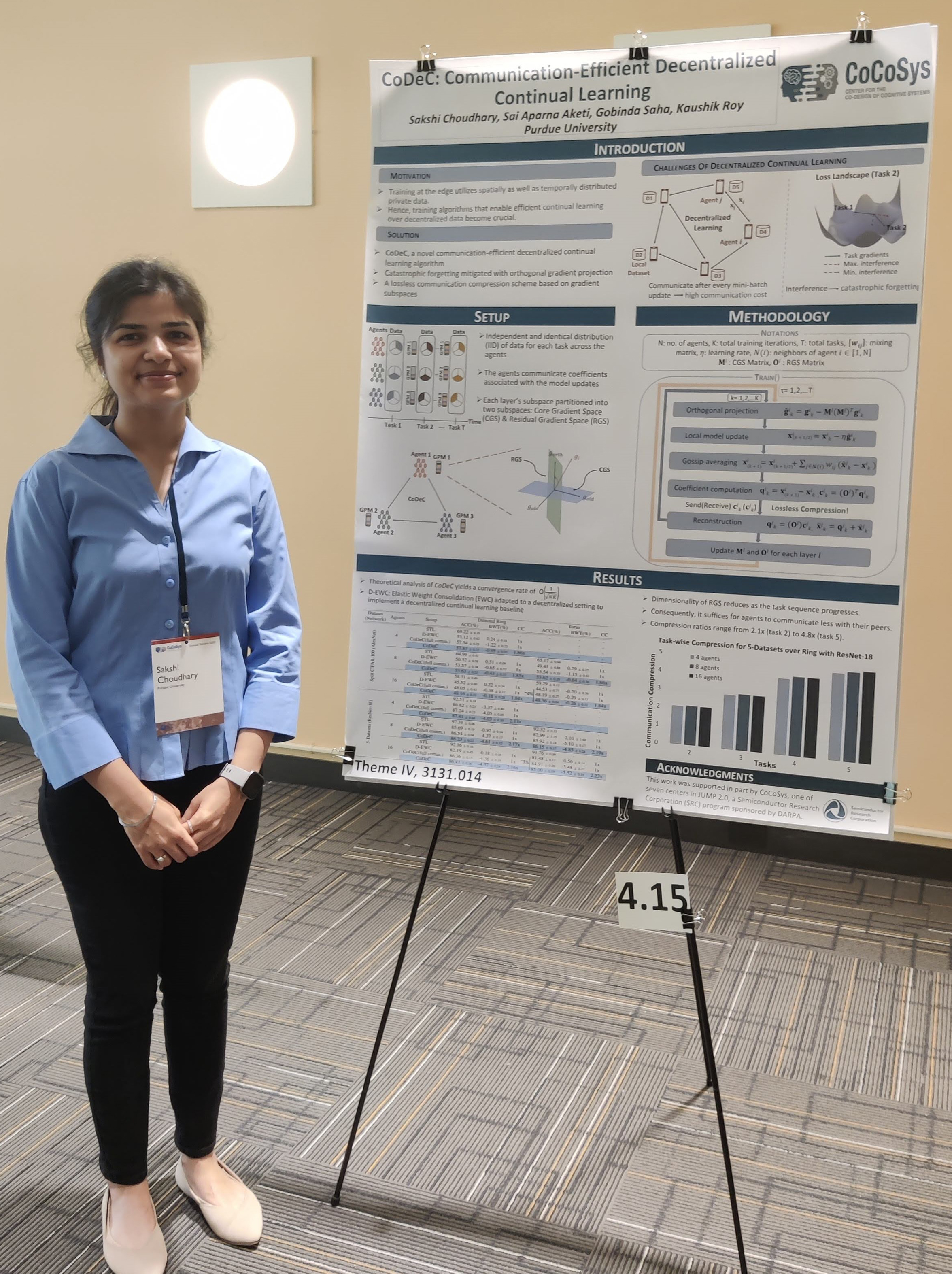
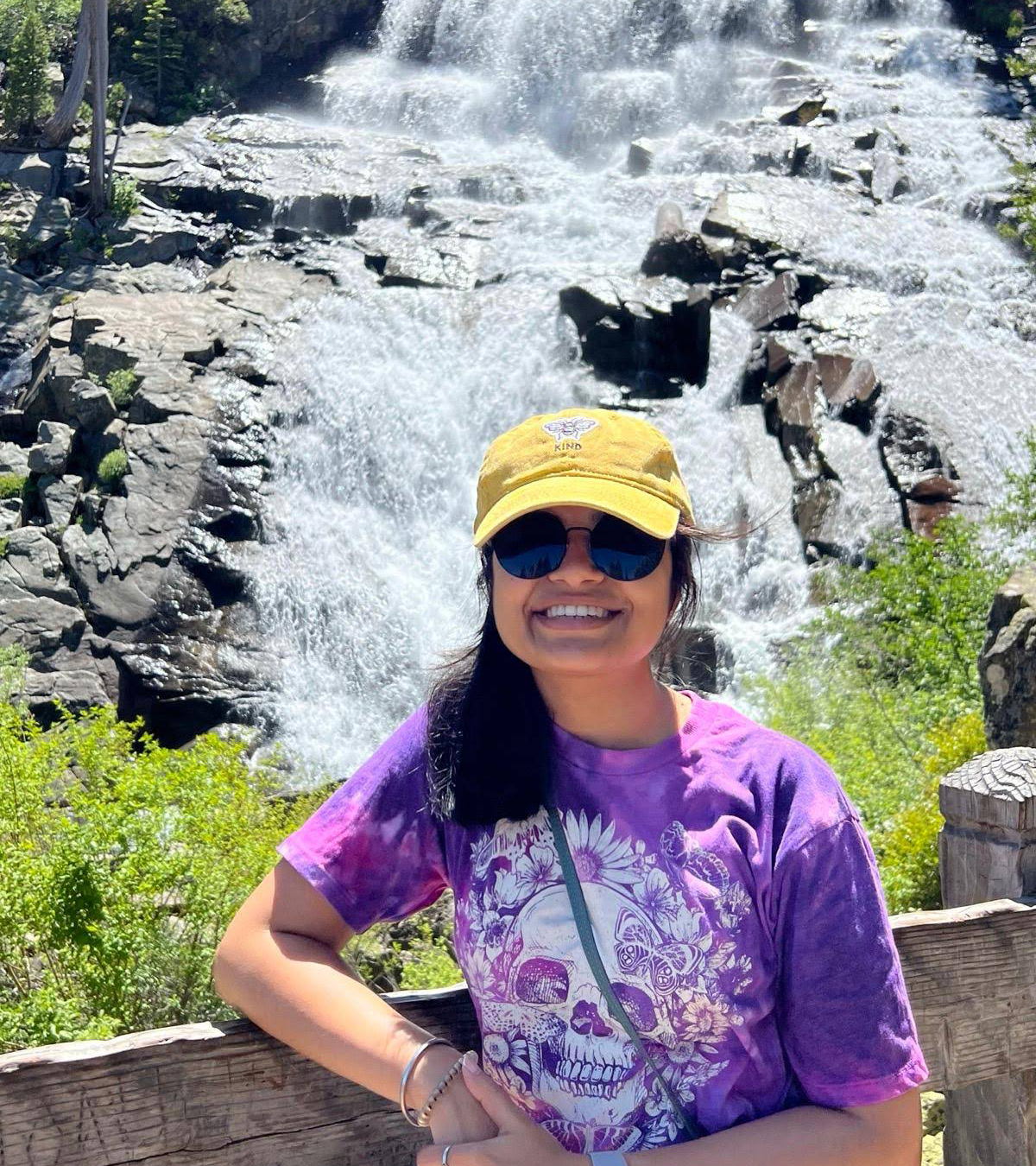
Soumyadeep Chandra
Soumyadeep Chandra joined Kaushik Roy's NRL in 2021 after earning his BS degree from Jadavpur University, Electronics and Communication Engineering. His research interest is in scene understanding and AI for hardware.
Soumyadeep is currently developing ThermAI, a generative AI-driven neural network architecture designed to predict the thermal behavior of electronic circuits under both transient and steady-state conditions. Unlike traditional thermal modeling, which is accurate but slow and computationally intensive, ThermAI offers faster, more efficient predictions. By optimizing circuit design and preventing overheating, ThermAI has the potential to enhance the performance and reliability of electronic systems across a range of applications. "ThermAI sits at the intersection of artificial intelligence, electronics, and thermal management -- three rapidly advancing fields with a substantial impact on modern technology," Soumyadeep explains.
In addition to his work on ThermAI, Soumyadeep is developing techniques for scene understanding and unsupervised temporal alignment of video data -- a critical challenge in computer vision. His research focuses on enabling machines to interpret complex, dynamic scenes without relying on costly labeled data. By leveraging unsupervised learning methods, Soumyadeep aims to allow systems to learn directly from raw video inputs, which could revolutionize applications like autonomous driving, surveillance, and healthcare, where real-time adaptability with minimal data is essential. "It's fascinating because it tackles one of the most complex challenges in computer vision -- helping machines understand the unpredictable, dynamic world as humans do," Soumyadeep explains.
