Naveen Verma
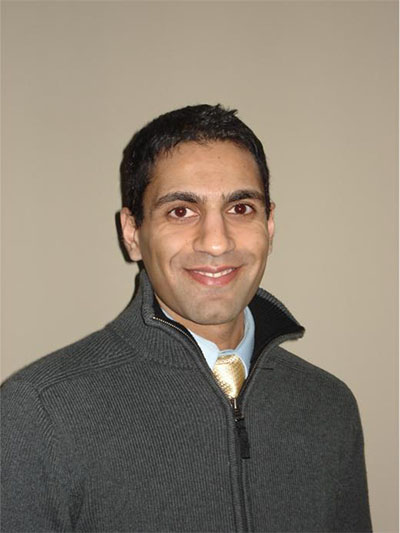
Naveen Verma received the B.A.Sc. degree in Electrical and Computer Engineering from the UBC, Vancouver, Canada in 2003, and the M.S. and Ph.D. degrees in Electrical Engineering from MIT in 2005 and 2009 respectively. Since July 2009 he has been with the Department of Electrical Engineering at Princeton University, where he is currently an Associate Professor. His research focuses on advanced sensing systems, exploring how systems for learning, inference, and action planning can be enhanced by algorithms that exploit new sensing and computing technologies. This includes research on large-area, flexible sensors, energy-efficient statistical-computing architectures and circuits, and machine-learning and statistical-signal-processing algorithms. Prof. Verma has served as a Distinguished Lecturer of the IEEE Solid-State Circuits Society, and currently serves on the technical program committees for ISSCC, VLSI Symp., DATE, and IEEE Signal-Processing Society (DISPS). Prof. Verma is recipient or co-recipient of the 2006 DAC/ISSCC Student Design Contest Award, 2008 ISSCC Jack Kilby Paper Award, 2012 Alfred Rheinstein Junior Faculty Award, 2013 NSF CAREER Award, 2013 Intel Early Career Award, 2013 Walter C. Johnson Prize for Teaching Excellence, 2013 VLSI Symp. Best Student Paper Award, 2014 AFOSR Young Investigator Award, 2015 Princeton Engineering Council Excellence in Teaching Award, and 2015 IEEE Trans. CPMT Best Paper Award.
- M. Ozatay, L. Aygun, H. Jia, P. Kumar, Y. Mehlman, C. Wu, S. Wagner, J. C. Sturm, and N. Verma, "Artificial Intelligence Meets Large-scale Sensing: using Large-Area-Electronics (LAE) to enable intelligent spaces," IEEE Custom Integrated Circuits Conf. (CICC), May 2018.
- Y. Afsar, T. Moy, N. Brady, S. Wagner, J. C. Sturm, and N. Verma, "An Architecture for Large-Area Sensor Acquisition Using Frequency-Hopping ZnO TFT DCOs," IEEE J. Solid-State Circuits, (JSSC), vol. 53, no. 1, pp 297-308, Jan. 2018.
- Z. Wang and N. Verma, "A Low-Energy Machine-Learning Classifier Based on Clocked Comparators for Direct Inference on Analog Sensors," IEEE Trans. on Circuits and Systems (TCAS-I), vol. no. 11, pp. 2954-2965, Nov. 2017.
- H. Jia, J. Lu, N. K. Jha, and N. Verma. "A Heterogeneous Microprocessor for Energy-scalable Sensor Inference Using Genetic Programming," IEEE VLSI Symp. on Circuits (VLSIC), June 2017.
- J. Zhang, Z. Wang, and N. Verma, "In-Memory Computation of a Machine-Learning Classifier in a Standard 6T SRAM Array," IEEE J. of Solid-State Circuit (JSSC), vol. 52, no. 4, pp. 915-924, April 2017.
- T. Moy, L. Huang, W. Rieutort-Louis, C. Wu, P. Cuff, S. Wagner, J. C. Sturm, and N. Verma, "An EEG Acquisition and Biomarker-Extraction System Using Low-Noise-Amplifier and Compressive-Sensing Circuits Based on Flexible, Thin-Film Electronics," IEEE J. of Solid-State Circuits (JSSC), vol. 52, no. 1, pp. 309-321, Jan. 2017.
- T. Moy, W. Rieutort-Louis, S. Wagner, J. C. Sturm, and N. Verma, "A Thin-film, Large-area Sensing and Compression System for Image Detection," IEEE Trans. on Circuits and Systems I (TCAS-I), Vol. 63, no. 11, pp. 1833-1844, Nov. 2016.
- W. Rieutort-Louis, T. Moy, Z. Wang, S. Wagner, J. C. Sturm, and N. Verma, "A Large-Area Image Sensing and Detection System Based on Embedded Thin-Film Classifiers," IEEE J. Solid-State Circuits (JSSC), vol. 51, no. 1, pp. 281-290, Jan. 2016.
- Z. Wang, R. Schapire, and N. Verma, "Error Adaptive Classifier Boosting (EACB): Leveraging Data-Driven Training Towards Hardware Resilience for Signal Inference," IEEE Trans. Circuits and Systems I (TCAS-I), vol. 62, no. 4, pp. 1136-1145, April 2015.
- Z. Wang, K. H. Lee, and N. Verma, "Overcoming Computational Errors in Sensing Platforms through Embedded Machine-learning Kernels," IEEE Trans. VLSI Systems (TVLSI).