Driven by a passion for solving real-world challenges at the crossroads of engineering, computer vision, and data science, Soumyadeep is focused on creating intelligent systems that can operate autonomously and provide valuable insights across various industries. "Research is tough, and it often takes multiple attempts to find solutions," he advises. "Approach each problem with curiosity and resilience, and always be open to learning and collaboration."
Although the transition to Purdue was initially challenging, Soumyadeep has found a supportive group of lab mates who make the PhD journey enjoyable. "We support each other both academically and personally," he shares. "I've learned to think critically, set meaningful life goals, and, on a lighter note, discovered that the secret to surviving a PhD involves fun road trips with friends and reconnecting with hobbies like badminton and painting."
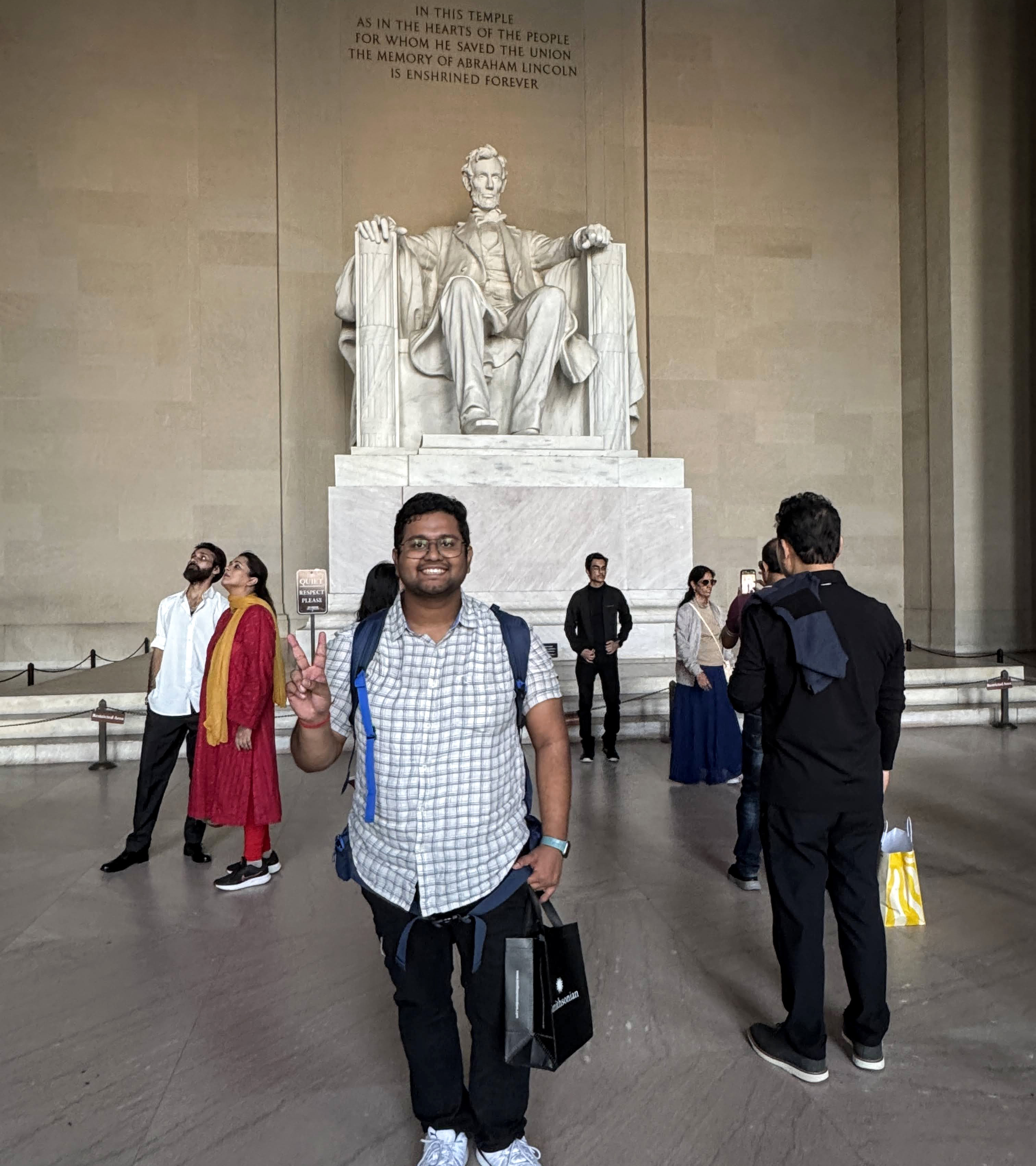
Manish Nagaraj
Purdue PhD candidate, Manish Nagaraj is currently working to reinforce and enhance a machine's ability to understand data. With a BS degree from PES Institute of Technology in Bangalore and an MS in Electrical Engineering from Purdue, Manish is focused on enhancing efficient usage of data in deep learning systems.
Currently, Manish is developing an innovative tool called the Relevancy metric, designed to evaluate the impact of individual data samples on deep learning model performance. It allows researchers to identify critical data subsets, streamlining training processes and boosting efficiency without compromising accuracy. By pinpointing high-impact samples, Manish's work enables models to learn from fewer data points, enhancing scalability and computational efficiency - key advantages in managing large datasets.
Manish passionately shares, "This research opens up new possibilities for improving data efficiency by revealing patterns in data that would otherwise go unnoticed, revealing redundancies and critical variations and biases. It opens up new avenues for improving data efficiency." His insights aim to transform model training by prioritizing the most valuable data, leading to more adaptable and resilient AI systems.
The implications of Manish's research extend to industries reliant on federated learning, such as healthcare and finance, where improved privacy and communication strategies can enhance collaborative AI efforts. Additionally, his real-time event-processing techniques can significantly benefit developers in robotics and transportation, enabling efficient object detection in fast-paced environments.
Manish's approach to tackling data efficiency encompasses several dimensions, from optimizing communication in distributed learning environments to creating scalable solutions for autonomous systems. "My goal is to drive innovative solutions to real-world problems using applied machine learning and deep learning," he explains.
As he prepares to lead a research team in industry after earning his PhD, Manish emphasizes the importance of collaboration and persistence. "This journey is not a sprint; it's a marathon. Pace yourself. Celebrate small milestones and seek feedback - it's crucial for staying motivated."
Outside of research, Manish is enjoying life at Purdue, discovering the joys of RPG gaming systems and diving into Dungeons and Dragons. "Our Saturday night board game sessions have become a highlight for me. It's a wonderful way to bond with friends and create lasting memories."
Manish's journey embodies the spirit of innovation and community, making significant strides in AI while fostering connections that enrich his graduate experience.
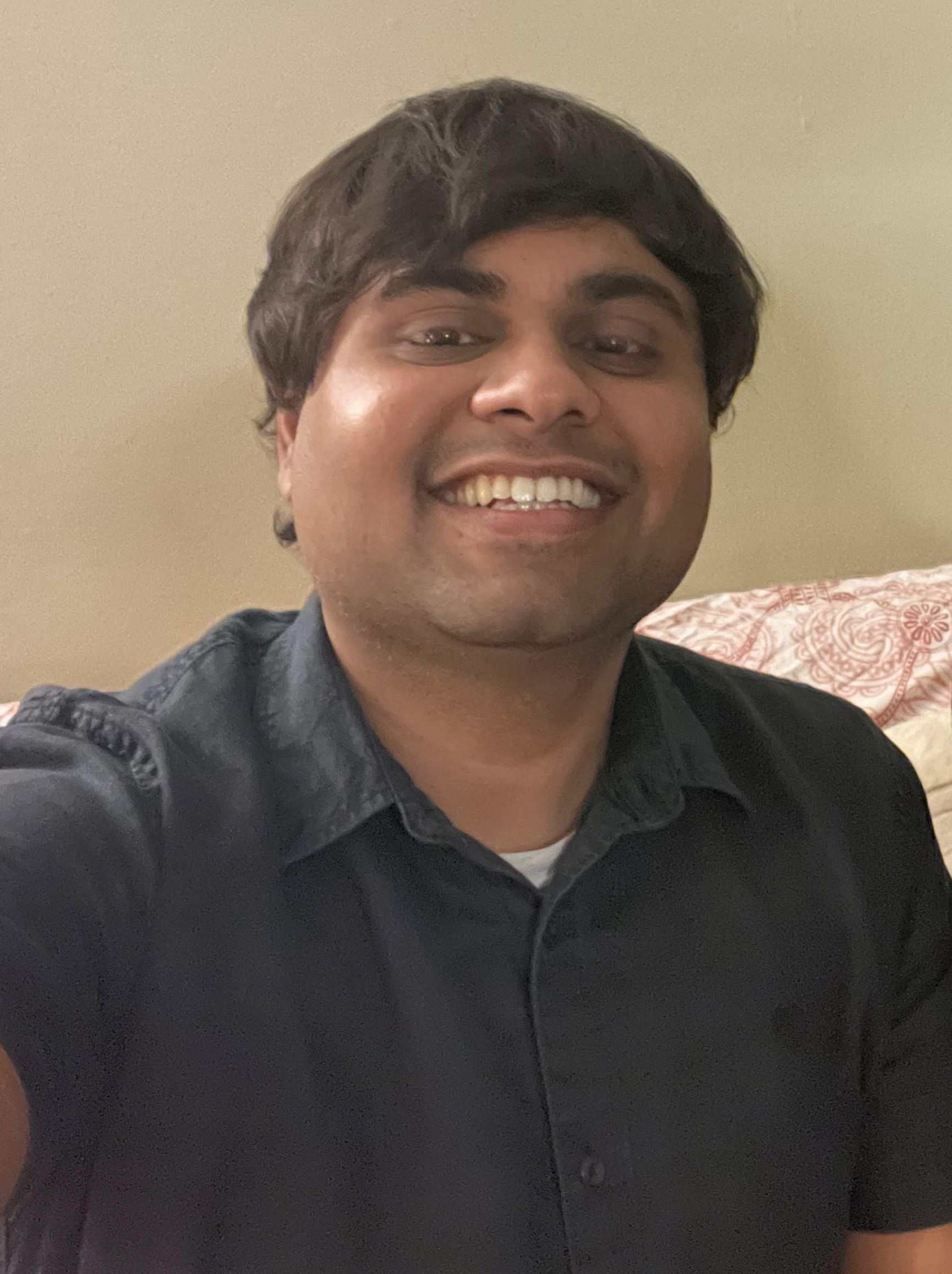
Sangamesh Kodge
A recent PhD graduate from Purdue ECE and Kaushik Roy's NRL, Sangamesh Kodge earned his BS from the Indian Institute of Technology, Kharagpur. His research explores cutting-edge topics in machine learning, machine unlearning, and natural language processing.
Sangamesh is leading a project titled SAP: Corrective Machine Unlearning with Scaled Activation Projection for Label Noise Robustness Label Corruption. This project tackles the issue of mislabeled training data, which can severely impact model performance. Collecting large, accurately labeled datasets is costly and time-consuming, and retraining models is resource-intensive.
To address this, Sangamesh developed Scaled Activation Projection (SAP), which uses Singular Value Decomposition (SVD) to correct label errors efficiently. SAP efficiently estimates correct labels using SVD, making it practical with limited mislabeled samples. This work is setting a new standard for corrective unlearning techniques and is expected to inspire further advancements in algorithms for large foundation models. Sangamesh is passionate about this research because label noise undermines AI reliability, and he aims to create effective solutions for machine learning.
Sangamesh's goal is to continue his research in machine learning, driven by the exciting advancements in artificial intelligence. He aims to contribute to groundbreaking research and collaborate with talented individuals to push the boundaries of what is possible in this field.
Throughout his PhD journey, Sangamesh has learned several key lessons that he hopes will benefit others. He says, "Focus on the problem you're solving and iterate and experiment for insights." He also says, "Don't try to do this alone -- communicate effectively, collaborate for new perspectives, and build strong relationships for support throughout your PhD journey."
Sangamesh was actively involved in the ECE Graduate Student Association and the ECE Graduate Committee, which helped him connect with peers and faculty, fostering a supportive community through various social and professional activities.
He fondly recalls his time in the NRL. Not only did they work on exciting projects together, but they also formed lasting friendships. Some of his favorite memories include adventurous trips with friends to places like the Great Smoky Mountains, Yellowstone, Alaska, and the Grand Canyon. He says, "These experiences will always remind me of my graduate studies at Purdue."
Sangamesh's journey reflects the passion and dedication of students striving to make significant contributions to the field of machine learning.
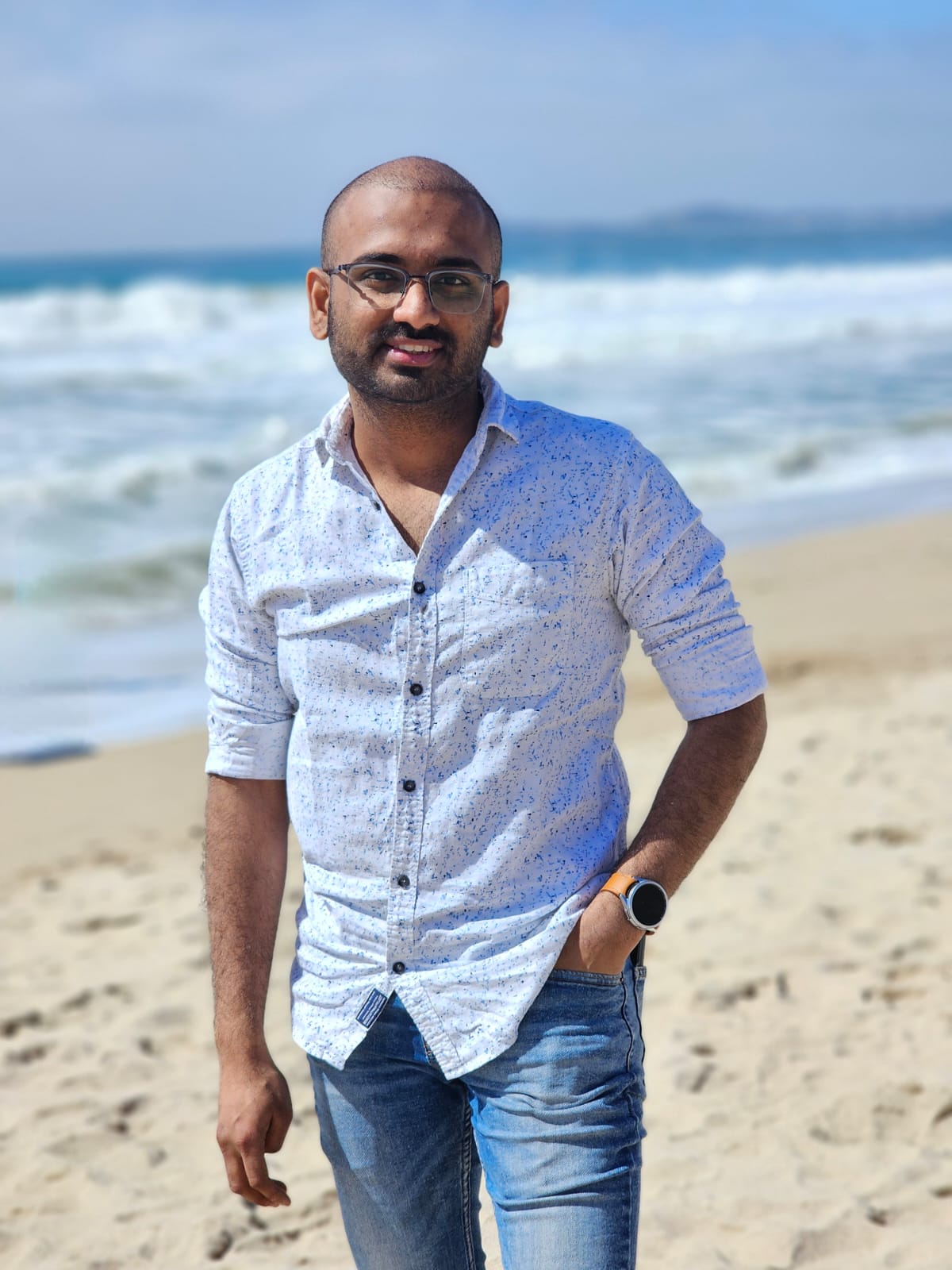
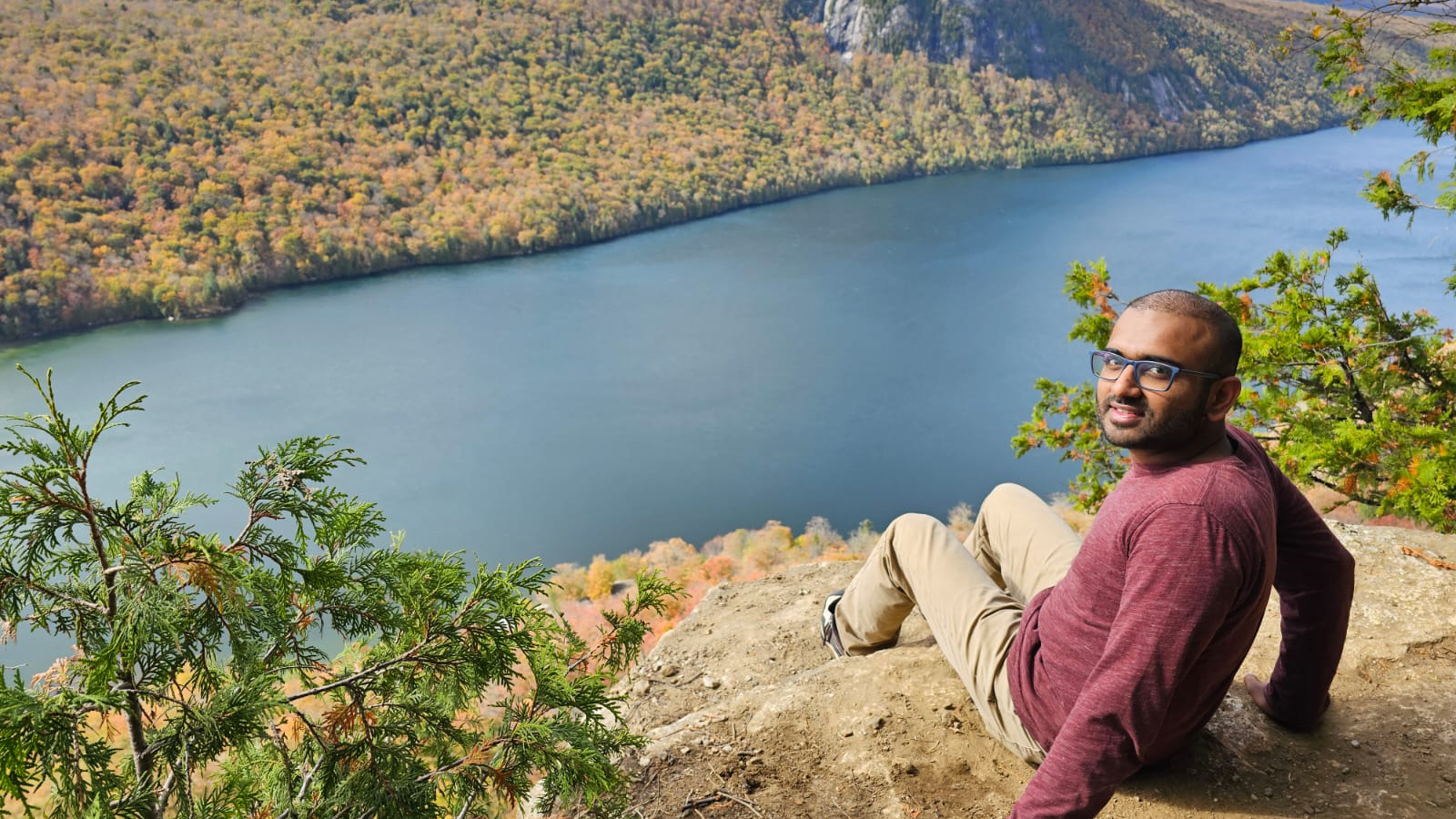
Sourav Sanyal
Meet Sourav Sanyal, a graduate student working under the direction of Professor Kaushik Roy. His passion for robotics and artificial intelligence is shaping the future of autonomous systems. Hailing from Jadavpur University in India, Sourav's academic journey has led him to pursue research in multimodal AI-guided autonomous navigation.
His current work aims to harness the power of Vision Language Models within Robot Operating System (ROS) frameworks to achieve holistic scene comprehension for robotic agents. "This work perfectly blends all the cool and relevant sub-fields I dabble with," he shares. His enthusiasm for combining different areas of AI reflects a desire to innovate and push the boundaries of what's possible in robotics.
At the heart of Sourav's research lies a critical challenge: how can robots understand and navigate complex environments like humans do? By dissecting state-of-the-art methods and integrating them into practical robotic tasks, he aims to create next-generation cognitive agents capable of interacting with the world in more sophisticated ways.
Sourav's commitment to advancing the field was showcased when he presented his paper titled "EV-Planner: Energy-Efficient Robot Navigation via Event-Based Physics-Guided Neuromorphic Planner," co-authored with Rohan Kumar Manna and Kaushik Roy, at the 40th Anniversary of the IEEE International Conference on Robotics and Automation. This opportunity not only highlighted his innovative work but also positioned him among leading researchers in the field, contributing valuable insights into energy-efficient robotic navigation.
Sourav's work is poised to benefit anyone interested in the latest advancements in AI for robotics. His unique approach -- breaking down complex methodologies to understand their core principles -- distinguishes his research in a crowded field. As he puts it, "I strive to make the latest AI trends accessible for tomorrow's robots."
With his sights set on securing an Applied Research Scientist role in a research lab, Sourav offers sage advice for future members of the NRL lab: "Have faith in the process. Work hard but don't forget to have fun along the way."
When asked about his most memorable experiences at Purdue, Sourav smiles, acknowledging the myriad of moments -- both sweet and bitter -- that have shaped his graduate life. "Too many to count. Each experience is close to my heart," he says, hinting at the friendships, challenges, and lessons learned that will stay with him long after graduation.

Efstathia Soufleri
Congratulations to Efstathia Soufleri on earning her PhD in Electrical and Computer Engineering from the Purdue Elmore Family School of Electrical and Computer Engineering! Efstathia was honored with her hood by her advisor, Professor Kaushik Roy, during the Purdue graduation ceremony on Saturday, August 3, 2024.
Efstathia completed her undergraduate studies at the National and Kapodistrian University of Athens. Her doctoral research focused on developing privacy-preserving and efficient machine learning algorithms. With her PhD now in hand, Efstathia is set to embark on a tenure-track faculty position in Europe.
In her most recent project, Efstathia devised an innovative method to train and perform inference directly on compressed videos. By leveraging the curvature of networks processing these compressed video modalities as a proxy, she achieved significant computational advantages. Her approach, which includes early exits in neural networks, offers improved generalization results, especially in scenarios with low inference latency and limited computational resources.
During her time with the NRL, Efstathia valued the opportunity to foster new collaborations and engage in discussions with fellow NRL members. The weekly NRL presentations and meetings were instrumental in keeping her informed about others' work, which helped refine her own research.
Efstathia cherished the chance to meet and connect with many new people through NRL, noting that she "formed friendships that will endure beyond my graduation." Her advice to future PhD students is to "Enjoy the PhD journey and make the most of this experience."
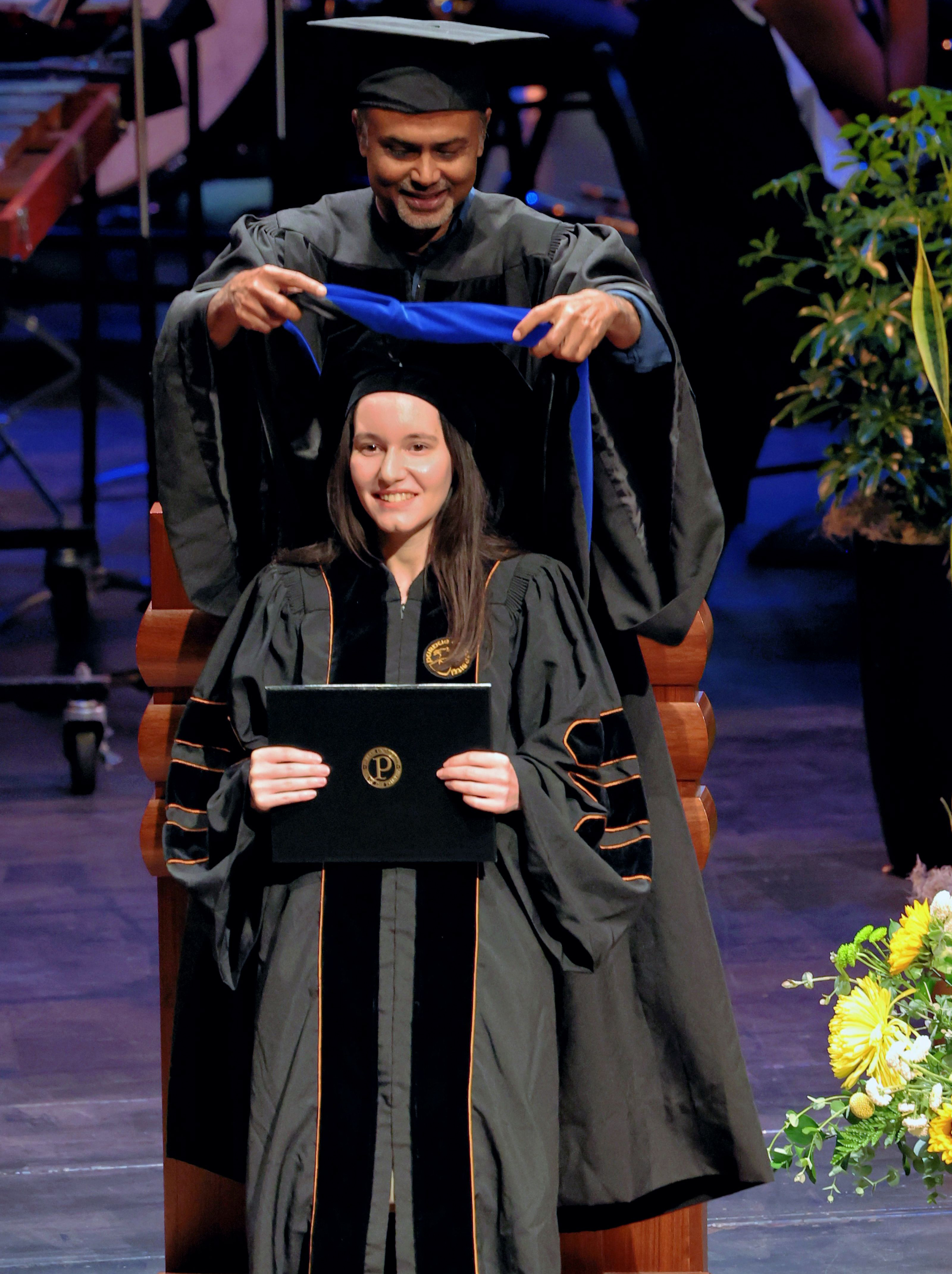